Machine-Learning-Based Predictive Modeling Analysis in Ambient RF Energy Harvesting for IoT Systems
IEEE Sensors Journal(2024)
摘要
The Internet of Things (IoT) has already ingrained itself into our daily lives, with the number of connected devices that are growing rapidly. Particularly, low-power wireless sensing devices are anticipated to make significant contributions to this expansion. These compact devices are designed to operate for an extended duration, spanning years or even decades, but the growing demand for such devices poses challenges in terms of ensuring sustainable power supply. To sustainably power these devices, ambient radio-frequency (RF) energy harvesting has emerged as a possible approach. However, placing the harvester in an optimal location is essential to maximize the reception of ambient RF energy and ensure reliable performance. In this article, we investigate the estimation of the ideal location for RF energy harvesting by utilizing machine-learning (ML) techniques in real-world scenarios. The study involves a frequency-dependent analysis and a received signal intensity analysis. A comparison of three different interpolation methods with five supervised ML algorithms is conducted, and the effect of reduced measurement points on estimation accuracy is evaluated. The outcomes demonstrate how well ML estimates the optimal location for energy scavenging and offer insights into creating sustainable energy systems.
更多查看译文
关键词
Energy harvesting,Internet of Things (IoT),machine learning (ML),prediction modeling,supervised learning
AI 理解论文
溯源树
样例
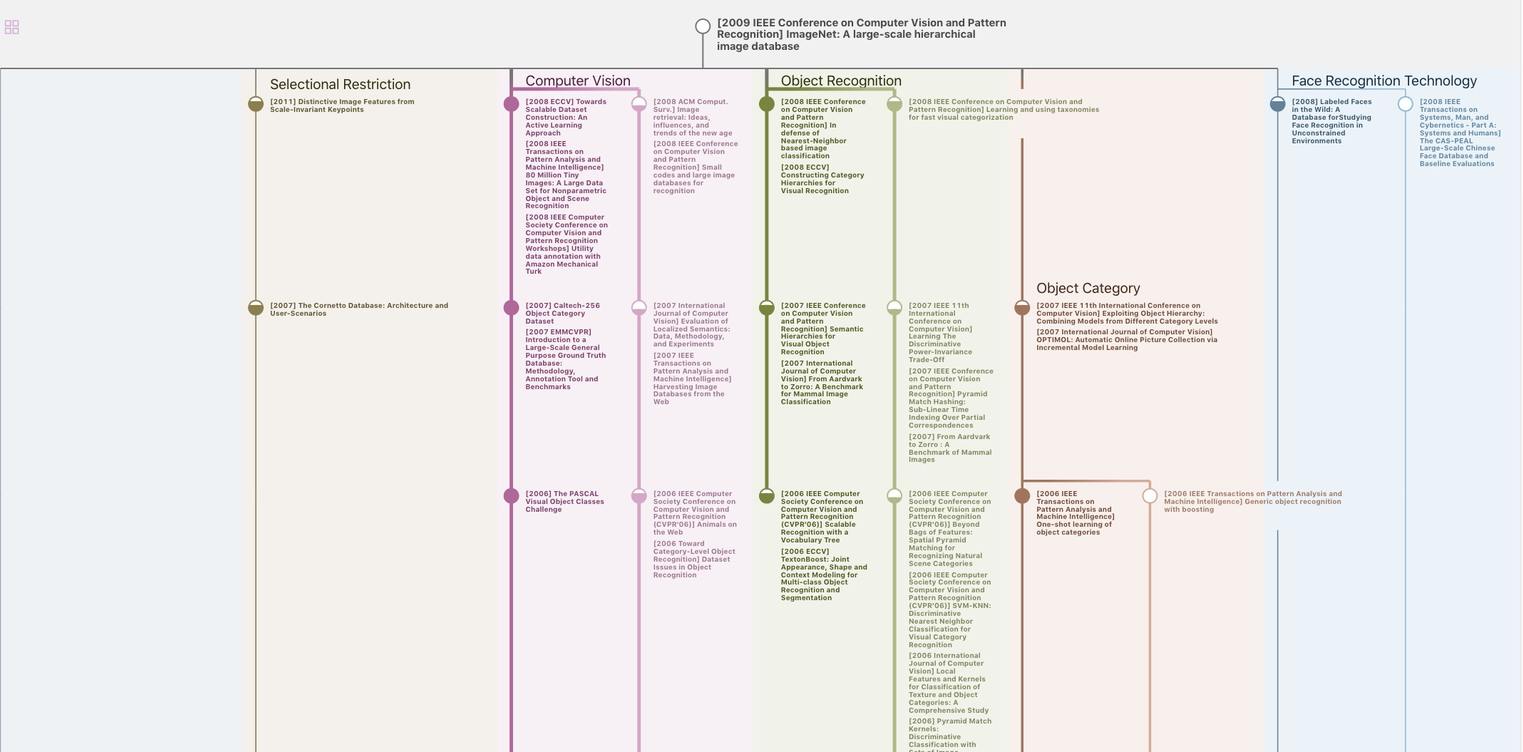
生成溯源树,研究论文发展脉络
Chat Paper
正在生成论文摘要