The effects of gender bias in word embeddings on patient phenotyping in the mental health domain
2023 11TH INTERNATIONAL CONFERENCE ON AFFECTIVE COMPUTING AND INTELLIGENT INTERACTION, ACII(2023)
摘要
Word embeddings, renowned for their role as superior semantic feature vector representation in diverse NLP tasks, can exhibit an undesired bias for stereotypical categories. The bias arises from the statistical and societal biases within the datasets used for training. In this study, we analyze the gender bias in four different pre-trained word embeddings for a range of affective computing tasks in the mental health domain including the detection of psychiatric disorders such as depression, and alcohol/substance abuse. We incorporate both contextual and non-contextual embeddings, which are trained not just on general domain data but also on data specific to the clinical domain. Our findings indicate that the bias in embeddings is towards different gender groups, depending on the type of embeddings and the training dataset. Furthermore, we highlight how these existing associations transfer to subsequent tasks and might even be amplified during supervised training for patient phenotyping. We also show that a simple method of data augmentation - swapping gender words - noticeably reduces bias in these subsequent tasks. The scripts to reproduce the results are available at: https://github.com/gizemsogancioglu/gender-bias-mental-health.
更多查看译文
关键词
fairness,bias mitigation,gender bias,bias in mental health,fairness in machine learning
AI 理解论文
溯源树
样例
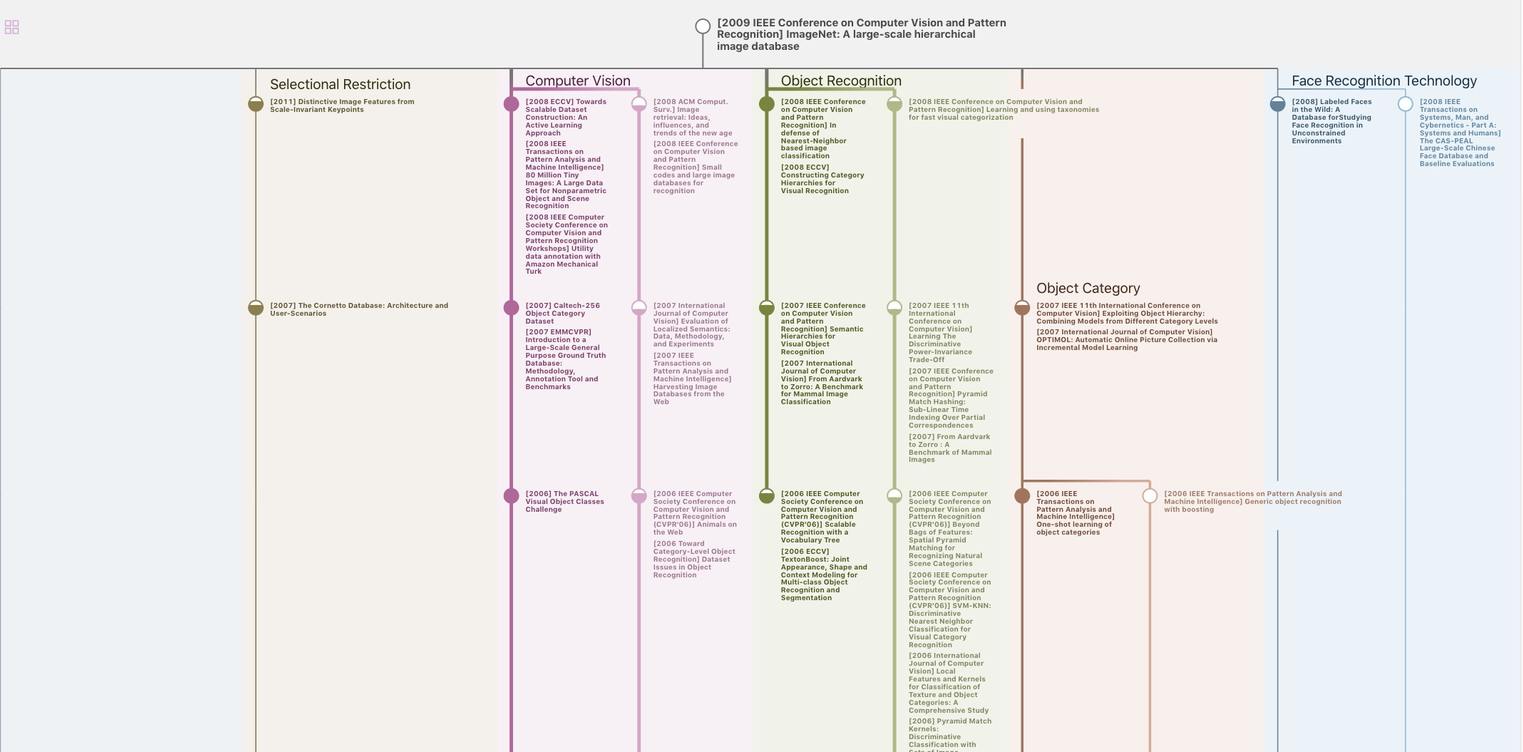
生成溯源树,研究论文发展脉络
Chat Paper
正在生成论文摘要