3D Knee and Hip Angle Estimation With Reduced Wearable IMUs via Transfer Learning During Yoga, Golf, Swimming, Badminton, and Dance
IEEE TRANSACTIONS ON NEURAL SYSTEMS AND REHABILITATION ENGINEERING(2024)
摘要
Wearable lower-limb joint angle estimation using a reduced inertial measurement unit (IMU) sensor set could enable quick, economical sports injury risk assessment and motion capture; however the vast majority of existing research requires a full IMU set attached to every related body segment and is implemented in only a single movement, typically walking. We thus implemented 3-dimensional knee and hip angle estimation with a reduced IMU sensor set during yoga, golf, swimming (simulated lower body swimming in a seated posture), badminton, and dance movements. Additionally, current deep-learning models undergo an accuracy drop when tested with new and unseen activities, which necessitates collecting large amounts of data for the new activity. However, collecting large datasets for every new activity is time-consuming and expensive. Thus, a transfer learning (TL) approach with long short-term memory neural networks was proposed to enhance the model's generalization ability towards new activities while minimizing the need for a large new-activity dataset. This approach could transfer the generic knowledge acquired from training the model in the source-activity domain to the target-activity domain. The maximum improvement in estimation accuracy (RMSE) achieved by TL is 23.6 degrees for knee flexion/extension and 22.2 degrees for hip flexion/extension compared to without TL. These results extend the application of motion capture with reduced sensor configurations to a broader range of activities relevant to injury prevention and sports training. Moreover, they enhance the capacity of data-driven models in scenarios where acquiring a substantial amount of training data is challenging.
更多查看译文
关键词
Deep learning,prosthesis,activity recog-nition,reduced sensor set,transformer
AI 理解论文
溯源树
样例
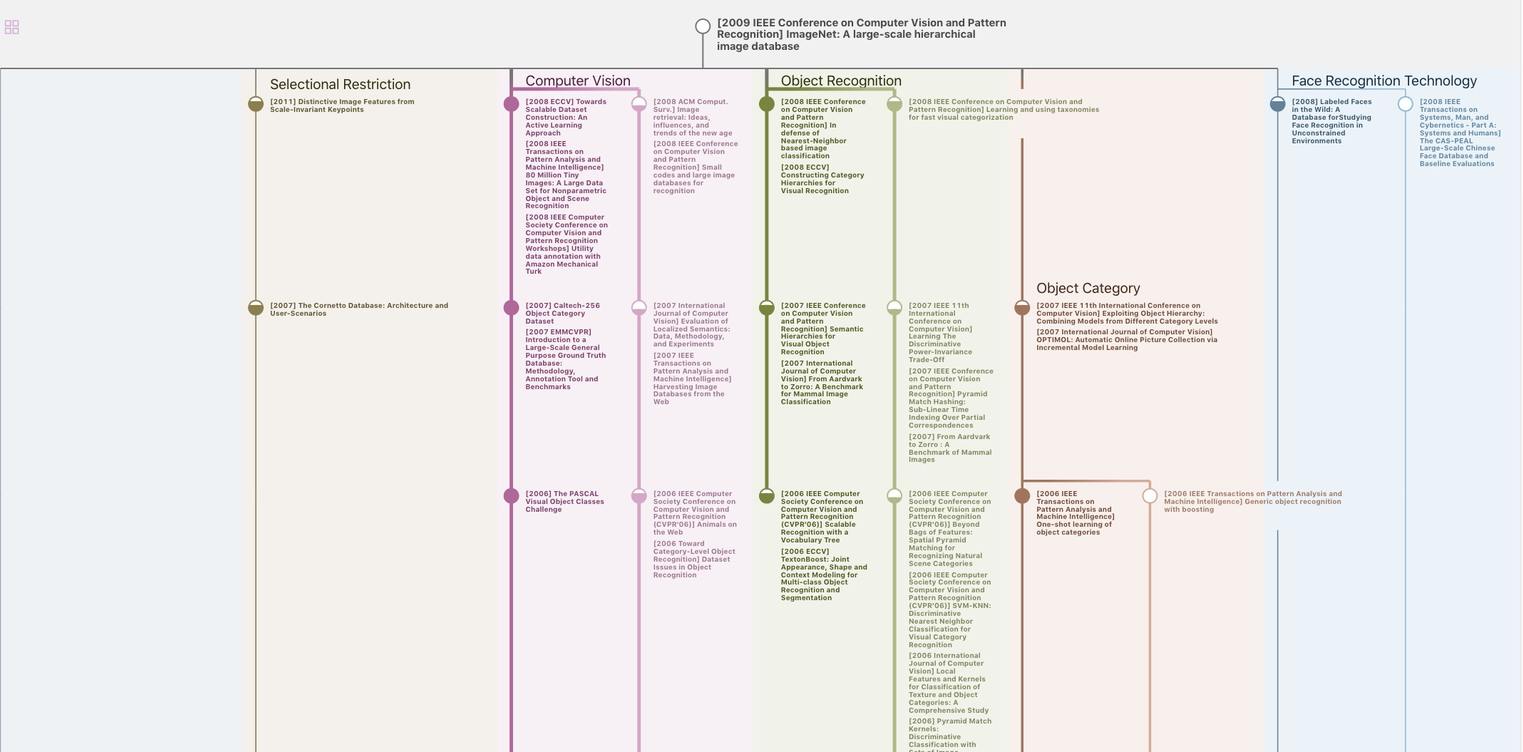
生成溯源树,研究论文发展脉络
Chat Paper
正在生成论文摘要