Pseudo anomalies enhanced deep support vector data description for electrocardiogram quality assessment
COMPUTERS IN BIOLOGY AND MEDICINE(2024)
摘要
Electrocardiogram (ECG) recordings obtained from wearable devices are susceptible to noise interference that degrades the signal quality. Traditional methods for assessing the quality of electrocardiogram signals (SQA) are mostly supervised and typically rely on limited types of noise in the training data, which imposes limitations in detecting unknown anomalies. The high variability of both ECG signals and noise presents a greater challenge to the generalization of traditional methods. In this paper, we propose a simple and effective unsupervised SQA method by modeling the SQA of ECG as a problem of anomaly detection, in which, a model of pseudo anomalies enhanced deep support vector data description is introduced to learn a more discriminative and generalized hypersphere of the high-quality ECG in a self-supervised manner. Specifically, we propose a series of ECG noise-generation methods to simulate the noise of real scenarios and use the generated noise samples as the pseudo anomalies to correct the hypersphere learned solely by the high-quality ECG samples. Finally, the quality of ECG can be measured based on the distance to the center of the hypersphere. Extensive experimental results on multiple public datasets and our constructed real -world 12-lead dataset demonstrate the effectiveness of the proposed method.
更多查看译文
关键词
Electrocardiogram,Quality assessment,Anomaly detection,Pseudo anomalies,Deep learning
AI 理解论文
溯源树
样例
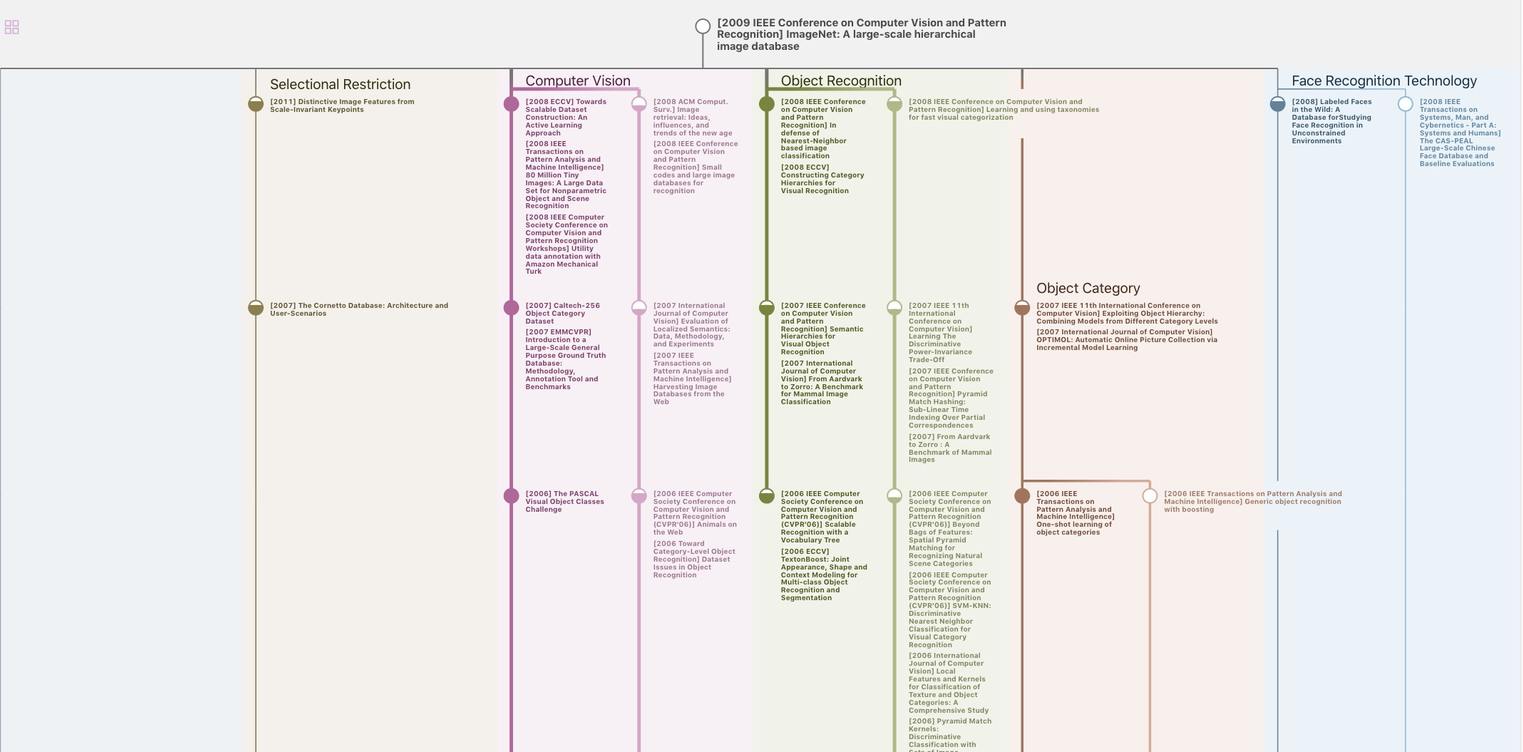
生成溯源树,研究论文发展脉络
Chat Paper
正在生成论文摘要