Multi-scale architectures matter: Examining the adversarial robustness of flow-based lossless compression
PATTERN RECOGNITION(2024)
摘要
Exact likelihood estimation on entropy coding makes flow-based models appealing for lossless image compression. However, the high fidelity storage cost is affected by the lossless compression ratio. The trade -off between efficiency and robustness of flow-based deep lossless compression models has not been fully explored. This paper characterizes the trade -off theoretically and empirically, revealing that flow-based models are susceptible to adversarial examples resulting in a significant change in compression ratio. The fragile robustness of flowbased models is due to their intrinsic multi-scale architectures lacking the Lipschitz property. Based on this insight, a stronger white-box attack, Auto-Weighted Projected Gradient Descent (AW-PGD), is developed to generate more universal adversarial examples. Additionally, a novel flow-based lossless compression model, Robust Integer Discrete Flow (R-IDF), is proposed to achieve comparable robustness to adversarial training without sacrificing compression efficiency. Experiments demonstrate that the PGD algorithm falls into local extreme values when attacking the compression model, but the proposed attack and defense methods effectively improve the invulnerability of the flow-based compression model.
更多查看译文
关键词
Flow models,Lossless compression,Adversarial attack,Lipschitz property
AI 理解论文
溯源树
样例
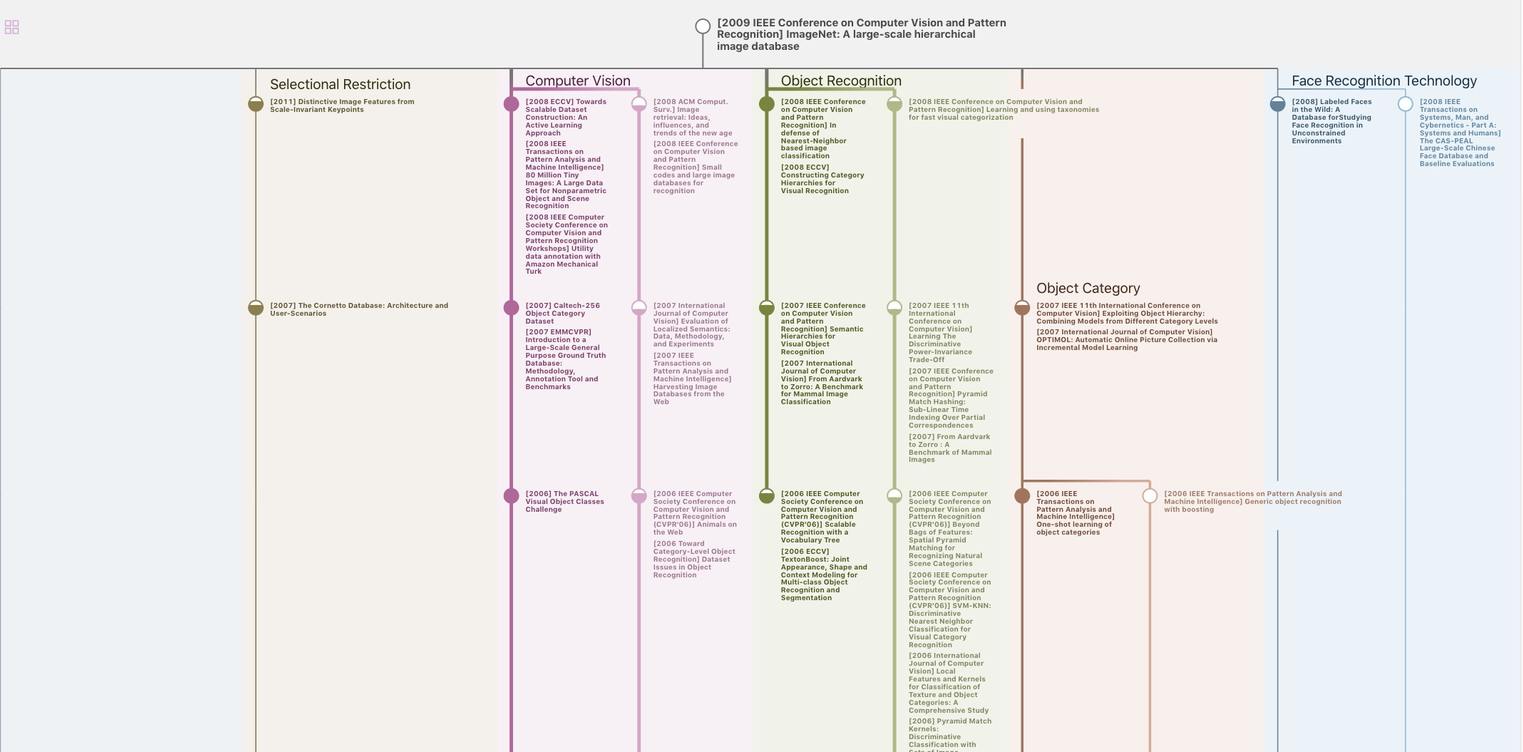
生成溯源树,研究论文发展脉络
Chat Paper
正在生成论文摘要