Fatigue life prediction of the FCC-based multi-principal element alloys via domain knowledge-based machine learning
ENGINEERING FRACTURE MECHANICS(2024)
摘要
We propose a machine learning (ML) model to predict the fatigue life of multi -principal element alloys (MPEAs) by extracting features from empirical formulas. The model is built on XGBoost and GBDT, and outperforms the single ML model, with almost all predictions lying in the +/- 2 error bands and the relative error not exceeding 0.16 in the extrapolation test. Feature analysis shows that for the nine explored MPEAs systems, their S-N curves are more suitable to be fitted by log N = a + b log sigma(max). Interpretable analysis indicates that for the explored alloys, elongation > 47% benefits the increase of fatigue life; if their yield strength is less than 720 MPa, improving strength will favor improvement in lifetime, otherwise improving ductility will favor lengthening their lifetime. It provides a fast and low-cost method to predict the fatigue life of those FCC -based MPEAs, which guides designing alloys with longer fatigue life.
更多查看译文
关键词
Fatigue life prediction,Domain knowledge,Machine learning,Multi-principal element alloy,Small dataset
AI 理解论文
溯源树
样例
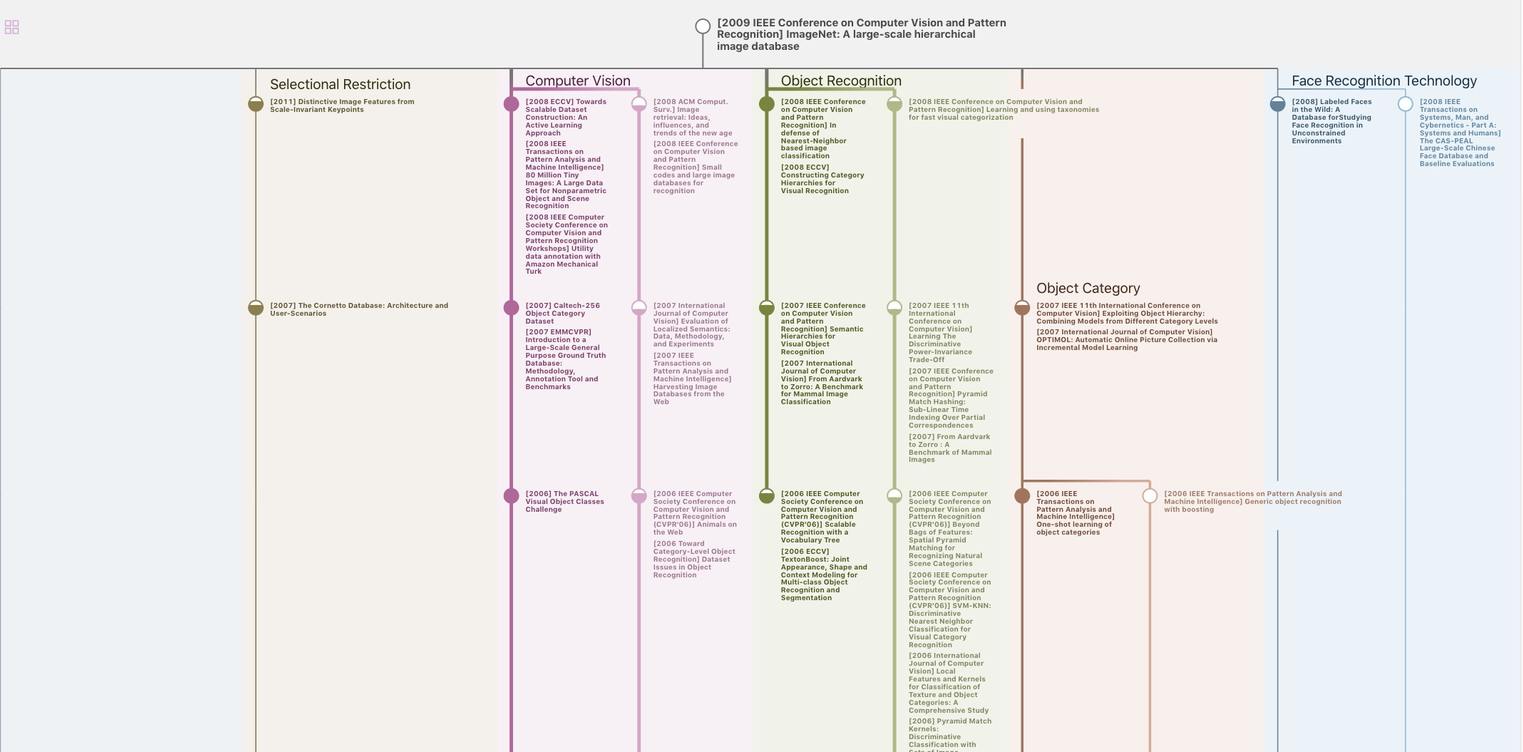
生成溯源树,研究论文发展脉络
Chat Paper
正在生成论文摘要