SHAP values accurately explain the difference in modeling accuracy of convolution neural network between soil full-spectrum and feature-spectrum
COMPUTERS AND ELECTRONICS IN AGRICULTURE(2024)
摘要
Acquiring soil nutrient content quickly and accurately through remote sensing is the key to advance precision agriculture. The development of deep learning has provided new technical means for soil hyperspectral modeling. However, the problem of poor interpretability of deep learning models limits its development. Although SHapley Additive exPlanations (SHAP) values based on game theory have been successfully applied to the interpretation of deep learning soil spectral modeling, whether they can accurately explain the differences in deep learning model accuracy remains to be verified. Based on this, we explored whether SHAP values can accurately explain the differences in convolutional neural network (CNN) modeling accuracy. We collected soil samples from agricultural land in the Liangshui River Basin in the southern mountainous and hilly areas of China, and measured the soil total nitrogen (STN) content and soil spectral data in the laboratory. We compared the effects of full-spectrum and feature-spectrum on the accuracy of deep learning models, and obtained the contribution of wavelengths in the CNN modeling process by calculating SHAP values. The results showed that combining different spectral pre-processing methods can play their respective advantages and help improve modeling accuracy. Among them, the CNN model obtained the highest prediction accuracy under the firstderivative Savitzky-Golay smoothing combination standard normal variate (SG1-SNV) spectral pre-processing in full-spectrum modeling. Compared with the feature-spectrum selected for modeling by Mutual information (MI) and competitive adaptive reweighted sampling (CARS), the CNN model achieved higher accuracy in most pre-processed spectra in full-spectrum modeling, and SHAP values accurately explained this reason. This is because the contribution is usually higher at most wavelengths with a high correlation with STN content. The feature-spectrum selected by CARS is more widely distributed but lacks continuity, and some wavelengths with high correlation and high contribution will also be missed. Meanwhile, some wavelengths with low correlation also have high contributions, which are usually not involved in the feature spectrum modeling of MI, thus affecting the modeling accuracy. Therefore, the deep learning model is more suitable for full-spectrum modeling due to its strong feature extraction and self-learning capabilities, and SHAP can obtain the wavelength contribution of the CNN model in soil spectral modeling, and then explain the differences in modeling accuracy. This study further proves the interpretability of deep learning, provides an important basis for the application of deep learning in soil hyperspectral modeling.
更多查看译文
关键词
Deep learning,Convolutional neural network,Interpretability,SHAP values,Soil total nitrogen
AI 理解论文
溯源树
样例
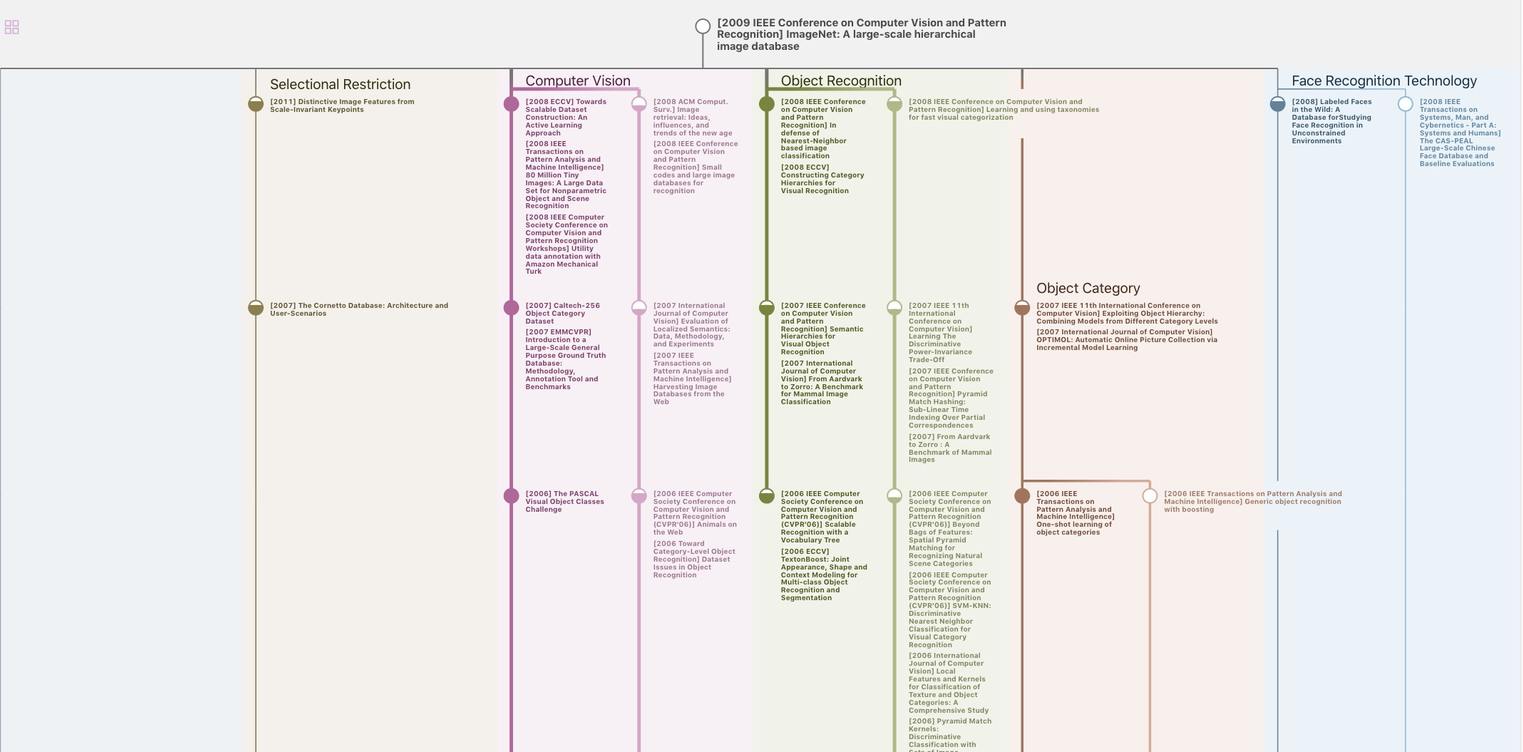
生成溯源树,研究论文发展脉络
Chat Paper
正在生成论文摘要