Cross-Dataset Distillation with Multi-tokens for Image Quality Assessment
PATTERN RECOGNITION AND COMPUTER VISION, PRCV 2023, PT VI(2024)
摘要
No Reference Image Quality Assessment (NR-IQA) aims to accurately evaluate image distortion by simulating human assessment. However, this task is challenging due to the diversity of distortion types and the scarcity of labeled data. To address these issues, we propose a novel attention distillation-based method for NR-IQA. Our approach effectively integrates knowledge from different datasets to enhance the representation of image quality and improve the accuracy of predictions. Specifically, we introduce a distillation token in the Transformer encoder, enabling the student model to learn from the teacher across different datasets. By leveraging knowledge from diverse sources, our model captures essential features related to image distortion and enhances the generalization ability of the model. Furthermore, to refine perceptual information from various perspectives, we introduce multiple class tokens that simulate multiple reviewers. This not only improves the interpretability of the model but also reduces prediction uncertainty. Additionally, we introduce a mechanism called Attention Scoring, which combines the attention-scoring matrix from the encoder with the MLP header behind the decoder to refine the final quality score. Through extensive evaluations of six standard NR-IQA datasets, our method achieves performance comparable to the state-of-the-art NR-IQA approaches. Notably, it achieves SRCC values of 0.932 (compared to 0.892 in TID2013) and 0.964 (compared to 0.946 in CSIQ).
更多查看译文
关键词
Image quality assessment,Distillation,Transformer
AI 理解论文
溯源树
样例
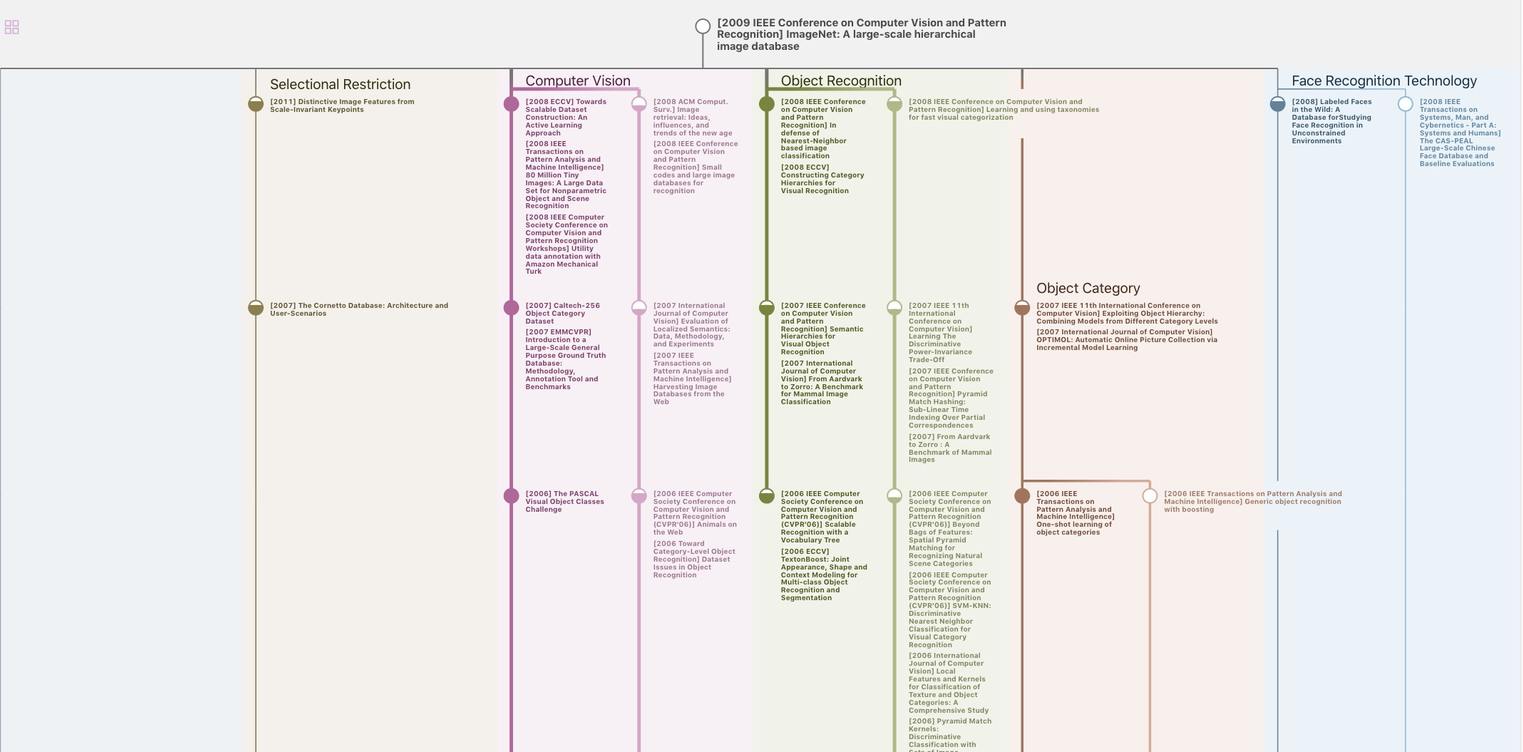
生成溯源树,研究论文发展脉络
Chat Paper
正在生成论文摘要