TPNet: Enhancing Weakly Supervised Polyp Frame Detection with Temporal Encoder and Prototype-Based Memory Bank
PATTERN RECOGNITION AND COMPUTER VISION, PRCV 2023, PT XII(2024)
Abstract
Polyp detection plays a crucial role in the early prevention of colorectal cancer. The availability of large-scale polyp video datasets and video-level annotations has spurred research efforts to formulate polyp detection as a weakly-supervised anomaly detection task, which leverages video-level labeled training data for detecting frame-level polyps. However, few studies have investigated the impact of specific properties within polyp videos, including temporal dynamics, ambiguity, and complex noise. In this work, we propose TPNet, a novel framework that addresses several challenges posed by colonoscopy videos, for weakly-supervised polyp frame detection. Specifically, we design a Temporal Encoder that effectively capturing the temporal dynamics and intricate patterns within polyp video segments to foster accuracy. Additionally, we introduce a Prototype-based Memory Bank that facilitates the storage and retrieval of significant discriminative information, which enhance the sensitivity and robustness in ambiguous and complicated conditions. Experiments conducted on one of the largest and most challenging colonoscopy datasets demonstrate that our proposed TPNet achieves state-of-the-art performance, surpassing the latest cutting-edge method with 6.19% in average precision (AP).
MoreTranslated text
Key words
Polyp Detection,Weakly Surpervised,Temporal Encoder,Prototype-based Memory Bank
AI Read Science
Must-Reading Tree
Example
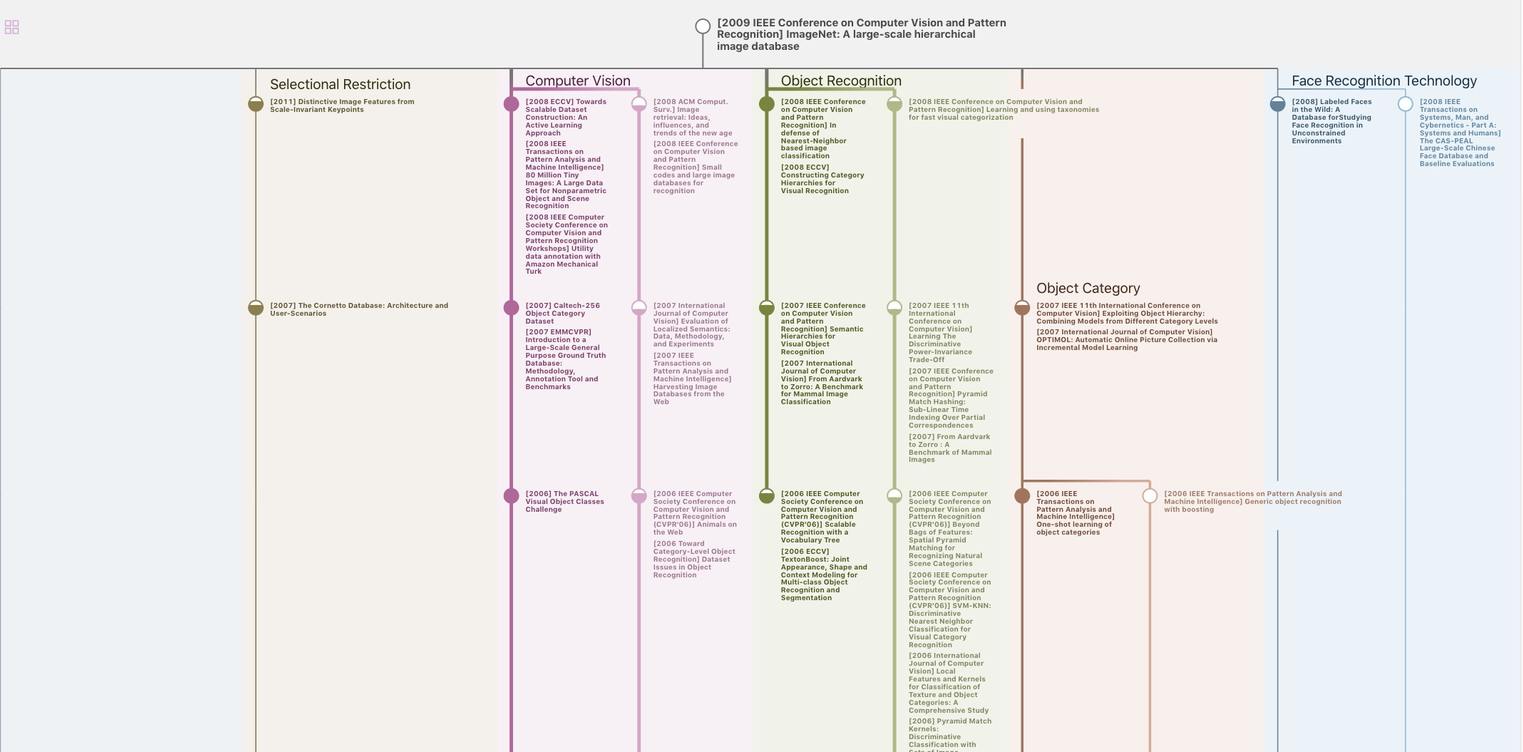
Generate MRT to find the research sequence of this paper
Chat Paper
Summary is being generated by the instructions you defined