ContextNet: Learning Context Information for Texture-Less Light Field Depth Estimation
PATTERN RECOGNITION AND COMPUTER VISION, PRCV 2023, PT VI(2024)
摘要
Depth estimation in texture-less regions of the light field is an important research direction. However, there are few existing methods dedicated to this issue. We find that context information is significantly crucial for depth estimation in texture-less regions. In this paper, we propose a simple yet effective method called ContextNet for texture-less light field depth estimation by learning context information. Specifically, we aim to enlarge the receptive field of feature extraction by using dilated convolutions and increasing the training patch size. Moreover, we design the Augment SPP (AugSPP) module to aggregate features of multiple-scale and multiple-level. Extensive experiments demonstrate the effectiveness of our method, significantly improving depth estimation results in texture-less regions. The performance of our method outperforms the current state-of-the-art methods (e.g., LFattNet, DistgDisp, OACC-Net, and SubFocal) on the UrbanLF-Syn dataset in terms of MSE x100, BadPix 0.07, BadPix 0.03, and BadPix 0.01. Our method also ranks third place of comprehensive results in the competition about LFNAT Light Field Depth Estimation Challenge at CVPR 2023 Workshop without any post-processing steps (The code and model are available at https:// github.com/chaowentao/ContextNet.).
更多查看译文
关键词
Light field,Depth estimation,Texture-less regions
AI 理解论文
溯源树
样例
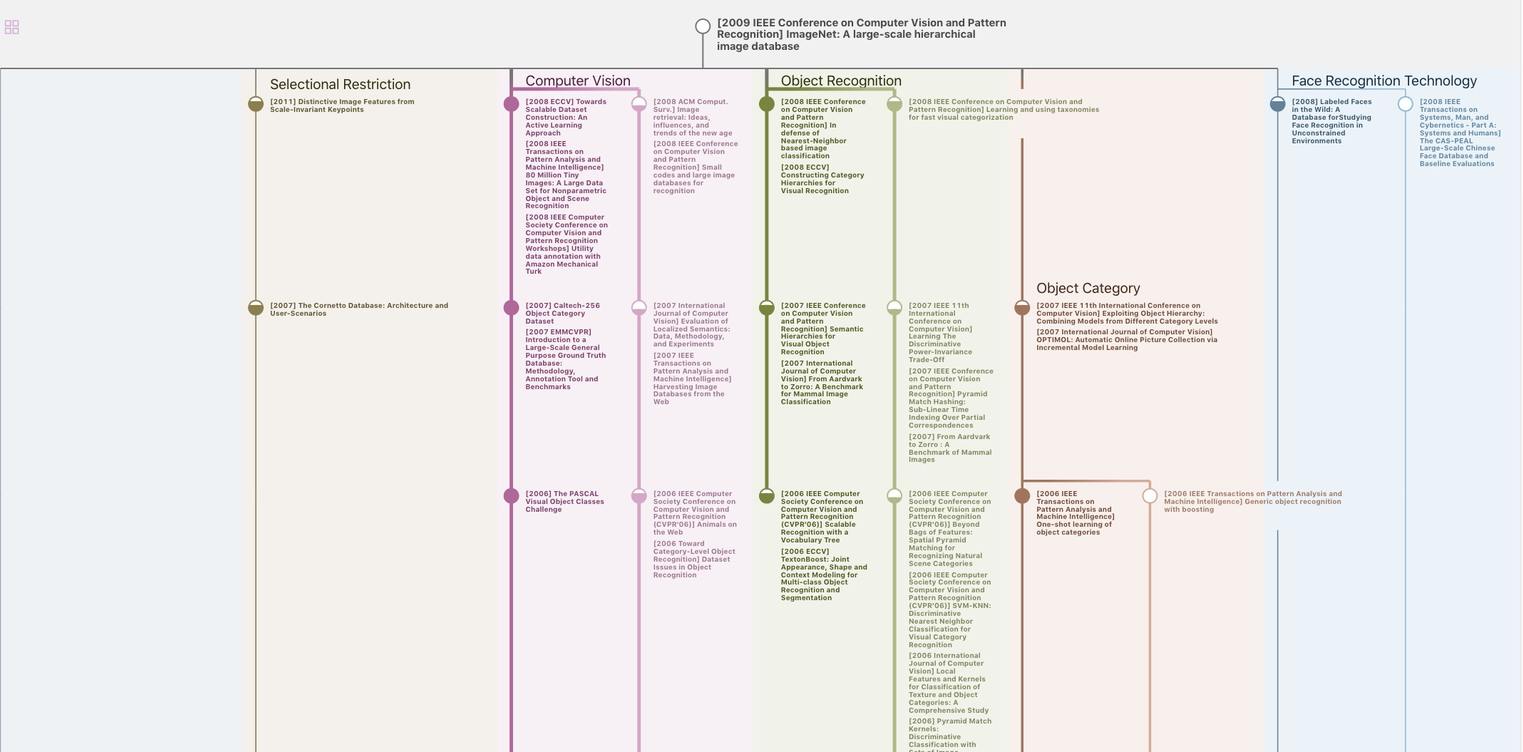
生成溯源树,研究论文发展脉络
Chat Paper
正在生成论文摘要