GLViG: Global and Local Vision GNN May Be What You Need for Vision
PATTERN RECOGNITION AND COMPUTER VISION, PRCV 2023, PT IX(2024)
摘要
In this article, we propose a novel vision architecture termed GLViG, which leverages graph neural networks (GNNs) to capture local and important global information in images. To achieve this, GLViG represents image patches as graph nodes and constructs two types of graphs to encode the information, which are subsequently processed by GNNs to enable efficient information exchange between image patches, resulting in superior performance. In order to address the quadratic computational complexity challenges posed by high-resolution images, GLViG adaptively samples the image patches and optimizes computational complexity to linear. Finally, to enhance the adaptation of GNNs to the 2Dimage structure, we use Depth-wise Convolution dynamically generated positional encoding as a solution to the fixed-size and static limitations of absolute position encoding in ViG. The extensive experiments on image classification, object detection, and image segmentation demonstrate the superiority of the proposed GLViG architecture. Specifically, the GLViG-B1 architecture achieves a significant improvement on ImageNet-1K when compared to the state-of-the-art GNN-based backbone ViG-Tiny (80.7% vs. 78.2%). Additionally, our proposed GLViG model surpasses popular computer vision models such as Convolutional Neural Networks (CNNs), Vision Transformers (ViTs), and Vision MLPs. We believe that our method has great potential to advance the capabilities of computer vision and bring a new perspective to the design of new vision architectures.
更多查看译文
关键词
Graph Neural Networks,Image Classification,Object Detection,Image Segmentation
AI 理解论文
溯源树
样例
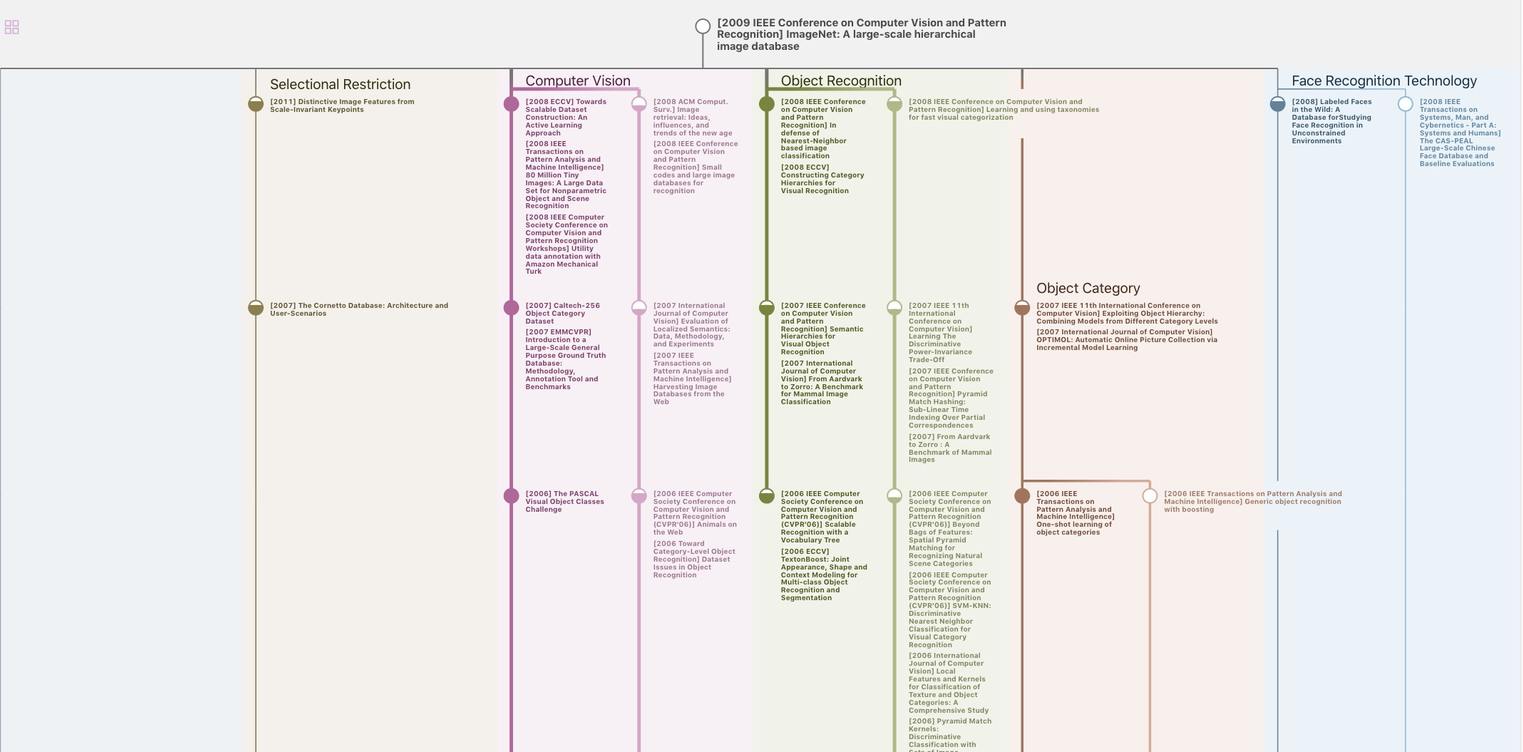
生成溯源树,研究论文发展脉络
Chat Paper
正在生成论文摘要