PVT-Crowd: Bridging Multi-scale Features from Pyramid Vision Transformer for Weakly-Supervised Crowd Counting
PATTERN RECOGNITION AND COMPUTER VISION, PRCV 2023, PT IX(2024)
摘要
Weakly-supervised crowd counting does not require locationlevel annotations, but only relies on count-level annotations to achieve the task of crowd counting for images, which is becoming a new research hotspot in the field of crowd counting. Currently, weakly-supervised crowd counting networks based on deep learning mostly use Transformers to extract features and establish global contexts, ignoring feature information at different scales, resulting in insufficient feature utilization. In this paper, we propose a well-designed end-to-end crowd counting network named PVT-Crowd bridging multi-scale features from the Pyramid Visual Transformer Encoder for weakly-supervised crowd counting. Specifically, Adjacent-Scale Bridging Modules (ASBM) enable the interaction of high-scale semantic and low-scale detailed information from both channel and spatial dimensions. The Global-Scale Bridging Module (GSBM) performs a secondary fusion of multi-scale feature information. Extensive experiments show that our PVT-Crowd outperforms most weakly-supervised crowd counting networks and obtains competitive performance compared to fully-supervised ones. In particular, crossdataset experiments confirm that our PVT-Crowd had a remarkable generality.
更多查看译文
关键词
crowd counting,multi-scale,transformer
AI 理解论文
溯源树
样例
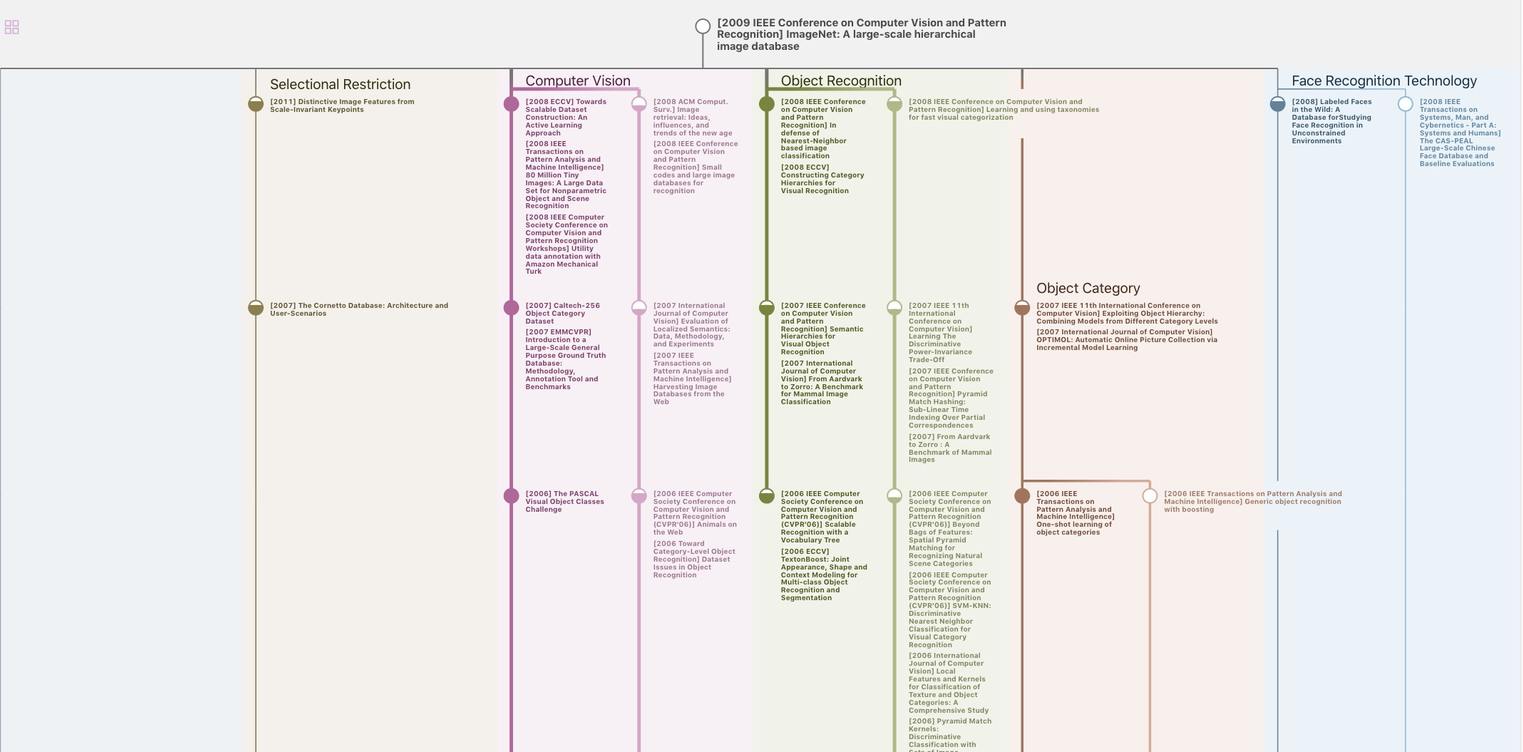
生成溯源树,研究论文发展脉络
Chat Paper
正在生成论文摘要