Learning Hierarchical Representations in Temporal and Frequency Domains for Time Series Forecasting
PATTERN RECOGNITION AND COMPUTER VISION, PRCV 2023, PT IX(2024)
摘要
Long-term time series forecasting is a critical task in many domains, including finance, healthcare, and weather forecasting. While Transformer-based models have made significant progress in time series forecasting, their high computational complexity often leads to compromises in model design, limiting the full utilization of temporal information. To address this issue, we propose a novel hierarchical decomposition framework that disentangles latent temporal variation patterns. Specifically, we decompose time series into trend and seasonal modes and further decompose seasonal temporal changes into coarse- and fine-grained states to capture different features of temporal sequences at different granularities. We use linear layers to embed local information for capturing fine-grained temporal changes and Fourier-domain attention to capture multi-periodic seasonal patterns to extract coarse-grained temporal dependency information. This forms a time series forecasting modeling from fine to coarse, and from local to global. Extensive experimental evaluation demonstrates that the proposed approach outperforms state-of-the-art methods on real-world benchmark datasets.
更多查看译文
关键词
Time series forecasting,hierarchical decomposition,fourier-domain attention,deep learning,supervised learning
AI 理解论文
溯源树
样例
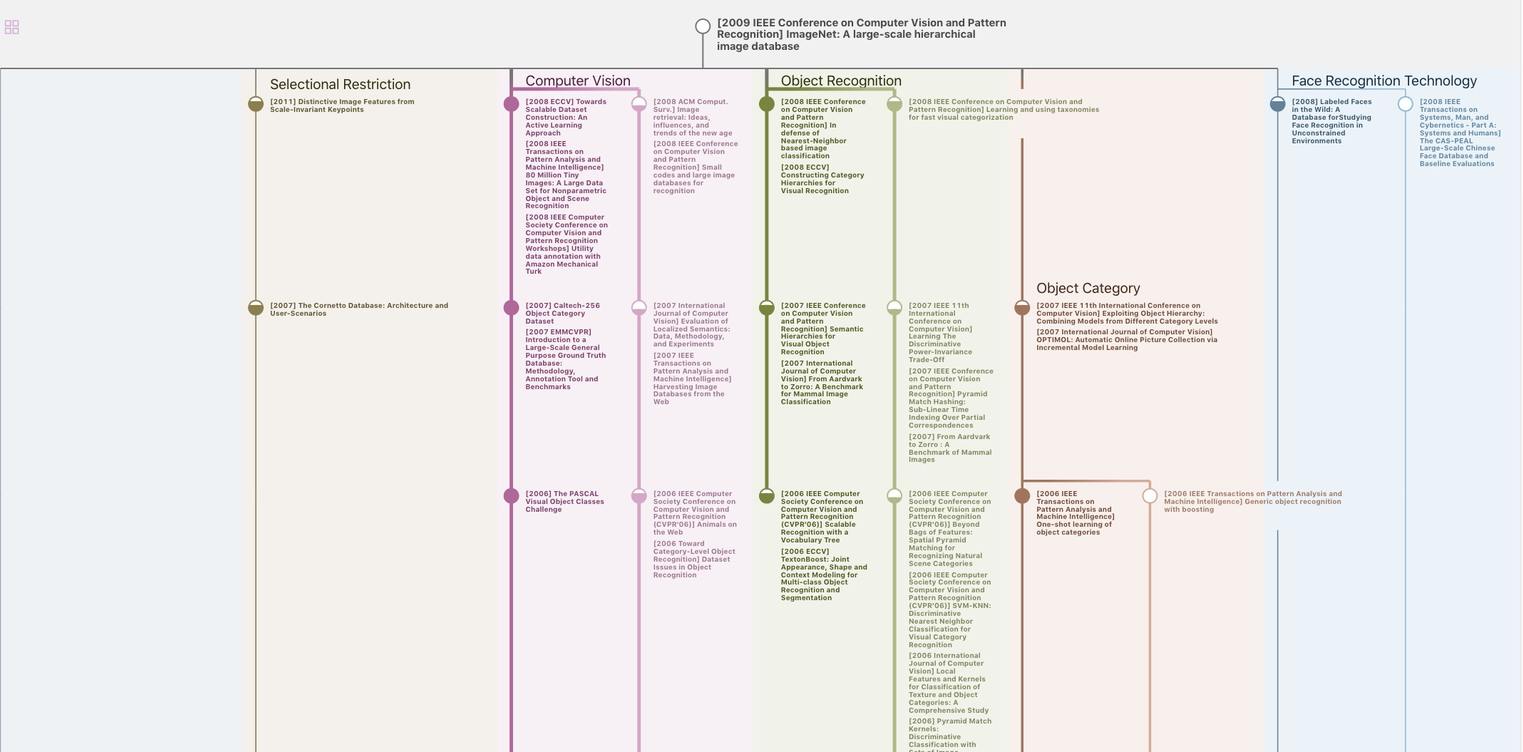
生成溯源树,研究论文发展脉络
Chat Paper
正在生成论文摘要