Spatial Feature Regularization and Label Decoupling Based Cross-Subject Motor Imagery EEG Decoding
PATTERN RECOGNITION AND COMPUTER VISION, PRCV 2023, PT XIII(2024)
摘要
Motor imagery (MI) serves as a vital approach to constructing brain-computer interfaces (BCIs) based on electroencephalogram (EEG) signals. However, the time-variant and label-coupling characteristics of EEG signals, combined with the limited sample sizes, often necessitate MI-EEG decoding across subjects. Unfortunately, existing methods encounter challenges related to interference from out-of-distribution features and feature-label coupling, resulting in the deterioration of decoding performance. To address these issues, this paper proposes a novel MI-EEG feature learning framework that focuses on decoupling features from labels and regularizing the feature representation. The proposed framework leverages aligned MI-EEG samples to extract Gaussian weighting regularized spatial features. Subsequently, a domain adaptation method is employed to decouple the extracted features from labels across different subjects' domains, thereby facilitating cross-subject MI-EEG decoding. To evaluate the effectiveness and efficiency of the proposed method, we conducted experiments using three benchmark MI-EEG datasets, consisting of four distinct groups of experiments. The experimental results demonstrate the effectiveness, efficiency, and parameter insensitivity of the proposed method, indicating its significant application value in the field of MI-EEG decoding.
更多查看译文
关键词
Motor Imagery,EEG Decoding,Pattern Recognition,Spatial Feature Regularization,Label Decoupling
AI 理解论文
溯源树
样例
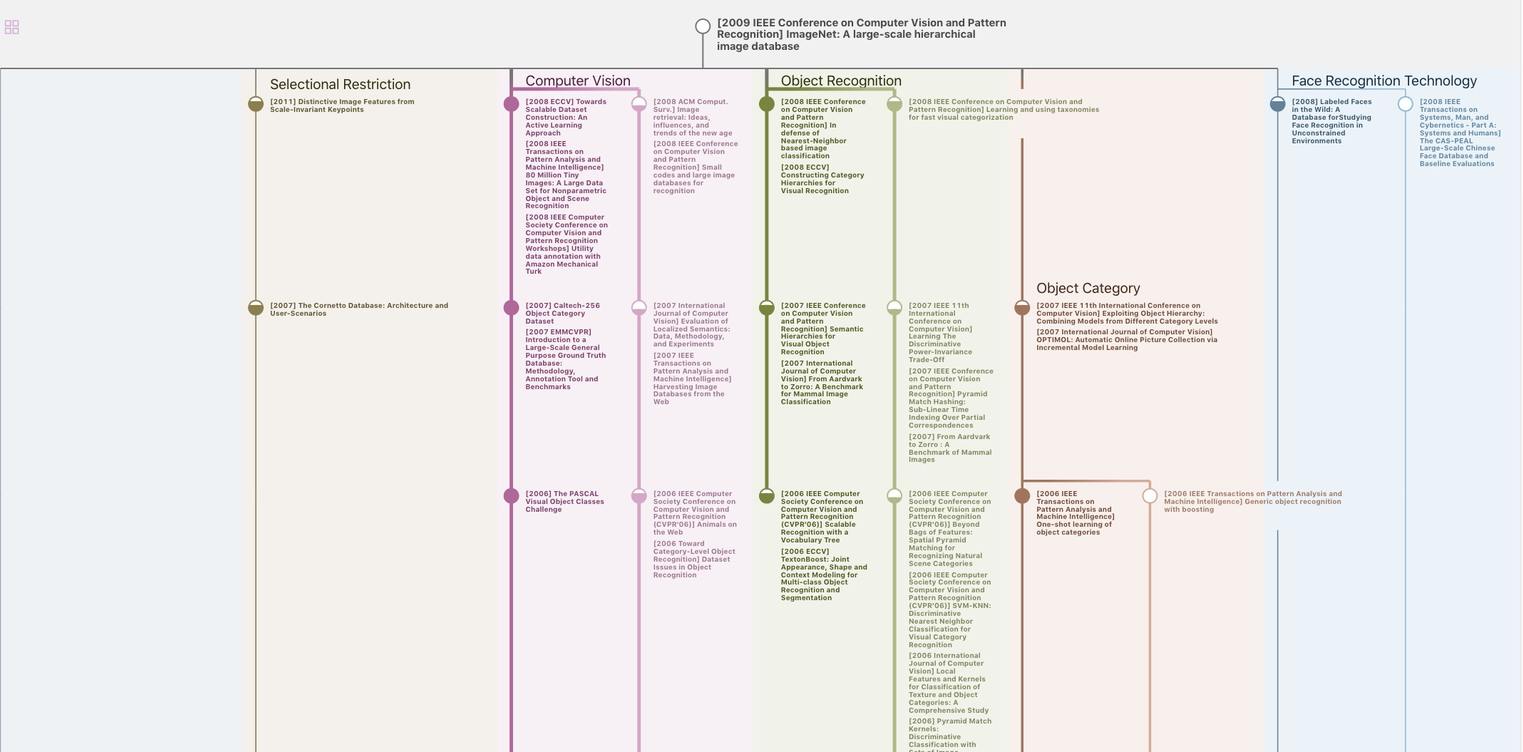
生成溯源树,研究论文发展脉络
Chat Paper
正在生成论文摘要