Frequency Domain Distillation for Data-Free Quantization of Vision Transformer
PATTERN RECOGNITION AND COMPUTER VISION, PRCV 2023, PT VIII(2024)
摘要
The increasing size of deep learning models has made model compression techniques increasingly important. Neural network quantization is a technique that can significantly compress models while preserving their original precision. However, conventional quantization methods relies on real training data, making it unsuitable for scenarios where data is unavailable. Data-Free quantization methods address this issue by synthesizing pseudo data to calibrate or fine tune the quantized model. However, these methods overlook an important problem, i.e., the mismatch between the low-frequency and high-frequency components of the synthesized pseudo data. This is due to the simultaneous optimization of low-frequency and high-frequency information, which can interfere with each other. We analyze the reasons behind this phenomenon and propose a frequency domain distillation (FDD) method to address this issue. Specifically, we first optimize the low-frequency component, followed by the high-frequency component, and employ distillation to make the high-frequency component more consistent with the low-frequency component. Additionally, we apply a progressive optimization strategy by gradually increasing the optimized region of pseudo data. We achieved state-of-the-art results on all the Vit models involved in our experiments, and complete ablation study also demonstrated the effectiveness of our method. Our code can be found at here.
更多查看译文
关键词
Model compression,Data-free quantization,Vision transformer
AI 理解论文
溯源树
样例
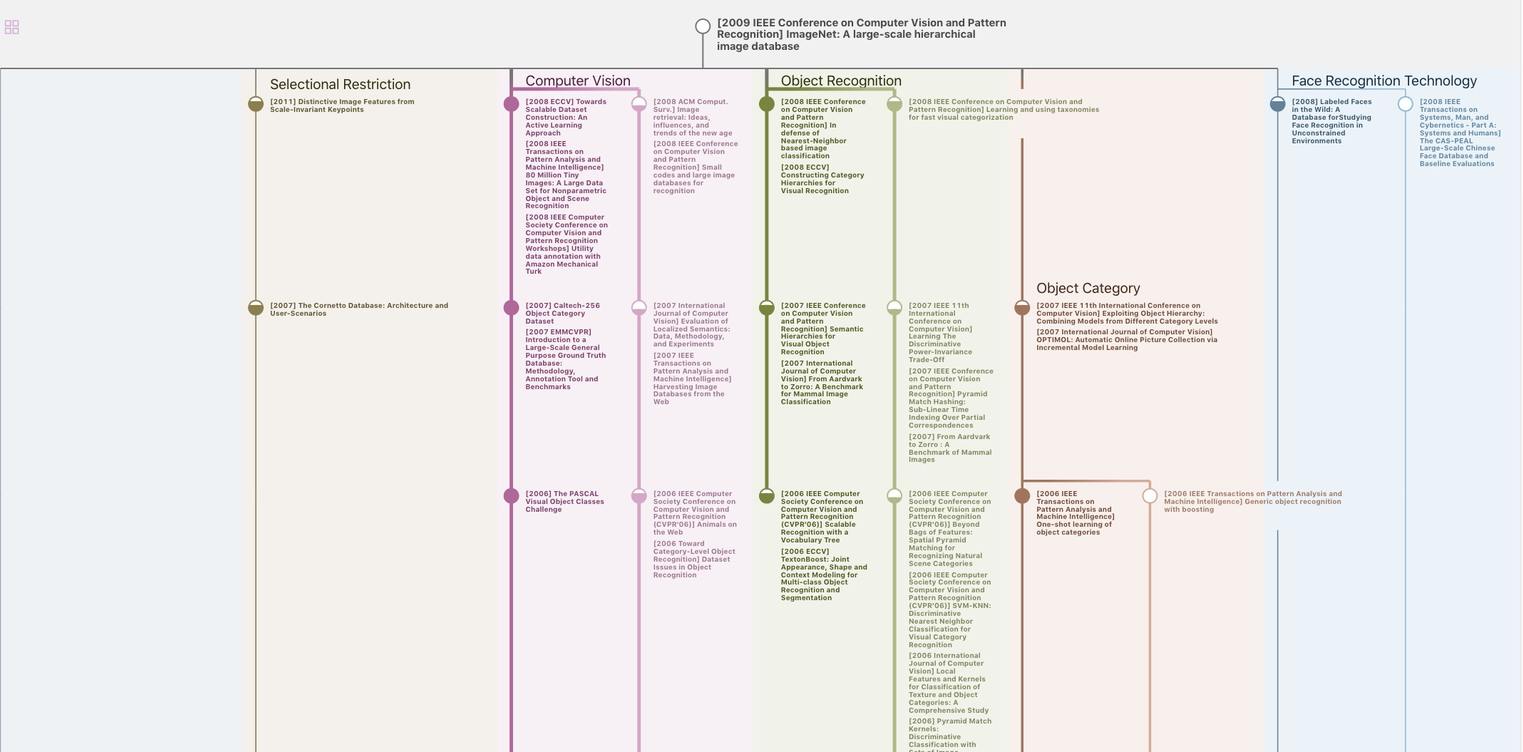
生成溯源树,研究论文发展脉络
Chat Paper
正在生成论文摘要