Global Variational Convolution Network for Semi-supervised Node Classification on Large-Scale Graphs
PATTERN RECOGNITION AND COMPUTER VISION, PRCV 2023, PT VIII(2024)
摘要
Graph Neural Networks (GNNs) have received much attention in the graph deep learning. However, there are some issues in extending traditional aggregation-based GNNs to large-scale graphs. With the rapid increase of neighborhood width, we find that the direction of aggregation can be disrupted and quite unbalanced, which compromises graphic structure and feature representation. This phenomenon is referred to Receptive Field Collapse. In order to preserve more structural information on large-scale graphs, we propose a novel Global Variational Convolutional Networks (GVCNs) for Semi-Supervised Node Classifications, which consists of a variational aggregation mechanism and a guidance learning mechanism. Variational aggregation can moderately map the unbalanced neighborhood distribution to a prior distribution. And the guidance learning mechanism, based on positive pointwise mutual information (PPMI), encourages the model to concentrate on more prominent graphic structures, which increases information entropy and alleviates Receptive Field Collapse. In addition, we propose a variational convolutional kernel to achieve effective global aggregation. Finally, we evaluate GVCNs on the Open Graph Benchmark (OGB) Arxiv and Products datasets. Up to the submission date (Jan 20, 2023), GVCNs achieve significant performance improvements compared to other aggregation-based GNNs, even state-of-the-art decoupling-based methods, the performance of GVCNs remains competitive with moderate spatiotemporal complexity. Our code can be obtained from: https://github.com/YideQiu/GVCN.
更多查看译文
关键词
Large-scale Graphs,Variational,Semi-Supervised Classification
AI 理解论文
溯源树
样例
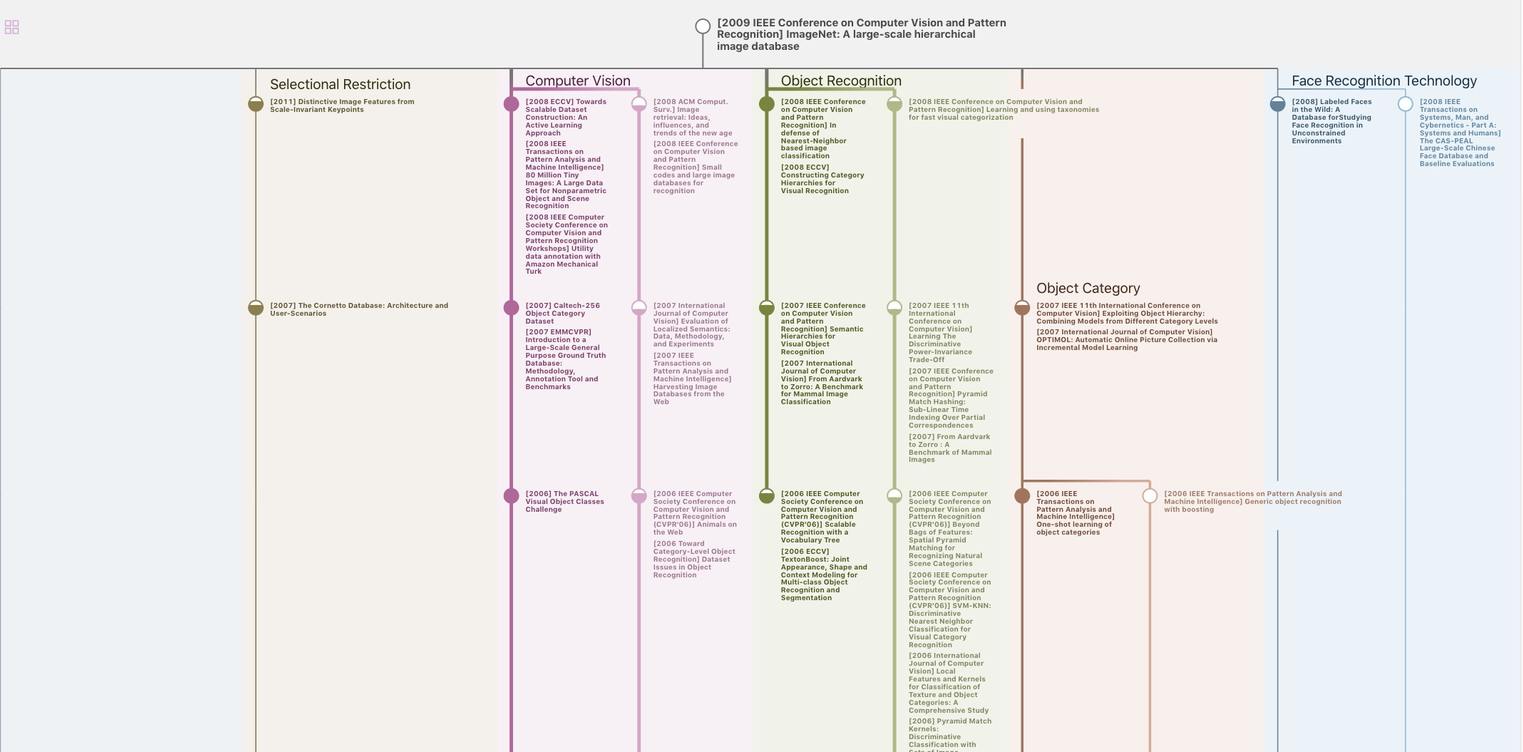
生成溯源树,研究论文发展脉络
Chat Paper
正在生成论文摘要