Grassmann Graph Embedding for Few-Shot Class Incremental Learning
PATTERN RECOGNITION AND COMPUTER VISION, PRCV 2023, PT VIII(2024)
摘要
Few-shot class incremental learning (FSCIL) poses a significant challenge in machine learning as it involves acquiring new knosstabwledge from limited samples while retaining previous knowledge. However, the scarcity of data for new classes not only leads to overfitting but also exacerbates catastrophic forgetting during incremental learning. To tackle these challenges, we propose the Grassmann Graph Embedding framework for Few-shot Class Incremental Learning (GGE-FSCIL), which effectively preserves the geometric properties and structural relationships of learned knowledge. Unlike most existing approaches that optimize network parameters in the Euclidean space, we leverage manifold space for optimizing the incremental learning network. Specifically, we embed the approximated characteristics of each class onto a Grassmann manifold, enabling the preservation of intra-class knowledge and enhancing adaptability to new tasks with few-shot samples. Recognizing that knowledge exists within interconnected relationships, we construct a neighborhood graph on the Grassmann manifold to maintain interclass structure information, thereby alleviating catastrophic forgetting. We extensively evaluated our method on CIFAR100, miniImageNet, and CUB200 datasets, and the results demonstrate that our approach surpasses the state-of-the-art methods in few-shot class incremental learning.
更多查看译文
关键词
shot Class Incremental Learning,Grassmann Manifold,Graph Embedding
AI 理解论文
溯源树
样例
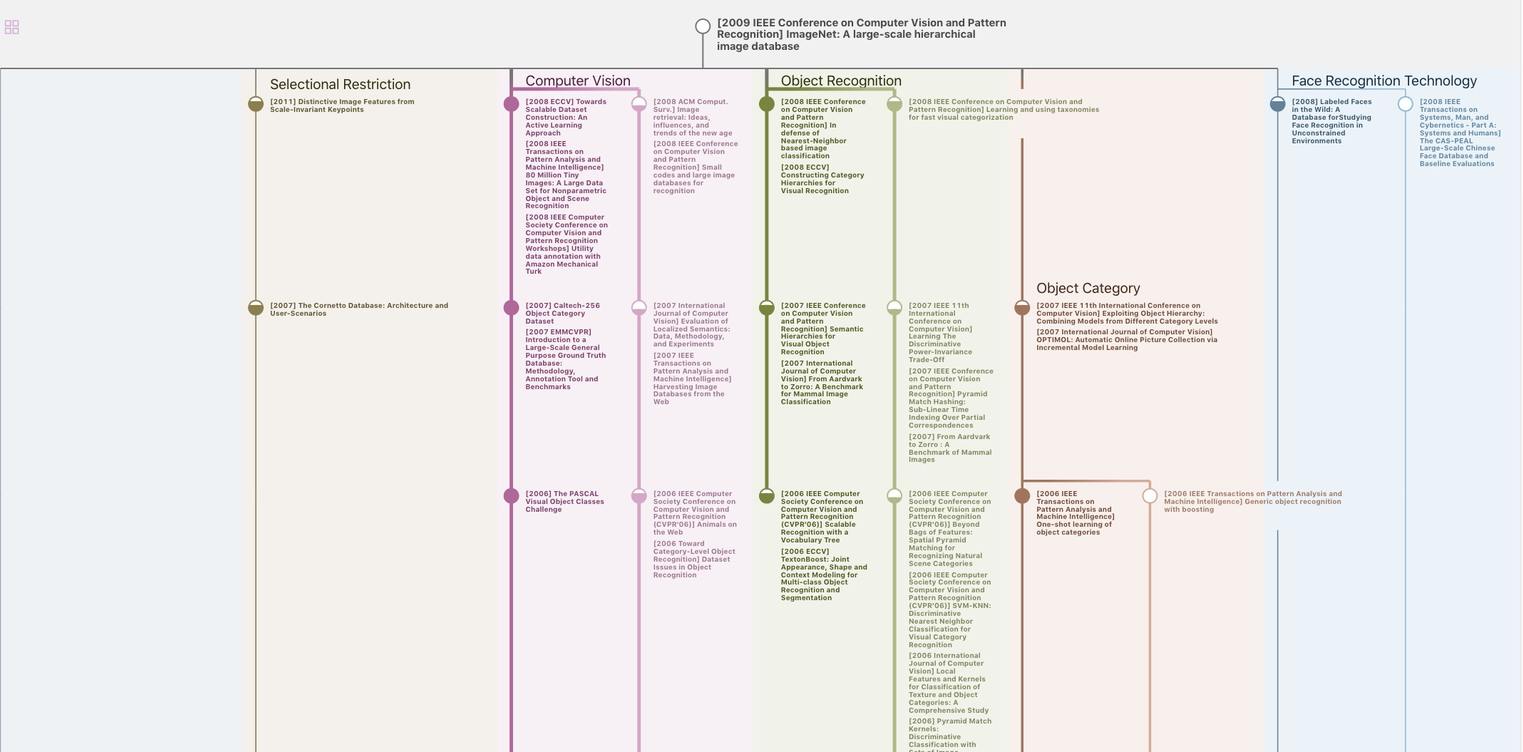
生成溯源树,研究论文发展脉络
Chat Paper
正在生成论文摘要