A Multi-modality Driven Promptable Transformer for Automated Parapneumonic Effusion Staging
PATTERN RECOGNITION AND COMPUTER VISION, PRCV 2023, PT XIII(2024)
摘要
Pneumonia is a prevalent disease, and some pneumonia patients develop parapneumonic effusion. Among patients with pneumonia, the mortality rate of patients with parapneumonic effusion is higher than that of patients without parapneumonic effusions. Parapneumonic effusion is classified as the uncomplicated stage or complicated stage. Patients with complicated parapneumonic effusion necessitate pleural drainage, while patients with uncomplicated parapneumonic effusion only require antibiotic treatment. Consequently, staging parapneumonic effusion plays a crucial role in reducing mortality among pneumonia patients. The previous method employs convolutional neural networks to extract features from CT slice and graph neural networks for classifying the slice-level feature sequence in parapneumonic effusion staging. However, we argue that transformers outperform graph neural networks in feature sequence classification. Thus, we integrate transformers into our method. Distinguishing between uncomplicated and complicated parapneumonic effusions based solely on CT slices is challenging due to their similar appearance. Therefore, we incorporate additional information in the form of a prompt to aid in the staging process. This allows us to propose a promptable model for parapneumonic effusion staging that incorporates multimodal information. Our method surpasses previous approaches, achieving impressive results with an F1-score of 92.72 and an AUC of 96.67.
更多查看译文
关键词
Deep learning,Medical image processing,Pneumonia effusion staging
AI 理解论文
溯源树
样例
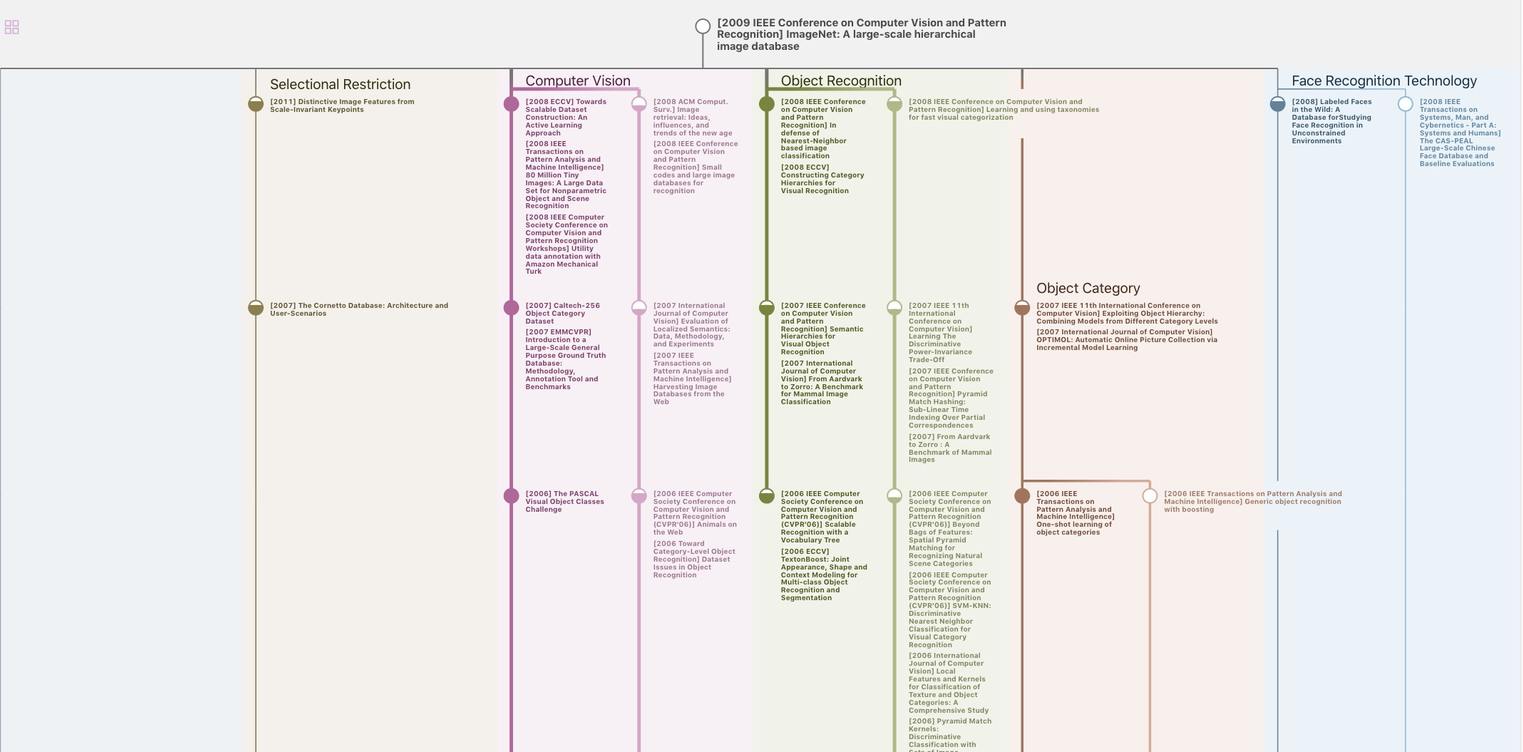
生成溯源树,研究论文发展脉络
Chat Paper
正在生成论文摘要