Knowledge Distillation of Attention and Residual U-Net: Transfer from Deep to Shallow Models for Medical Image Classification
PATTERN RECOGNITION AND COMPUTER VISION, PRCV 2023, PT XIII(2024)
摘要
With the widespread application of deep learning in medical image analysis, its capacity to handle high-dimensional and complex medical images has been widely recognized. However, high-accuracy deep learning models typically demand considerable computational resources and time, while shallow models are generally unable to compete with complex model. To overcome these challenges, this paper introduces a Knowledge Distillation methodology that merges features and soft labels, transfering knowledge encapsulated in the intermediate features and predictions of the teacher model to the student model. The student model imitates the teacher model's behavior, thereby improving its prediction accuracy. Based on this, we propose the Res-Transformer teacher model bases on the U-Net architecture and the ResU-Net student model incorporates Residuals. The Res-Transformer model employs dual Attention to acquire deep feature maps of the image, and subsequently employs Hierarchical Upsampling to restore the details in these feature maps. The ResU-Net model enhances stability via Residuals and recovers the loss of image information in convolution operations through optimized skip-connection. Finally, we evaluate on multiple disease datasets. The results show that the Res-Transformer achieves accuracy up to 94.3%. By applying knowledge distillation, abundant knowledge from the Res-Transformer is transferring knowledge of the Res-Transformer to the ResU-Net model, improving its accuracy up to 7.1%.
更多查看译文
关键词
Knowledge distillation,Medical image,U-Net,Residual,Attention
AI 理解论文
溯源树
样例
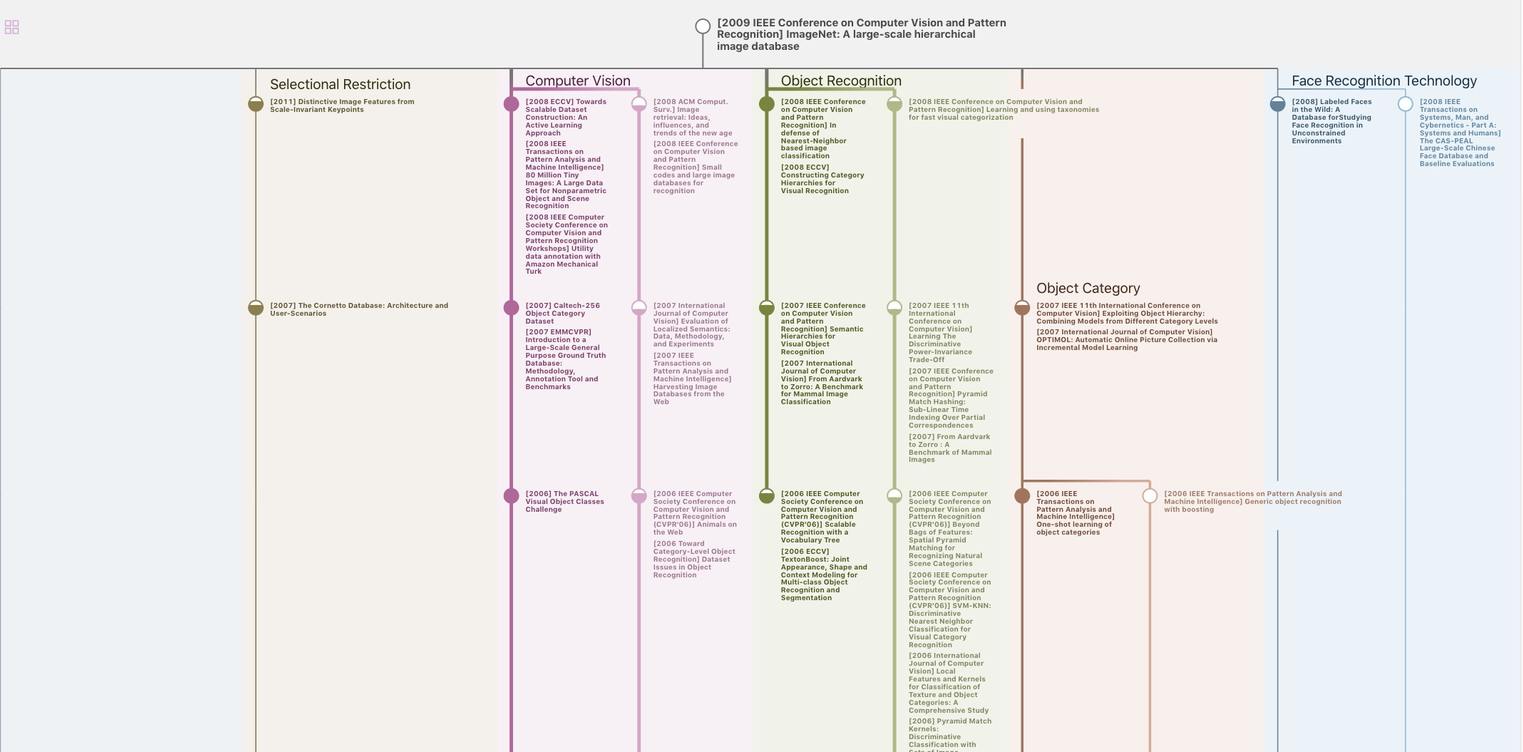
生成溯源树,研究论文发展脉络
Chat Paper
正在生成论文摘要