FlashViT: A Flash Vision Transformer with Large-Scale Token Merging for Congenital Heart Disease Detection
PATTERN RECOGNITION AND COMPUTER VISION, PRCV 2023, PT XIII(2024)
摘要
Congenital heart disease (CHD) is the most common congenital malformation and imaging examination is an important means to diagnose it. Currently, deep learning-based methods have achieved remarkable results in various types of imaging examinations. However, the issues of large parameter size and low throughput limit their clinical applications. In this paper, we design an efficient, light-weight hybrid model named FlashViT, to assist cardiovascular radiologists in early screening and diagnosis of CHD. Specifically, we propose the Large-scale Token Merging Module (LTM) for more aggressive similar token merging without sacrificing accuracy, which alleviate the problem of high computational complexity and resource consumption of self-attention mechanism. In addition, we propose an unsupervised homogenous pre-training strategy to tackle the issue of insufficient medical image data and poor generalization ability. Compared with conventional pre-training strategy that use ImageNet1K, our strategy only utilizes less than 1% of the class-agnostic medical images from ImageNet1K, resulting in faster convergence speed and advanced performance of the model. We conduct extensive validation on the collected CHD dataset and the results indicate that our proposed FlashViT-S achieves accuracy of 92.2% and throughput of 3753 fps with about 3.8 million parameters. We hope that this work can provide some assistance in designing laboratory models for future application in clinical practice.
更多查看译文
关键词
Congenital Heart Disease Detection,Large-scale Token Merging Module,Homologous Pre-training Strategy
AI 理解论文
溯源树
样例
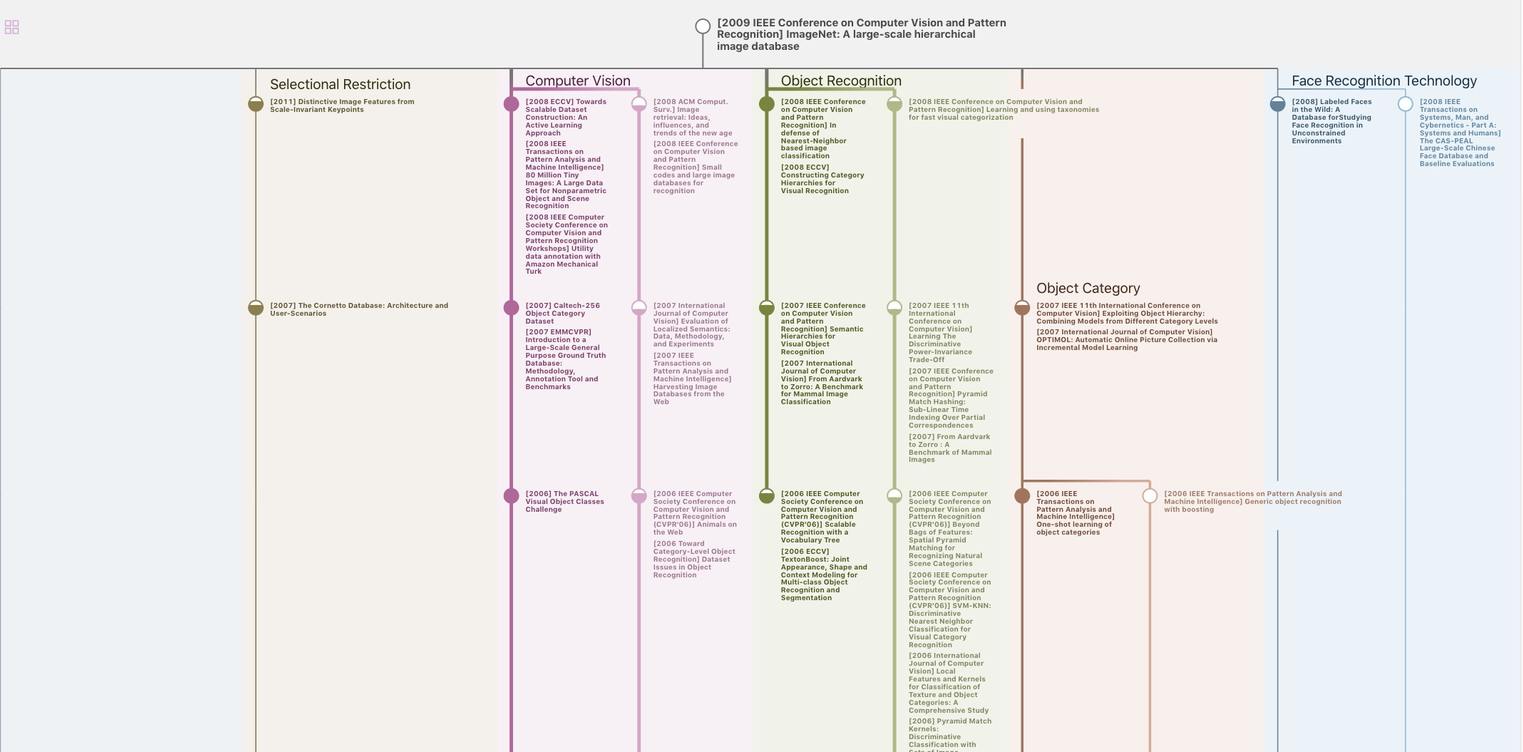
生成溯源树,研究论文发展脉络
Chat Paper
正在生成论文摘要