An Evolutionary Multiobjective Optimization Algorithm Based on Manifold Learning
PATTERN RECOGNITION AND COMPUTER VISION, PRCV 2023, PT VII(2024)
摘要
Multi-objective optimization problem is widespread in the real world. However, plenty of typical evolutionary multi-objective optimization (EMO) algorithms are extremely tough to deal with large-scale optimization problems (LSMOPs) due to the curse of dimensionality. In reality, the dimension of the manifold representing the Pareto solution set is much lower than that of the decision space. This work proposes a decision space reduction technique based on manifold learning using locality-preserving projects. The critical insight is to improve search efficiency through decision space reduction. The high-dimensional decision space is first mapped to a low-dimensional subspace for a more effective search. Subsequently, a transformation matrix which is Pseudo-inverse of the projection matrix, maps the resultant offspring solutions back to the primal decision space. The proposed decision space reduction technique can be integrated with most multi-objective evolutionary algorithms. This paper integrates it with NSGA-II, namely LPP-NSGA-II. We compare the proposed LPP-NSGA-II with four state-of-the-art EMO algorithms on thirteen test problems. The experimental results reveal the effectiveness of the proposed algorithm.
更多查看译文
关键词
Manifold learning,Evolutionary algorithm,Large-scale multi-objective optimization,Decision space reduction
AI 理解论文
溯源树
样例
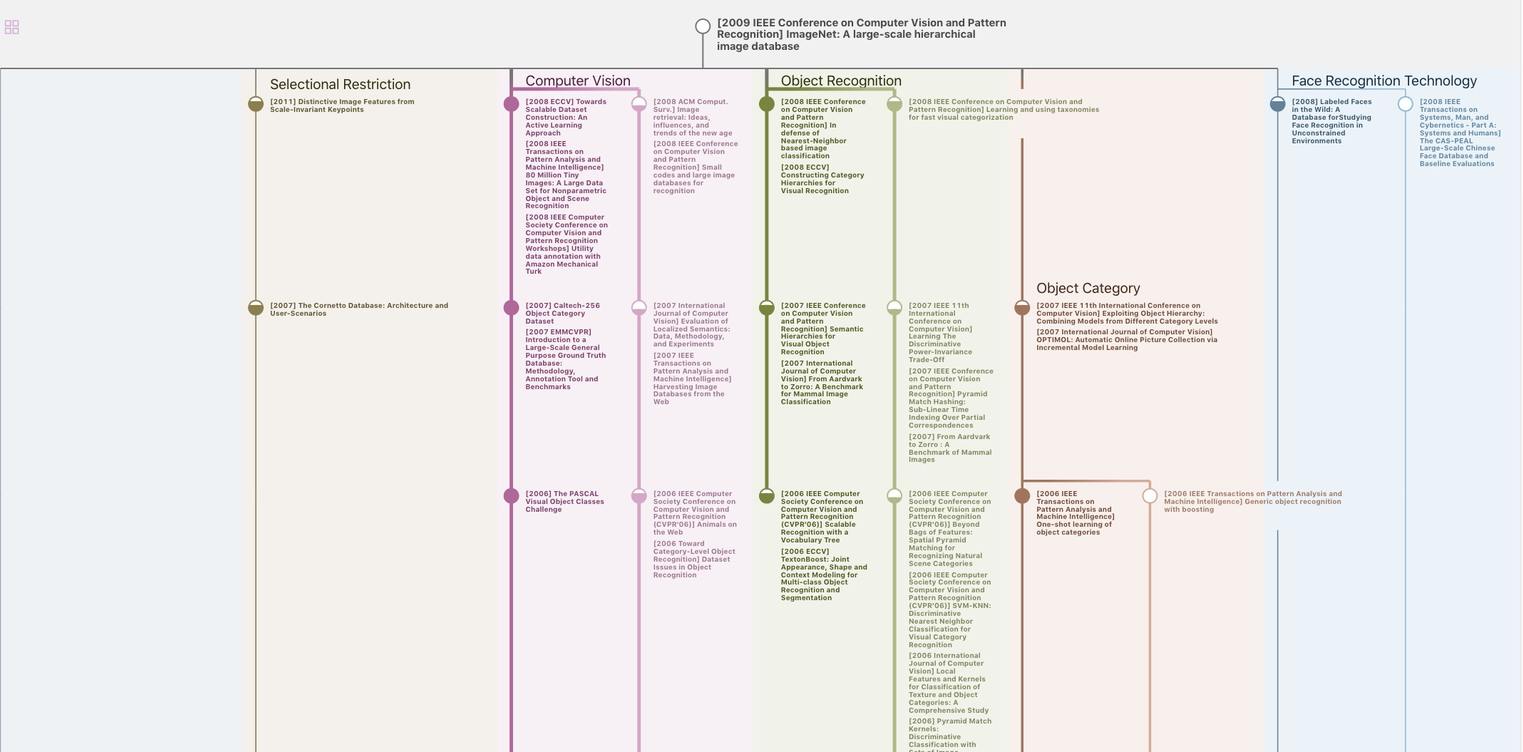
生成溯源树,研究论文发展脉络
Chat Paper
正在生成论文摘要