Deep Hough Transform for Gaussian Semantic Box-Lines Alignment
PATTERN RECOGNITION AND COMPUTER VISION, PRCV 2023, PT VII(2024)
摘要
Image correction and trimming for the digitization of paper-based records is a challenging task. Existing models and techniques for object detection are limited and cannot be applied directly. For example, non-directional bounding boxes cannot correct skewed images, paper-based scanned images with complex boundaries (torn pages and damaged edges) cannot locate obvious bounding boxes, resizing high-resolution image leads to accuracy errors, etc. To this end, in this paper, we proposed a Boundary Detection Network (BDNet) based on deep hough transform, which implements semantic boundary detection with geometrical restriction in a coarse-to-fine mean. The model is mainly divided into two stages: coarse location and refined adjustment. The former predicts the boundaries' orientations and positions at the down-sampled image. The latter refines the boundary positions using the image patches sampled from the coarsely-located boundary in the original image. Among them, each stage contains two main modules: The Semantic Box-Lines (SBL) utilizes Gaussian heatmaps to capture extensive boundary semantic information, while the Deep Hough Alignment (DHA) efficiently extracts global line orientations to align semantic boundaries (box or lines). Detailed experiments and metric analysis verify that our proposed model is effective and feasible on the open-source datasets we collected, i.e., for scanned images with a resolution of 2500x3500 pixels, our method can accurately locate content boundaries and achieve 0.95 IoU accuracy.
更多查看译文
关键词
Image correction and trimming,Object detection,Deep hough transform,Semantic boundary,Gaussian heatmap
AI 理解论文
溯源树
样例
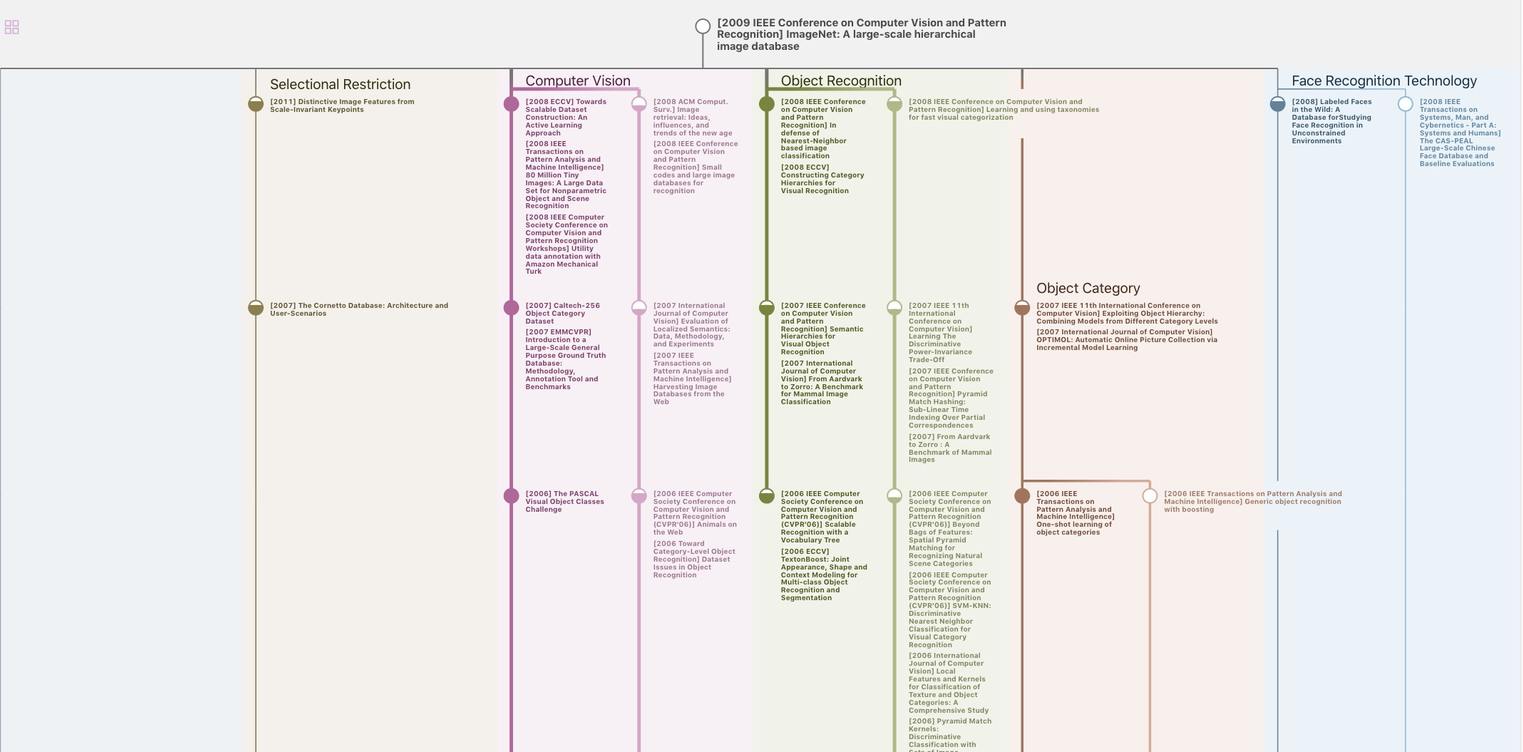
生成溯源树,研究论文发展脉络
Chat Paper
正在生成论文摘要