DBDAN: Dual-Branch Dynamic Attention Network for Semantic Segmentation of Remote Sensing Images
PATTERN RECOGNITION AND COMPUTER VISION, PRCV 2023, PT IV(2024)
摘要
Attention mechanism is capable to capture long-range dependence. However, its independent calculation of correlations can hardly consider the complex background of remote sensing images, which causes noisy and ambiguous attention weights. To address this issue, we design a correlation attention module (CAM) to enhance appropriate correlations and suppress erroneous ones by seeking consensus among all correlation vectors, which facilitates feature aggregation. Simultaneously, we introduce the CAM into a local dynamic attention (LDA) branch and a global dynamic attention (GDA) branch to obtain the information on local texture details and global context, respectively. In addition, considering the different demands of complex and diverse geographical objects for both local texture details and global context, we devise a dynamic weighting mechanism to adaptively adjust the contributions of both branches, thereby constructing a more discriminative feature representation. Experimental results on three datasets suggest that the proposed dual-branch dynamic attention network (DBDAN), which integrates the CAM and both branches, can considerably improve the performance for semantic segmentation of remote sensing images and outperform representative state-of-the-art methods.
更多查看译文
关键词
Remote Sensing,Semantic Segmentation,Attention Mechanism,Deep Learning
AI 理解论文
溯源树
样例
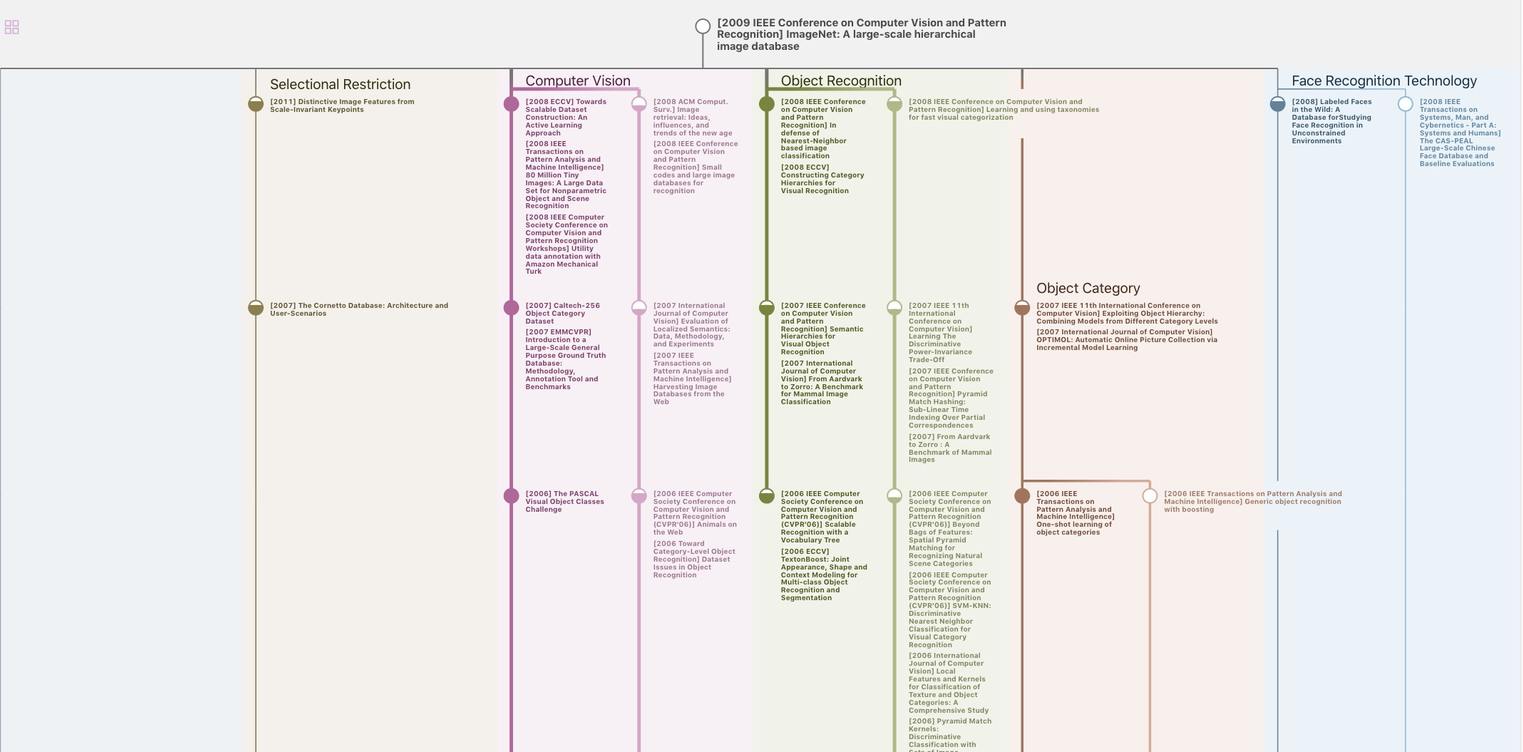
生成溯源树,研究论文发展脉络
Chat Paper
正在生成论文摘要