Improved Conditional Generative Adversarial Networks for SAR-to-Optical Image Translation
PATTERN RECOGNITION AND COMPUTER VISION, PRCV 2023, PT IV(2024)
摘要
Synthetic aperture radar (SAR) has the potential to operate effectively in all weather conditions, making it a desirable tool in various fields. However, the inability of untrained individuals to visually identify ground cover in SAR images poses challenges in practical applications such as environmental monitoring, disaster assessment, and land management. To address this issue, generative adversarial networks (GANs) have been used to transform SAR images into optical images. This technique is commonly referred to as SAR to optical image translation. Despite its common use, the traditional methods often generate optical images with color distortion and blurred contours. Therefore, a novel approach utilizing conditional generative adversarial networks (CGANs) is introduced as an enhanced method for SAR-to-optical image translation. A style-based calibration module is incorporated, which learns the style features of the input SAR images and matches them with the style of real optical images to achieve color calibration, thereby minimizing the differences between the generated output and real optical images. Furthermore, a multi-scale strategy is incorporated in the discriminator. Each branch of the multi-scale discriminator is dedicated to capturing texture and edge features at different scales, thereby enhancing the texture and edge information of the image at both local and global levels. Experimental results demonstrate that the proposed approach surpasses existing image translation techniques in terms of both visual effects and evaluation metrics.
更多查看译文
关键词
Optical images,Synthetic Aperture Radar (SAR),SAR-to-optical translation,Conditional Generative Adversarial Network (CGANs)
AI 理解论文
溯源树
样例
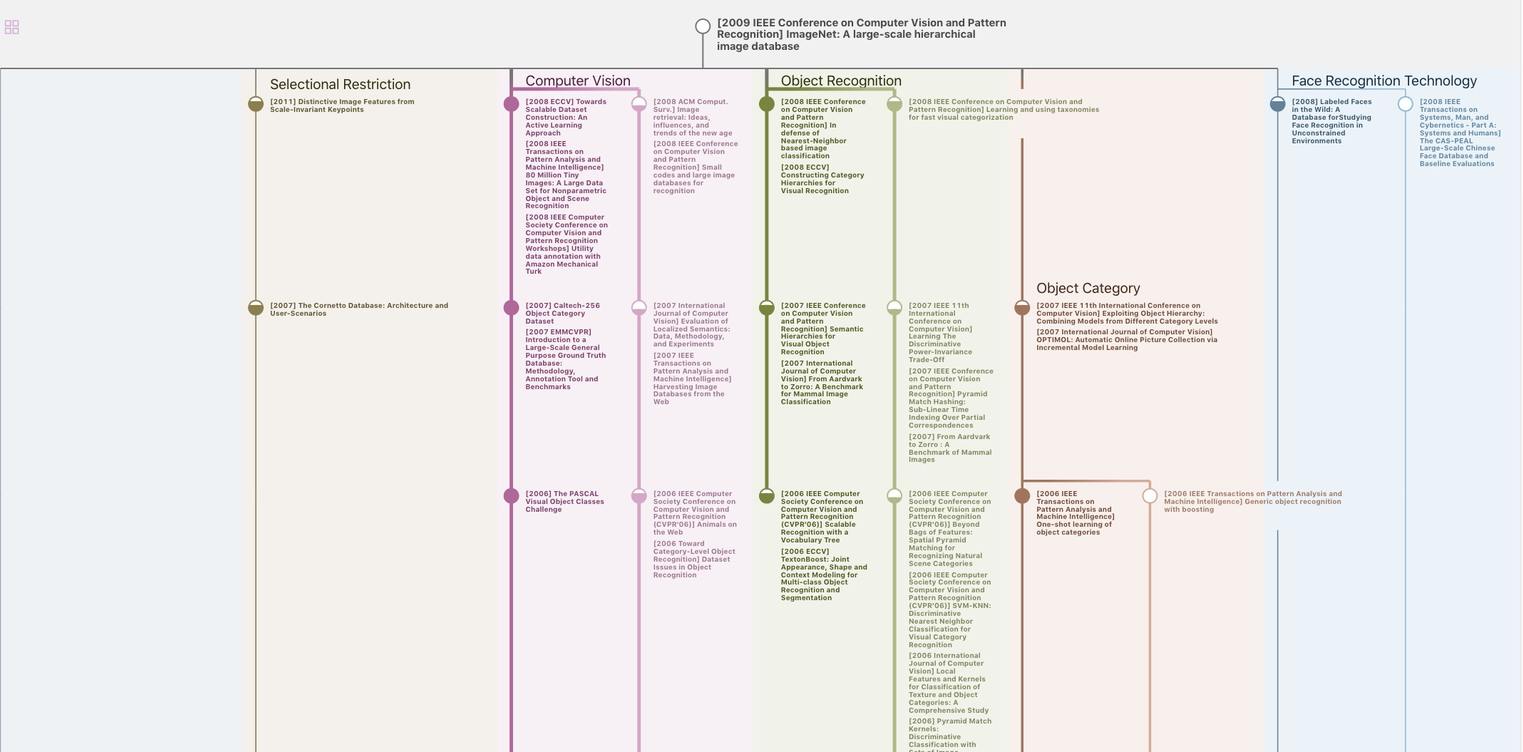
生成溯源树,研究论文发展脉络
Chat Paper
正在生成论文摘要