Exploring Frequency Attention Learning and Contrastive Learning for Face Forgery Detection
PATTERN RECOGNITION AND COMPUTER VISION, PRCV 2023, PT V(2024)
摘要
Face forgery detection has become a critical security concern due to advances in manipulation techniques. Most methods look for forged clues from the spatial or vanilla frequency domain, leading to serious over-fitting. In this paper, we propose a Frequency Attention Module (FAM) that enhances model generalizability in face forgery detection. We theoretically demonstrate the feasibility of frequency attention learning, which allows the network to automatically refine subtle but discriminative forged features and suppress irrelevant components in the frequency domain without complex manual partitions. Besides, considering that commonly-used cross-entropy loss neglects the intra-class compactness, we design the DeepFake Contrastive Loss (DFCL) to decrease intra-class variances for real faces and enlarge inter-class differences in the feature space. Extensive experiments show that our method significantly outperforms SoTA methods on widely-used benchmarks.
更多查看译文
关键词
Face Forgery Detection,Frequency Attention Learning,Contrastive Learning
AI 理解论文
溯源树
样例
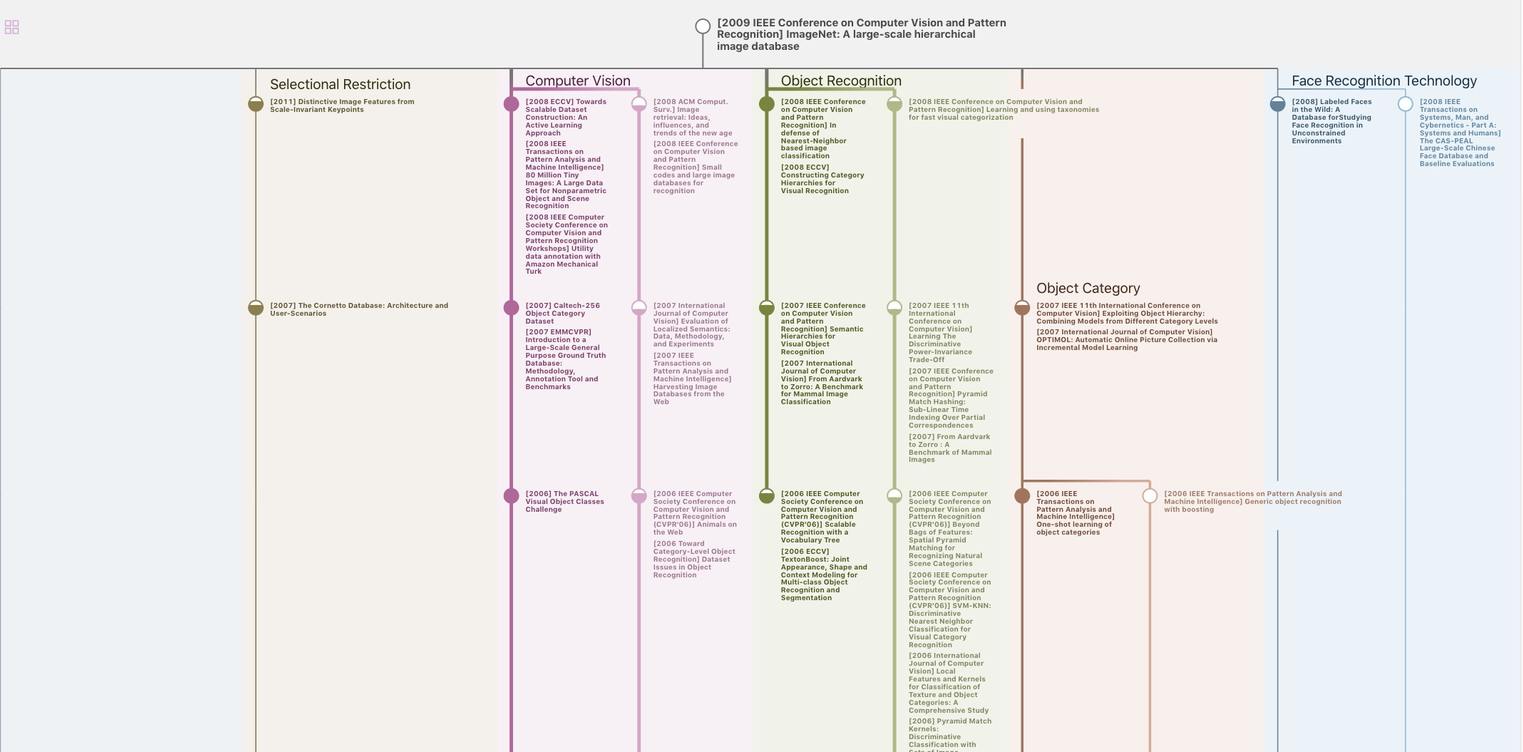
生成溯源树,研究论文发展脉络
Chat Paper
正在生成论文摘要