Shared Nearest Neighbor Calibration for Few-Shot Classification
PATTERN RECOGNITION AND COMPUTER VISION, PRCV 2023, PT IV(2024)
摘要
Few-shot classification aims to classify query samples using very few labeled examples. Most existing methods follow the Prototypical Network to classify query samples by matching them to the nearest centroid. However, scarce labeled examples tend to bias the centroids, which leads to query samples matching the wrong centroids. In this paper, we address the mismatching problem of centroids and query samples by optimizing the matching strategy. The idea is to combine the Shared Nearest Neighbor similarity with cosine similarity proportionally to calibrate the matching results of the latter. Furthermore, we also improve a bias-diminishing approach to increase the number of shared nearest neighbors between query samples and the centroid of their class. We validate the effectiveness of our method with extensive experiments on three few-shot classification datasets: miniImageNet, tieredImageNet, and CUB-200-2011 (CUB). Moreover, our method has achieved competitive performance across different settings and datasets.
更多查看译文
关键词
Few-shot classification,metric learning,transductive
AI 理解论文
溯源树
样例
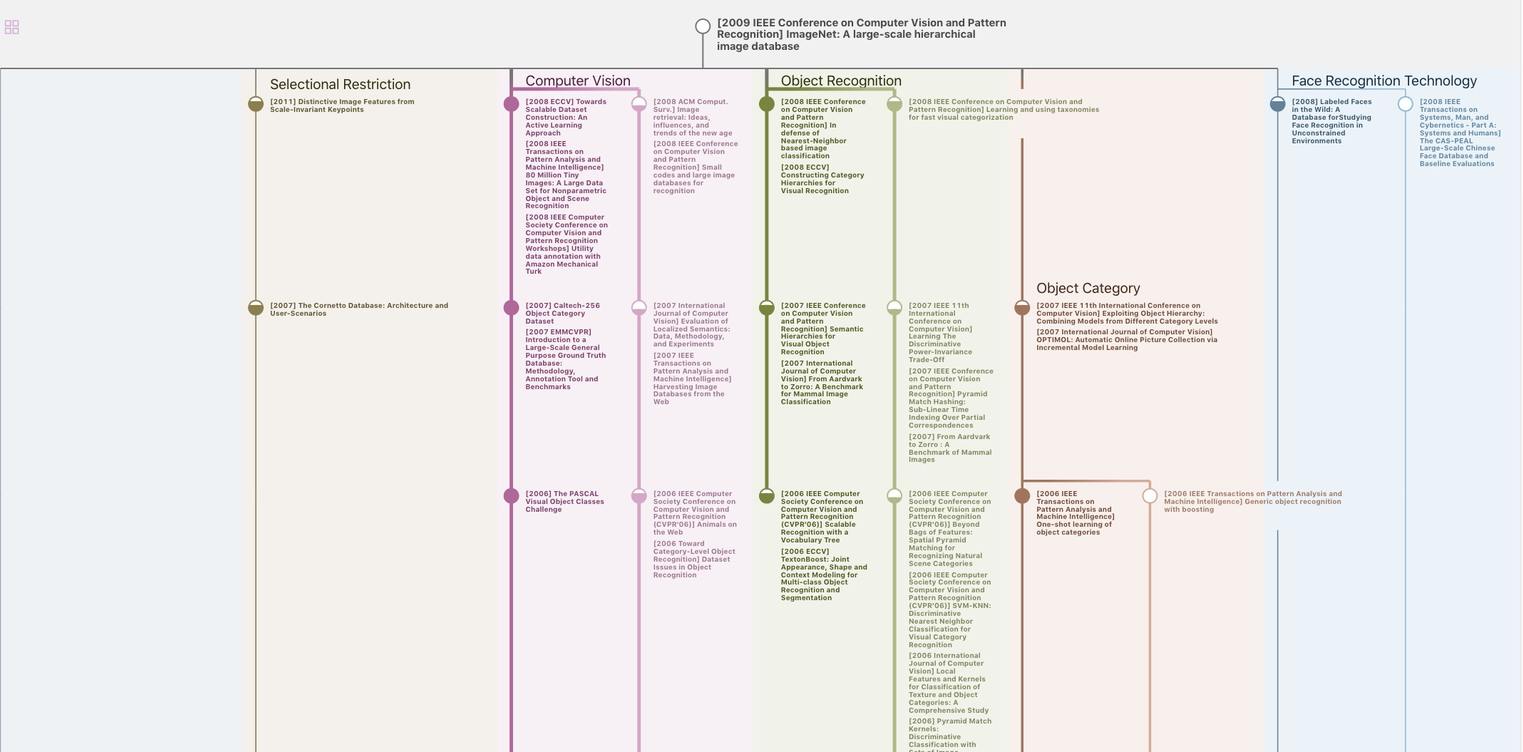
生成溯源树,研究论文发展脉络
Chat Paper
正在生成论文摘要