Minimum Assumption Reconstruction Attacks: Rise of Security and Privacy Threats Against Face Recognition
PATTERN RECOGNITION AND COMPUTER VISION, PRCV 2023, PT V(2024)
摘要
Facial Recognition (FR), despite its remarkable precision and advancements achieved through deep learning, exhibits vulnerability to security threats, specifically originating from deep generative models proficient in synthesizing deceptive face images. Generative Adversarial Networks (GANs) present substantial risks by showcasing the capacity to exploit potential vulnerabilities within FR systems. While the existing research primarily focuses on the scenario of a compromised database facilitating facial reconstruction attacks, it often overlooks more realistic threats where adversaries attack with a limited number of queries without breaching the database. This work introduces Minimum Assumption Reconstruction Attacks (MARA), offering a realistic attack framework against FR systems. MARA treats an attacker as a regular user interacting with the FR system's user interface and observing the matching scores. We formulate the MARA attack as an optimization problem, aiming to find a latent vector in the W+ latent space of StyleGAN for generating adversarial face images that can bypass the targeted FR system. A latent space mining strategy is also proposed to enhance attack performance by obtaining 'good' initial guesses in the latent space. Our experiments show that MARA achieves performance comparable to false accept attacks while adhering to query limits and mimicking user-like interaction behavior. This study highlights the importance of considering attack models requiring minimal effort from the adversary, an essential perspective for adversarial research that seeks to guard against powerful and less resource-intensive attacks.
更多查看译文
关键词
Face Recognition Attack,Reconstruction Attack,Black-box Attack,White-box Attack
AI 理解论文
溯源树
样例
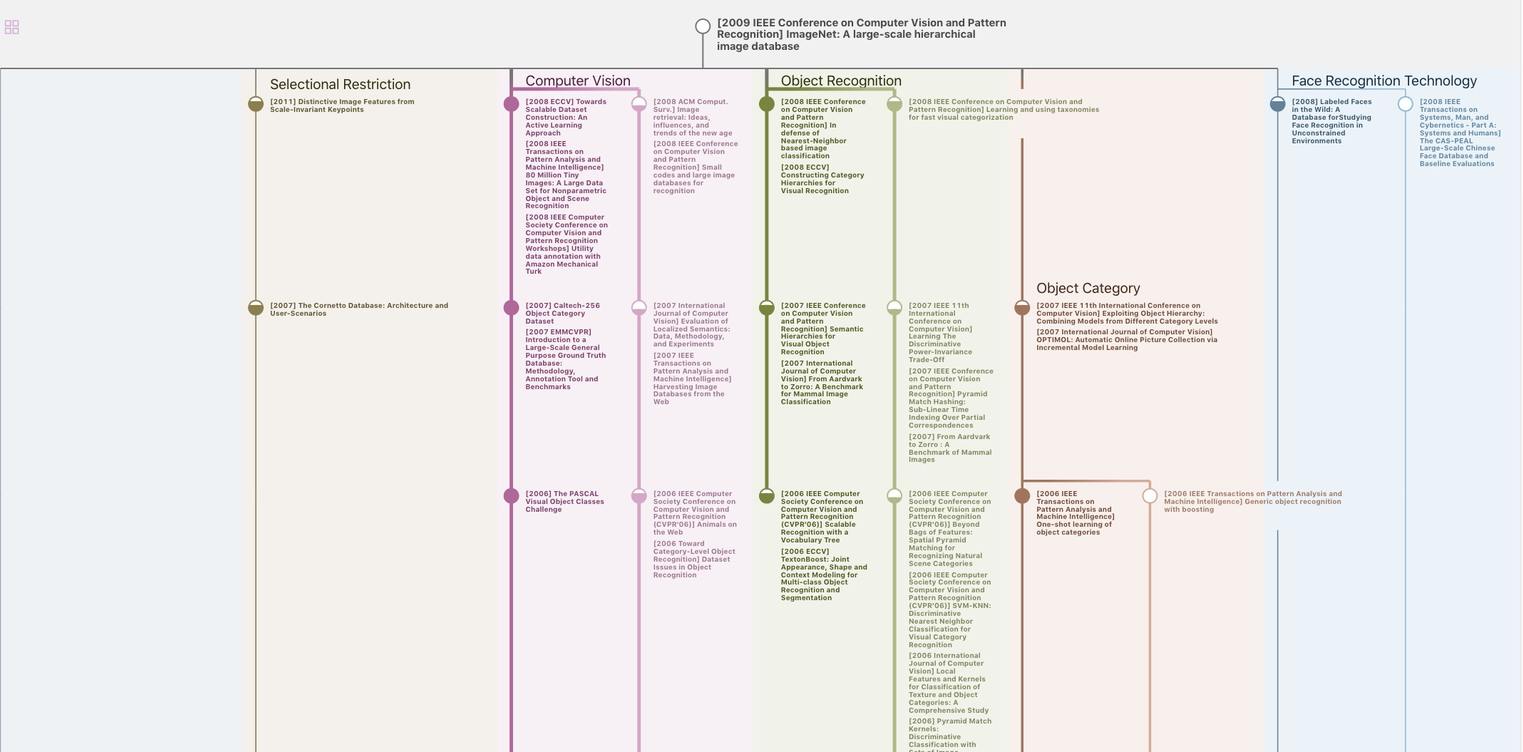
生成溯源树,研究论文发展脉络
Chat Paper
正在生成论文摘要