Inter-class Sparsity Based Non-negative Transition Sub-space Learning
PATTERN RECOGNITION AND COMPUTER VISION, PRCV 2023, PT III(2024)
摘要
Least squares regression has shown promising performance in the supervised classification. However, conventional least squares regression commonly faces two limitations that severely restrict their effectiveness. Firstly, the strict zero-one label matrix utilized in least squares regression provides limited freedom for classification. Secondly, the modeling process does not fully consider the correlations among samples from the same class. To address the above issues, this paper proposes the interclass sparsity-based non-negative transition sub-space learning (ICSNTSL) method. Our approach exploits a transition sub-space to bridge the raw image space and the label space. By learning two distinct transformation matrices, we obtain a low-dimensional representation of the data while ensuring model flexibility. Additionally, an inter-class sparsity term is introduced to learn a more discriminative projection matrix. Experimental results on image databases demonstrate the superiority of ICSN-TSL over existing methods in terms of recognition rate. The proposed ICSN-TSL achieves a recognition rate of up to 98% in normal cases. Notably, it also achieves a classification accuracy of over 87% even on artificially corrupted images.
更多查看译文
关键词
Least squares regression,inter-class sparsity,transition sub-space learning
AI 理解论文
溯源树
样例
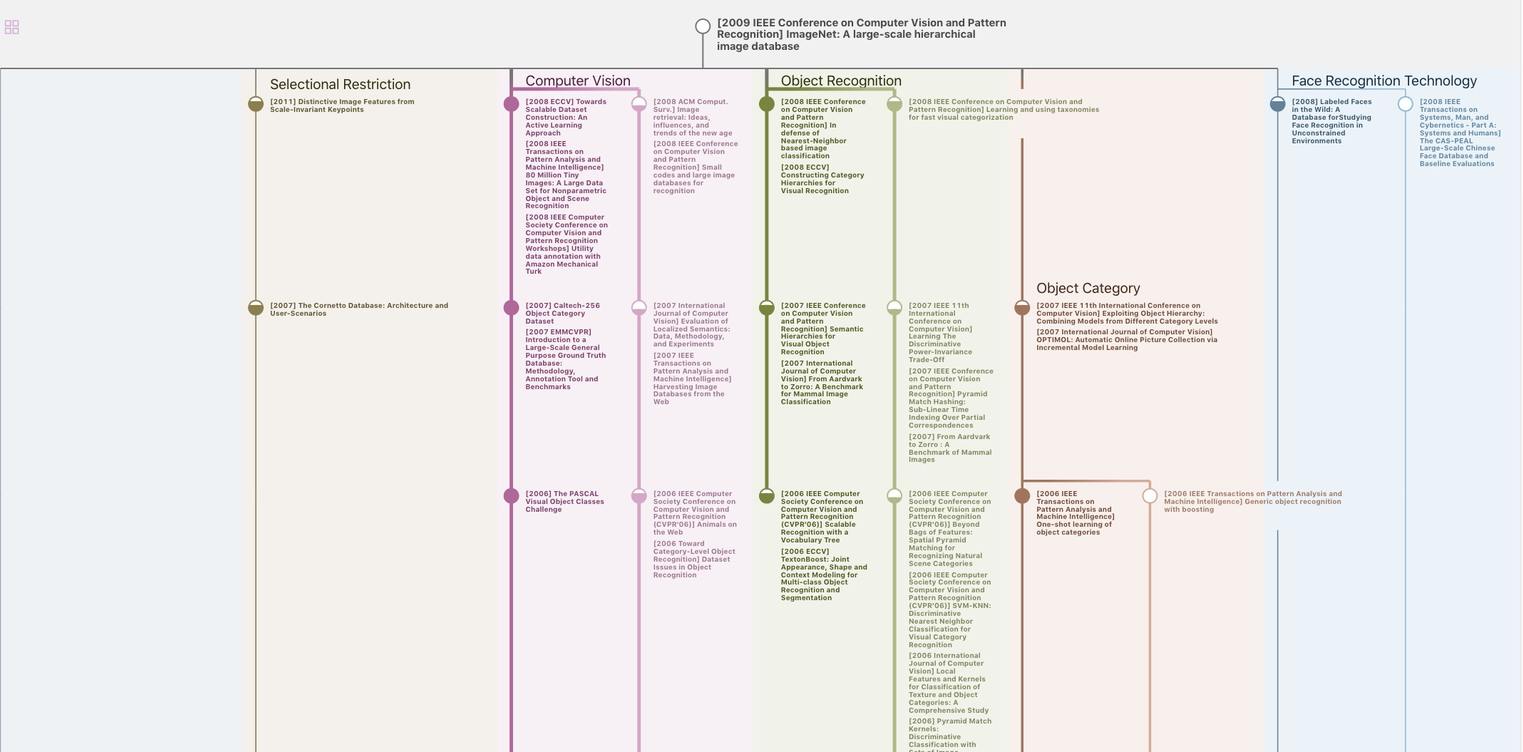
生成溯源树,研究论文发展脉络
Chat Paper
正在生成论文摘要