Causal Discovery via the Subsample Based Reward and Punishment Mechanism
PATTERN RECOGNITION AND COMPUTER VISION, PRCV 2023, PT III(2024)
摘要
The discovery of causal relationships between variables from a large number of nonlinear observations is an important research direction in the field of data mining. Extensive studies have highlighted the challenge of constructing accurate causal networks using existing algorithms using large-scale, nonlinear, and high-dimensional data. To address the challenge of this, we propose a general method for causal discovery algorithms applicable to handling large sample data, namely the subsample based reward and punishment mechanism (SRPM) method, which can handle nonlinear large sample data more effectively. We mainly made three contributions. First, we determine the size of the sample split based on experiments to obtain better learning results. Secondly, we developed a reward and punishment mechanism where each sub-sample dynamically changes its own weight in the process of learning the network skeleton, and proposed the SRPM, a subsample method based on the reward and punishment mechanism. Finally, we combine SRPM with three different additive noise model structure learning algorithms applicable to non-Gaussian nonlinear data respectively, and demonstrate the effectiveness of the method through experiments on data generated by a variety of nonlinear function dependencies. Compared with the existing algorithms, the causal network construction algorithm based on SRPM method has a great improvement in accuracy and time performance, and the effectiveness of the method is also verified in the real power plant data.
更多查看译文
关键词
causal discovery,nonlinear,high-dimensional,large sample
AI 理解论文
溯源树
样例
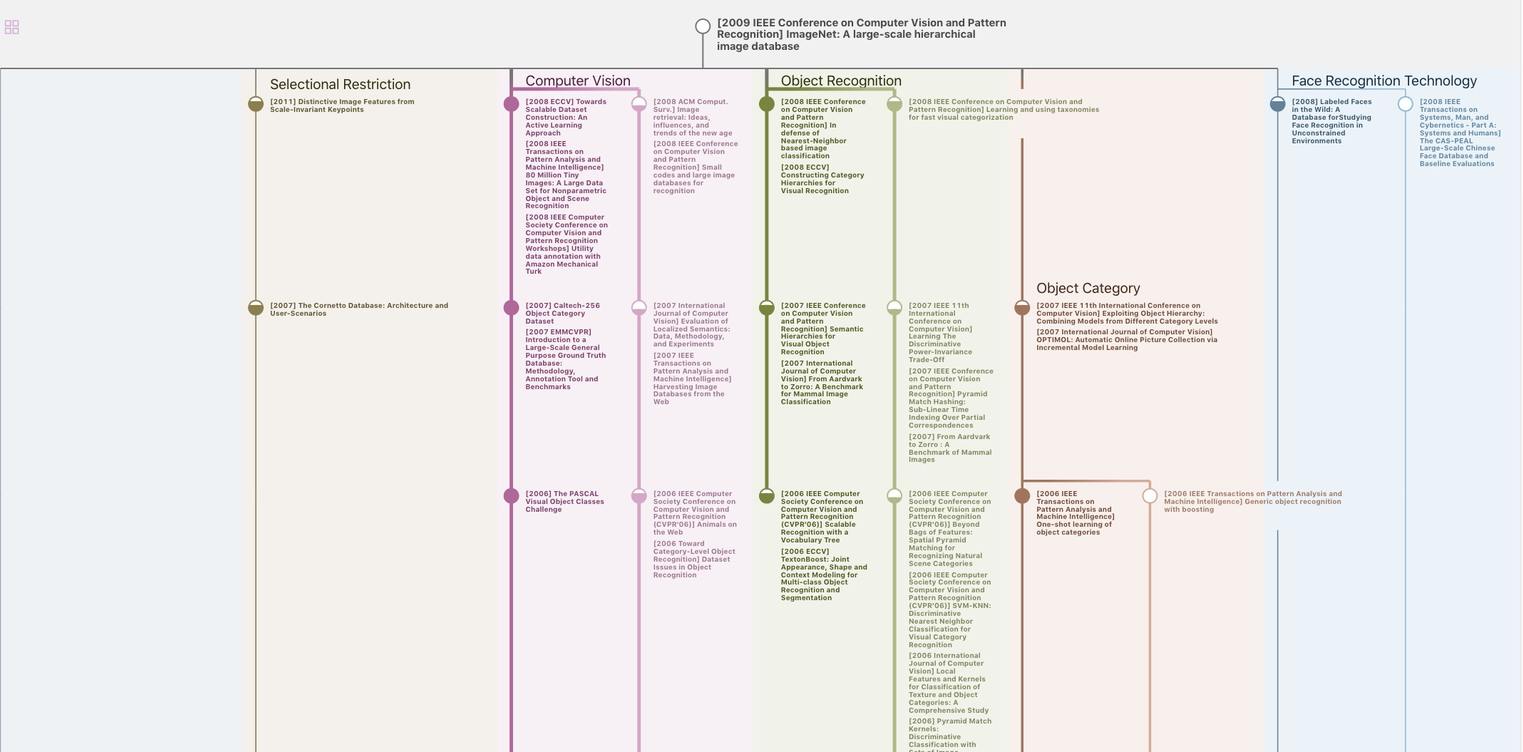
生成溯源树,研究论文发展脉络
Chat Paper
正在生成论文摘要