Urban flood extent segmentation and evaluation from real-world surveillance camera images using deep convolutional neural network
ENVIRONMENTAL MODELLING & SOFTWARE(2024)
摘要
This study explores the use of Deep Convolutional Neural Network (DCNN) for semantic segmentation of flood images. Imagery datasets of urban flooding were used to train two DCNN-based models, and camera images were used to test the application of the models with real-world data. Validation results show that both models extracted flood extent with a mean F1-score over 0.9. The factors that affected the performance included still water surface with specular reflection, wet road surface, and low illumination. In testing, reduced visibility during a storm and raindrops on surveillance cameras were major problems that affected the segmentation of flood extent. High-definition web cameras can be an alternative tool with the models trained on the data it collected. In conclusion, DCNN-based models can extract flood extent from camera images of urban flooding. The challenges with using these models on real-world data identified through this research present opportunities for future research.
更多查看译文
关键词
Deep learning,Deep convolutional neural network,Semantic segmentation,Flood extent
AI 理解论文
溯源树
样例
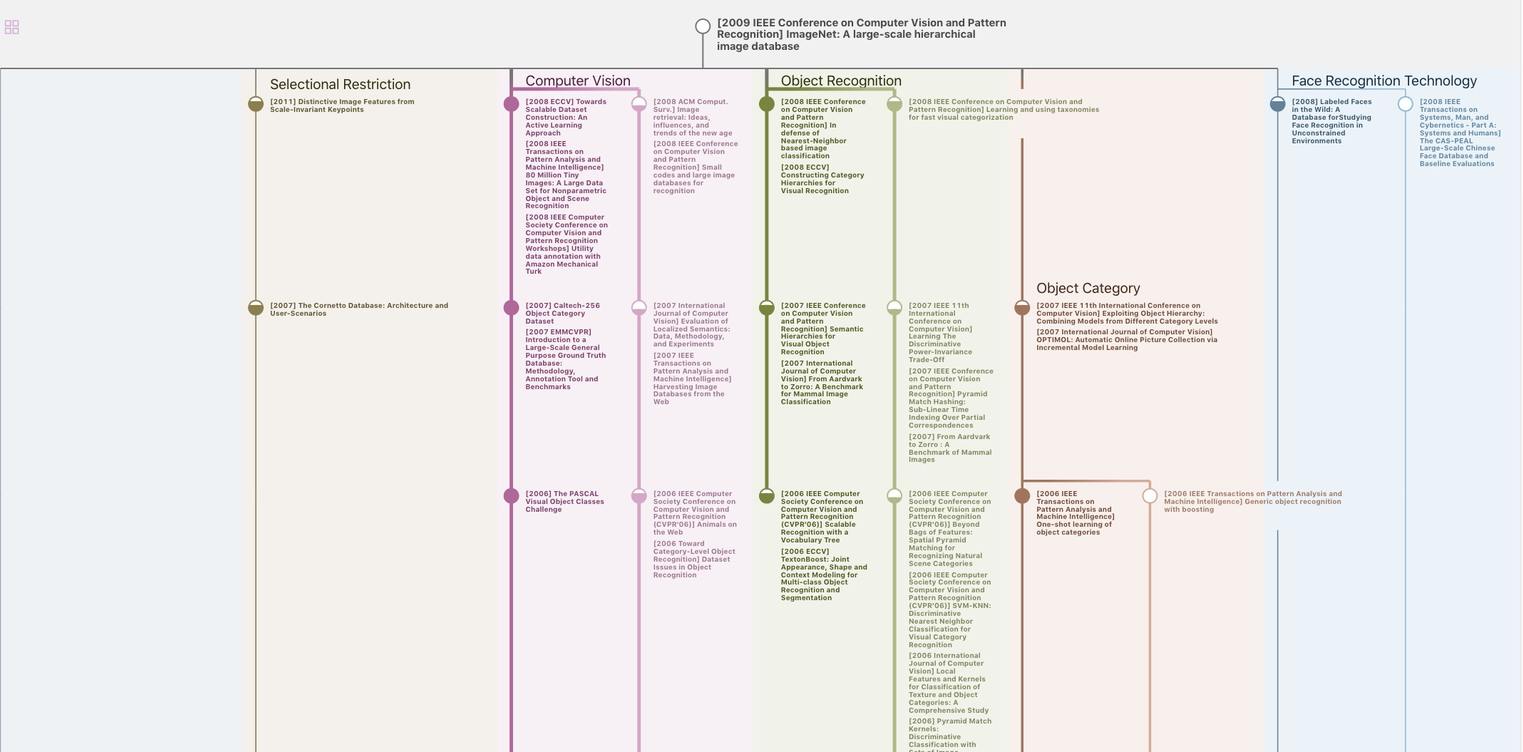
生成溯源树,研究论文发展脉络
Chat Paper
正在生成论文摘要