A Multi-group similarity-decoding-based method for deep model ensembling applied in the microcalcification classification on digital mammograms
BIOMEDICAL SIGNAL PROCESSING AND CONTROL(2024)
摘要
Classifying mammograms scored at Breast Imaging -Reporting and Data System (BI-RADS ) 4 is crucial for the early detection of breast cancer. But it is particularly challenging because microcalcifications (MCs) vary greatly in shape and distribution. Traditional machine learning and deep models are either time-consuming or poorly generalized. Recently, deep ensemble learning, which can improve generalization, has been developed rapidly. However, most ensembling strategies are based on weighted average and often sensitive to biased learners. To achieve data-adaption, they employ complex techniques to implement dynamic weight calculation. In this paper, we proposed a novel multi -group similarity -decoding -based method (MG-SDM) which enables a more robust and easier ensembling of different deep models. Firstly, the multi -group (MG) strategy is designed to transform model ensembling into a process of feature -level fusion, which could alleviate the impact of a single model on overall performance. Specifically, individual features of different models are grouped based on the position, and feature fusion is performed within each group. Secondly, the similarity -decoding -based method (SDM) is developed to fuse features. All individual features are vectorized through linear transformation. Then their similarities with a learnable latent embedding, which is introduced to denote most task -related features, are calculated using inner product. Finally, the similarity values among features within same group are normalized to be weights for a subsequent weighted summation operation. Extensive experiments on the clinical dataset demonstrate the superiority of the proposed over other state-of-the-art methods.
更多查看译文
关键词
Microcalcification classification,Data-adaption,Multi-group,Similarity decoding,Learnable latent embedding
AI 理解论文
溯源树
样例
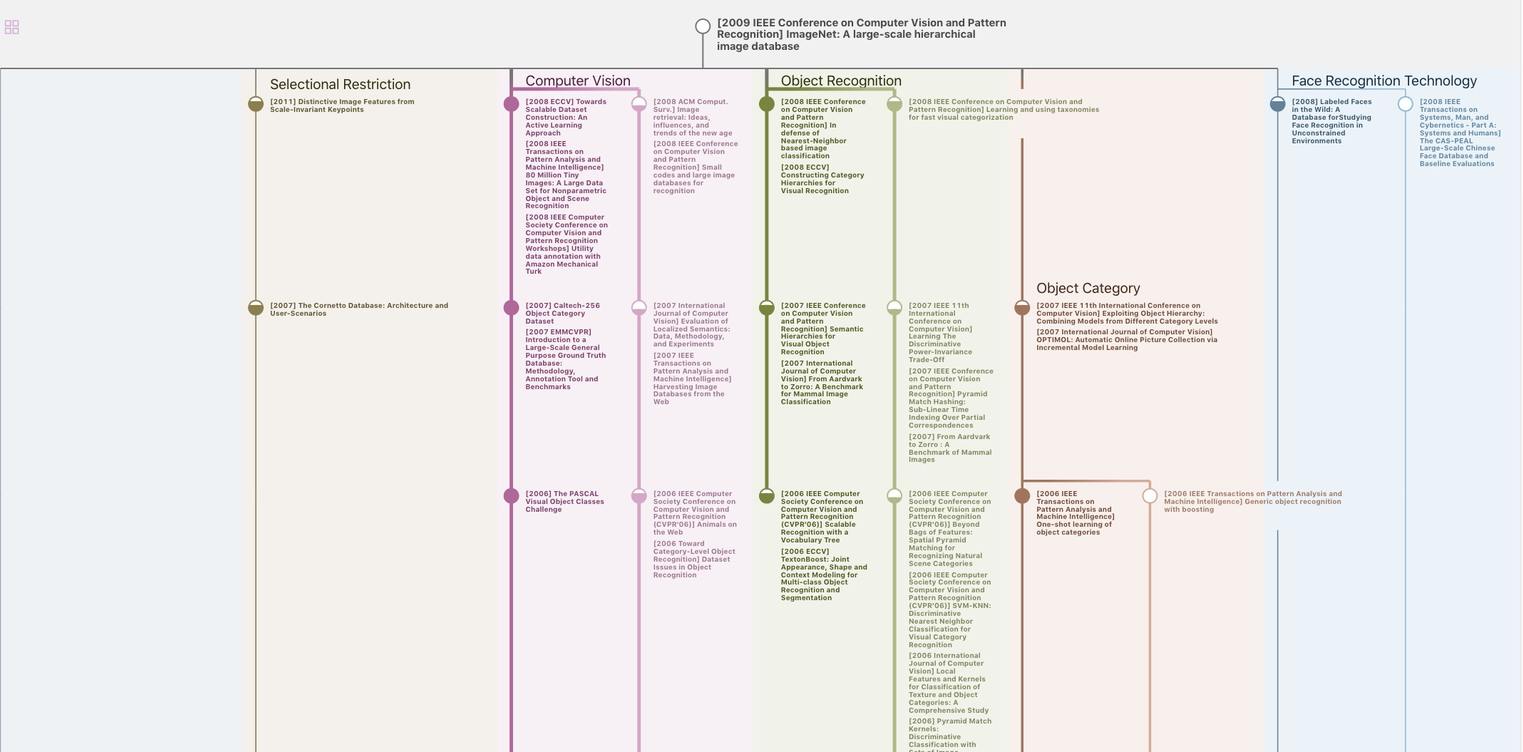
生成溯源树,研究论文发展脉络
Chat Paper
正在生成论文摘要