OctPCGC-Net: Learning Octree-Structured Context Entropy Model for Point Cloud Geometry Compression
PATTERN RECOGNITION AND COMPUTER VISION, PRCV 2023, PT II(2024)
摘要
In Point Cloud Geometry Compression (PCGC), an accurate context entropy model is necessary to reduce spatial redundancy. The octree-based auto-regressive context entropy model has great potential to explore large-scale context dependency. However, over-concentrated attention maps and instability of training process usually occur in large-scale context entropy models. To address these problems, we propose a novel OctPCGC-Net for PCGC based on deep learning framework. Specifically, we introduce a scaled cosine attention method in a large-scale context entropy model to alleviate the problem of over-concentrated attentionmaps caused by self-attentionmechanism, thereby improving the model's prediction accuracy. In order to improve the stability of model training, we further introduce a residual post normalization strategy to alleviate the phenomenon of accumulating activation scores as the network deepens, which makes the activation scores of different layers smoother and more stable. Experimental results show that comparedwith the state-of-the-art large-scale auto-regressive entropy models, our method saves 6.3%, 8.7%, and 6.3% bitrates in terms of Bjontegaard Delta Bit Rate (BDBR) on benchmark datasets SemanticKITTI, 8iVFB, and Owlii, respectively. Additionally, our method also achieves higher reconstruction quality (D1 PSNR) and smaller Chamfer distance (CD) under similar bits per point (BPP) on SemanticKITTI dataset.
更多查看译文
关键词
Point cloud geometry compression,Octree context,Entropy model,Scaled cosine attention
AI 理解论文
溯源树
样例
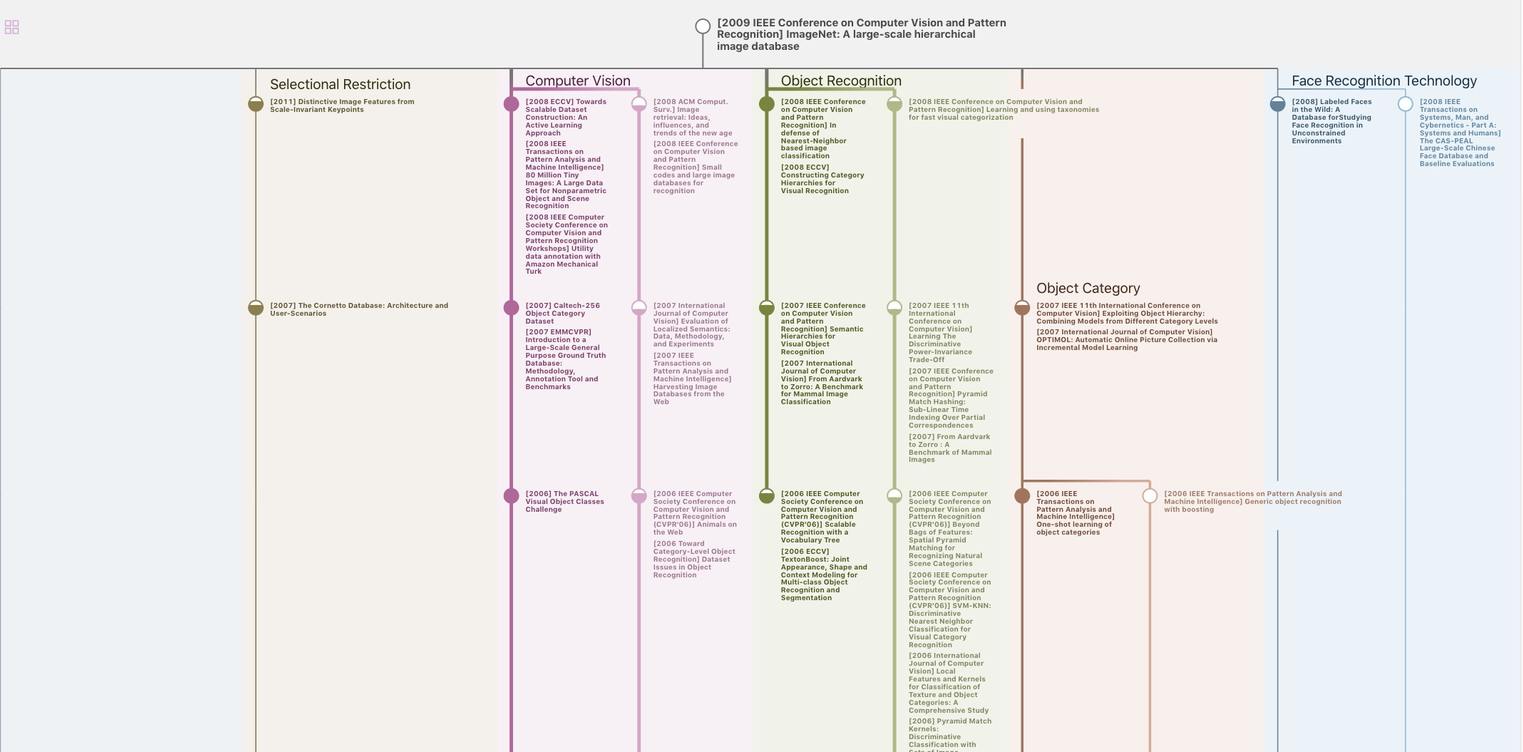
生成溯源树,研究论文发展脉络
Chat Paper
正在生成论文摘要