Generalizable Neural Radiance Field with Hierarchical Geometry Constraint
PATTERN RECOGNITION AND COMPUTER VISION, PRCV 2023, PT II(2024)
摘要
The emergence of the generalized neural radiance field models has greatly expanded the applicability of novel view synthesis task. However, existing methods primarily rely on 2D features obtained from neighboring views to assist the rendering, which often leads to degradation in unseen viewpoints. Some approaches introduce cost volumes in the model to provide geometric priors, but such coarse geometric information fails to constrain the model effectively, resulting in rendered images with abundant artifacts. In this work, we propose to provide the model with hierarchical geometry constraints to achieve better rendering results. We introduce cascaded MVSNet to provide hierarchical scene structural features for inferring the underlying scene geometry. It constrains the model's rendering under unseen viewpoints. Also, the hierarchical features provide fine representations that helps the reconstruction of details. Additionally, we utilize the hierarchical depth maps generated by cascade MVSNet to constrain the sampling process, ensuring that the sampled points are concentrated near the scene surface. Such sampling strategy filtering out numerous useless sampling points and improving both the sampling efficiency and rendering quality. Unlike previous generalized methods, we employ a new weight function proposed by Neus to eliminate inherent biases in the density field, disentangling the prediction of color and density with a signed distance field. Extensive experiments have demonstrated that our proposed method significantly improves the rendering quality and outperforms previous methods.
更多查看译文
关键词
Novel view synthesis,Neural radiance field,Generalization
AI 理解论文
溯源树
样例
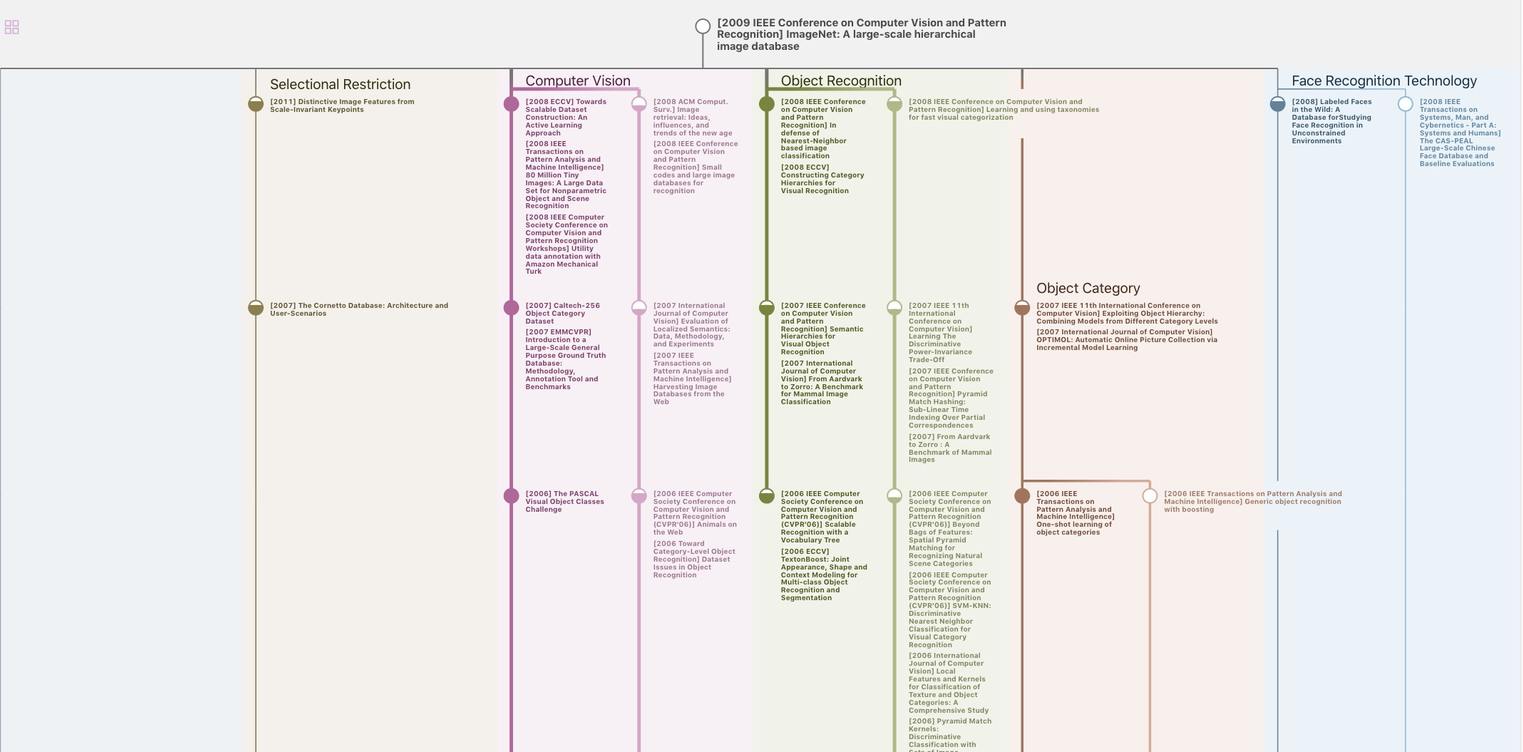
生成溯源树,研究论文发展脉络
Chat Paper
正在生成论文摘要