Contrastive Perturbation Network for Weakly Supervised Temporal Sentence Grounding
PATTERN RECOGNITION AND COMPUTER VISION, PRCV 2023, PT I(2024)
摘要
The purpose of temporal sentence grounding is to find the most relevant temporal period corresponding to the natural language query in an unmodified video. In recent years, the weak supervision paradigm, which does not require tedious annotations of starting and ending positions of the corresponding video segments, has gained significant attention due to its low annotation cost and reasonable efficiency. However, its effectiveness is seriously affected by the low-quality negative samples generated with random strategies. In this paper, we propose a Contrastive Perturbation Network (CPN), which introduces perturbation schemes into contrastive learning of weak supervised temporal sentence grounding. The perturbation involves both the proposal generation module and the reconstruction module of the CPN. In the proposal generation module, we introduce the KL divergence loss to minimize the distribution differences between the perturbed positive and real positive proposals, to force the network to be robust to the redundant information and learn fine-grained alignments between the text and video modalities. The reconstruction module leverages the perturbed features to generate a highly challenging negative proposal and strengthens the supervision to the proposal generation module by distinguishing the positive and negative proposals with the use of contrastive learning. Extensive experiments on two public benchmarks, i.e., ActivityNet Captions and Charades-STA, demonstrate that the proposed CPN could effectively improve the performance of weakly supervised temporal sentence grounding.
更多查看译文
关键词
Temporal Sentence grounding,Perturbation,Contrastive learning,Cross-modal analysis
AI 理解论文
溯源树
样例
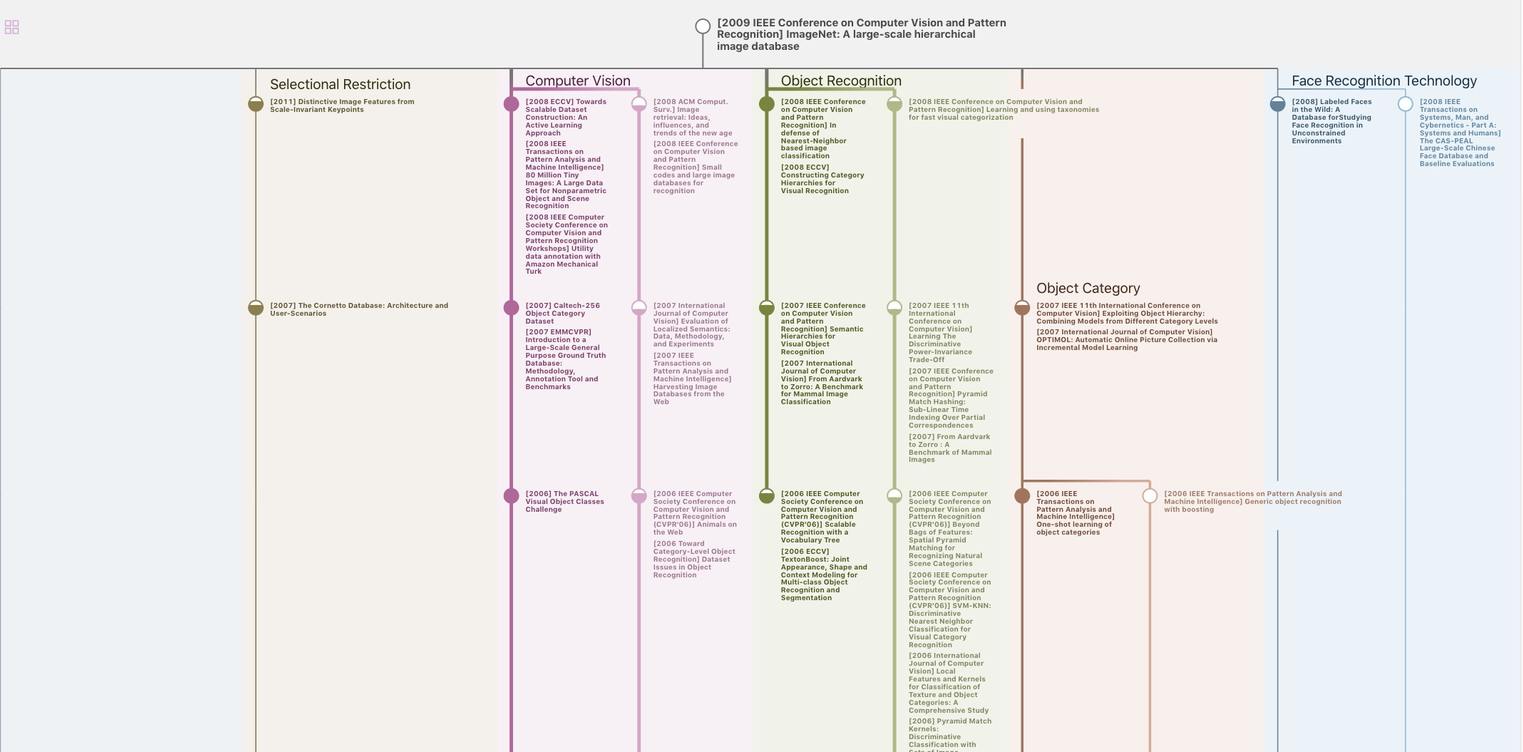
生成溯源树,研究论文发展脉络
Chat Paper
正在生成论文摘要