Modality Interference Decoupling and Representation Alignment for Caricature-Visual Face Recognition
PATTERN RECOGNITION AND COMPUTER VISION, PRCV 2023, PT I(2024)
摘要
Cross-modality face recognition aims to match facial images across different modalities. This task becomes very challenging when one of the modalities is the facial caricature, which enhances instinctive facial features through extreme distortions and exaggerations with diverse styles by artists. In this paper, we develop a novel modality interference decoupling and representation alignment (MIR) method for visual-caricature face recognition. Our MIR method consists of a backbone network, an identity-interference orthogonal decoupling (IIOD) module, and a modality feature alignment (MFA) module. The IIOD module adopts a three-branch structure to decouple the deep semantic features extracted by the backbone network into identity features and modality features. In IIOD, we design an identity subspace alignment (ISA) module to align the identity features from different branches. Moreover, we design the MFA module to perform feature alignment between the modality feature from the IIOD module and that from the pre-trained modality interference information encoder (MIE) via adversarial learning, extracting the modality-specific information. Based on the above designs, we can effectively alleviate the interference of modality differences and style differences, improving the final performance. Extensive experimental results on multiple datasets show that our proposed method outperforms several state-of-the-art cross-modality face recognition methods.
更多查看译文
关键词
Cross-modality face recognition,Feature decomposition,Representation Alignment
AI 理解论文
溯源树
样例
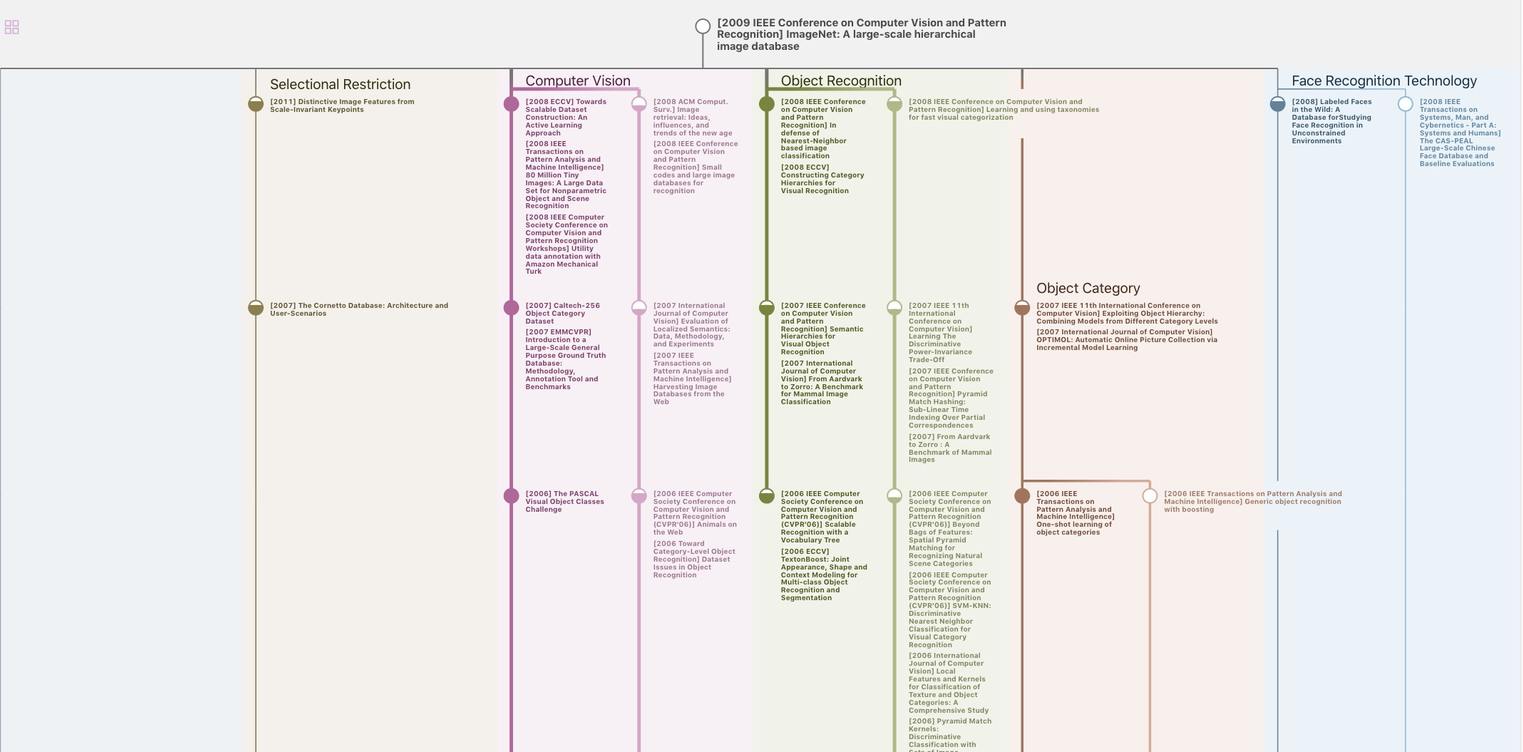
生成溯源树,研究论文发展脉络
Chat Paper
正在生成论文摘要