A Fuzzy Error Based Fine-Tune Method for Spatio-Temporal Recognition Model
PATTERN RECOGNITION AND COMPUTER VISION, PRCV 2023, PT I(2024)
摘要
The spatio-temporal convolution model is widely recognized for its effectiveness in predicting action in various fields. This model typically uses video clips as input and employs multiple clips for inference, ultimately deriving a video-level prediction through an aggregation function. However, the model will give a high confidence prediction result, regardless of whether the input clips have sufficient spatiotemporal information to indicate its class or not. The inaccurate high confidence prediction errors can subsequently affect the accuracy of the video-level results. Although the current approach to mitigating this problem involves increasing the number of clips used, it fails to address this problem from its root causes. To solve this issue, we propose a fine-tuning framework based on Fuzzy error loss, aimed at further refining the well-trained spatio-temporal convolution model that relies on dense sampling. By giving a low confidence prediction output for clips with insufficient spatio-temporal information, our framework strives to enhance the accuracy of video-level motion recognition. We conducted extensive experiments on two motion recognition datasets, namely UCF101 and Kinetics-Sounds, to evaluate the effectiveness of our proposed framework. The results indicate a significant improvement in motion recognition accuracy at the video level on both data sets.
更多查看译文
关键词
Action recognition,Fine-tuning method,Spatio-temporal convolution
AI 理解论文
溯源树
样例
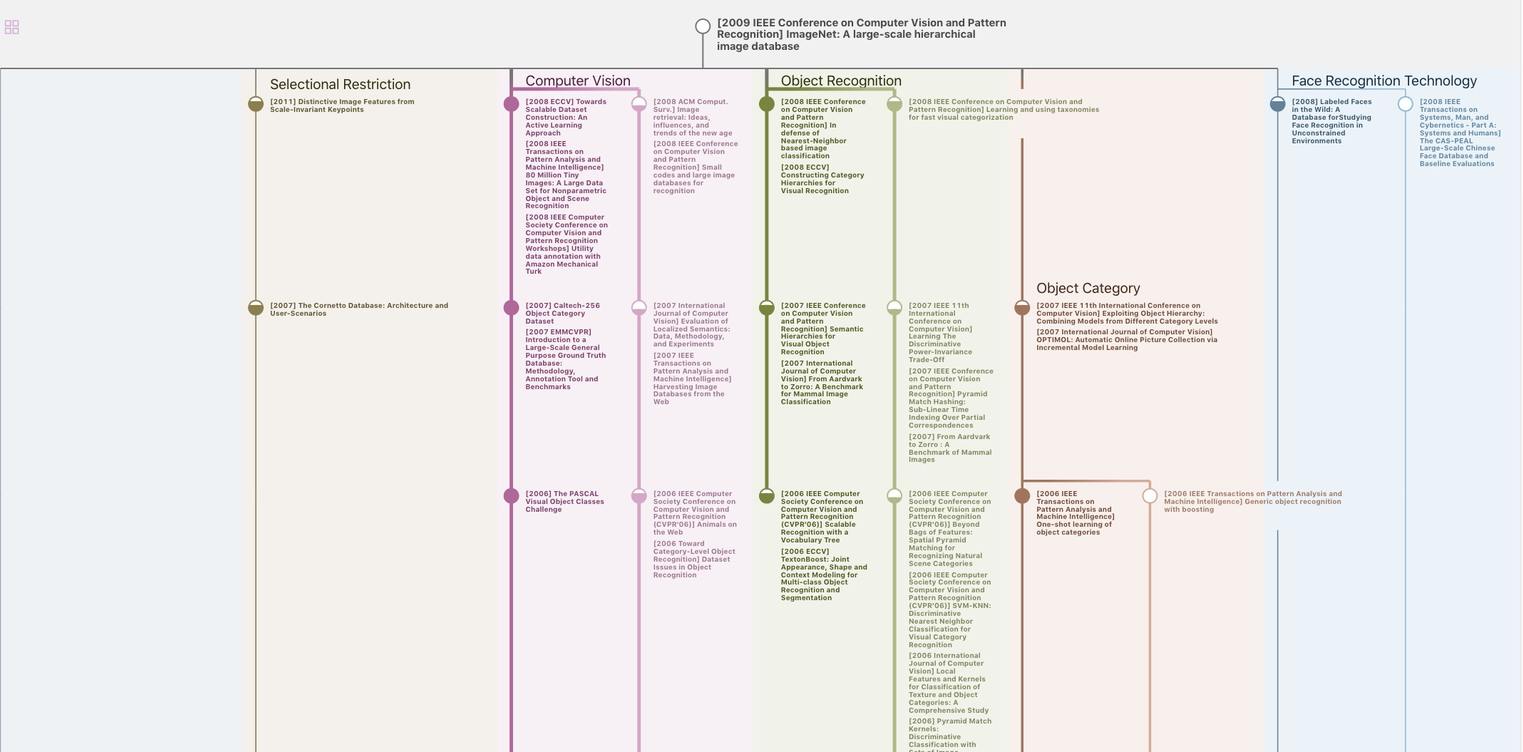
生成溯源树,研究论文发展脉络
Chat Paper
正在生成论文摘要