Dual Frequency Transformer for Efficient SDR-to-HDR Translation
Machine Intelligence Research(2024)
摘要
The SDR-to-HDR translation technique can convert the abundant standard-dynamic-range (SDR) media resources to high-dynamic-range (HDR) ones, which can represent high-contrast scenes, providing more realistic visual experiences. While recent vision Transformers have achieved promising performance in many low-level vision tasks, there are few works attempting to leverage Transformers for SDR-to-HDR translation. In this paper, we are among the first to investigate the performance of Transformers for SDR-to-HDR translation. We find that directly using the self-attention mechanism may involve artifacts in the results due to the inappropriate way to model long-range dependencies between the low-frequency and high-frequency components. Taking this into account, we advance the self-attention mechanism and present a dual frequency attention (DFA), which leverages the self-attention mechanism to separately encode the low-frequency structural information and high-frequency detail information. Based on the proposed DFA, we further design a multi-scale feature fusion network, named dual frequency Transformer (DFT), for efficient SDR-to-HDR translation. Extensive experiments on the HDRTV1K dataset demonstrate that our DFT can achieve better quantitative and qualitative performance than the recent state-of-the-art methods. The code of our DFT is made publicly available at https://github.com/CS-GangXu/DFT .
更多查看译文
关键词
Standard-dynamic-range to high-dynamic-range (SDR-to-HDR) translation,Transformer,dual frequency attention (DFA),frequency-aware feature decomposition,efficient model
AI 理解论文
溯源树
样例
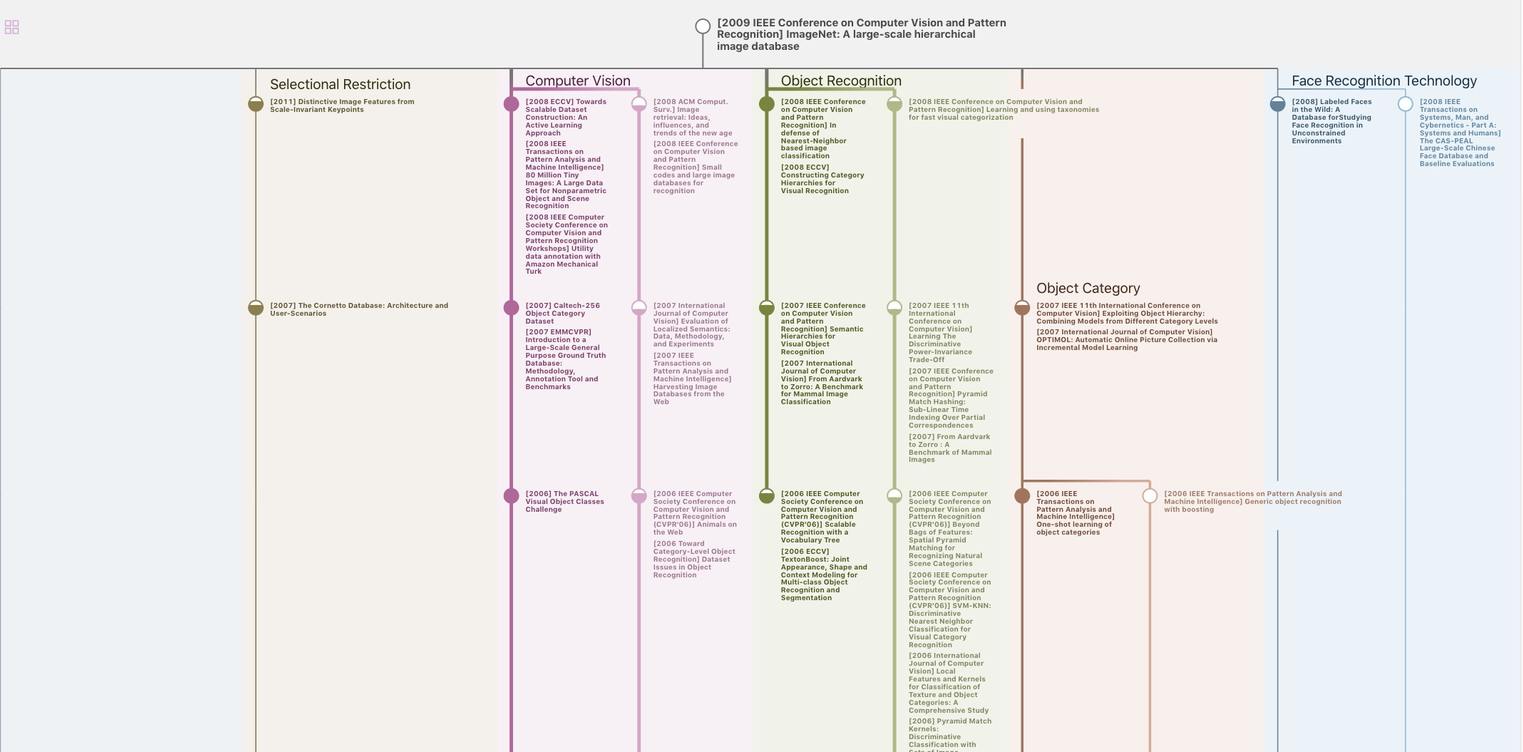
生成溯源树,研究论文发展脉络
Chat Paper
正在生成论文摘要