Treatment-Aware Hyperbolic Representation Learning for Causal Effect Estimation with Social Networks
CoRR(2024)
摘要
Estimating the individual treatment effect (ITE) from observational data is a crucial research topic that holds significant value across multiple domains. How to identify hidden confounders poses a key challenge in ITE estimation. Recent studies have incorporated the structural information of social networks to tackle this challenge, achieving notable advancements. However, these methods utilize graph neural networks to learn the representation of hidden confounders in Euclidean space, disregarding two critical issues: (1) the social networks often exhibit a scalefree structure, while Euclidean embeddings suffer from high distortion when used to embed such graphs, and (2) each ego-centric network within a social network manifests a treatment-related characteristic, implying significant patterns of hidden confounders. To address these issues, we propose a novel method called Treatment-Aware Hyperbolic Representation Learning (TAHyper). Firstly, TAHyper employs the hyperbolic space to encode the social networks, thereby effectively reducing the distortion of confounder representation caused by Euclidean embeddings. Secondly, we design a treatment-aware relationship identification module that enhances the representation of hidden confounders by identifying whether an individual and her neighbors receive the same treatment. Extensive experiments on two benchmark datasets are conducted to demonstrate the superiority of our method.
更多查看译文
AI 理解论文
溯源树
样例
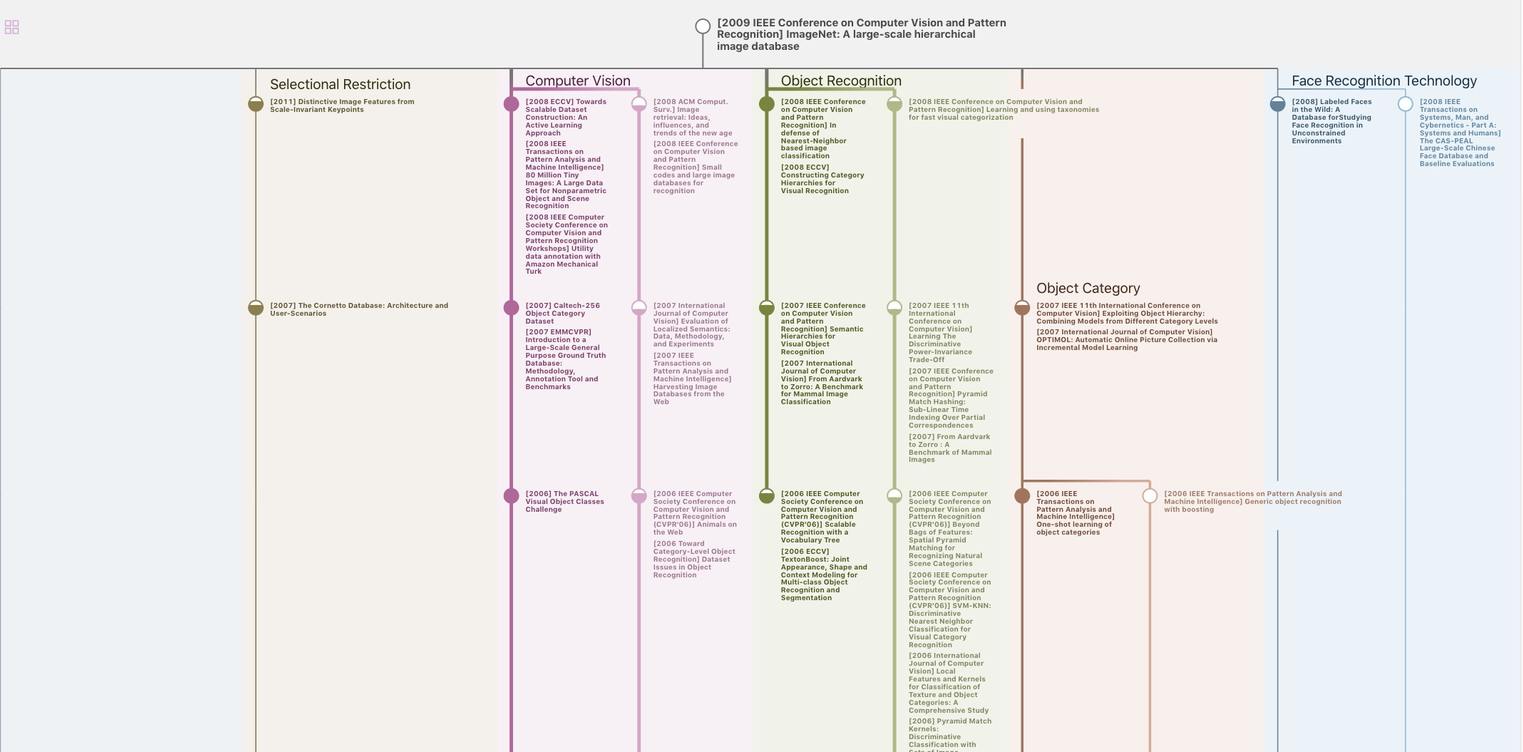
生成溯源树,研究论文发展脉络
Chat Paper
正在生成论文摘要