Hierarchical Augmentation and Distillation for Class Incremental Audio-Visual Video Recognition.
IEEE transactions on pattern analysis and machine intelligence(2024)
摘要
Audio-visual video recognition (AVVR) aims to integrate audio and visual clues to categorize videos accurately. While existing methods train AVVR models using provided datasets and achieve satisfactory results, they struggle to retain historical class knowledge when confronted with new classes in real-world situations. Currently, there are no dedicated methods for addressing this problem, so this paper concentrates on exploring Class Incremental Audio-Visual Video Recognition (CIAVVR). For CIAVVR, since both stored data and learned model of past classes contain historical knowledge, the core challenge is how to capture past data knowledge and past model knowledge to prevent catastrophic forgetting. As audio-visual data and model inherently contain hierarchical structures, i.e., model embodies low-level and high-level semantic information, and data comprises snippet-level, video-level, and distribution-level spatial information, it is essential to fully exploit the hierarchical data structure for data knowledge preservation and hierarchical model structure for model knowledge preservation. However, current image class incremental learning methods do not explicitly consider these hierarchical structures in model and data. Consequently, we introduce Hierarchical Augmentation and Distillation (HAD), which comprises the Hierarchical Augmentation Module (HAM) and Hierarchical Distillation Module (HDM) to efficiently utilize the hierarchical structure of data and models, respectively. Specifically, HAM implements a novel augmentation strategy, segmental feature augmentation, to preserve hierarchical model knowledge. Meanwhile, HDM introduces newly designed hierarchical (video-distribution) logical distillation and hierarchical (snippet-video) correlative distillation to capture and maintain the hierarchical intra-sample knowledge of each data and the hierarchical inter-sample knowledge between data, respectively. Evaluations on four benchmarks (AVE, AVK-100, AVK-200, and AVK-400) demonstrate that the proposed HAD effectively captures hierarchical information in both data and models, resulting in better preservation of historical class knowledge and improved performance. Furthermore, we provide a theoretical analysis to support the necessity of the segmental feature augmentation strategy.
更多查看译文
关键词
Audio-visual video recognition,class incremental learning,hierarchical augmentation and distillation
AI 理解论文
溯源树
样例
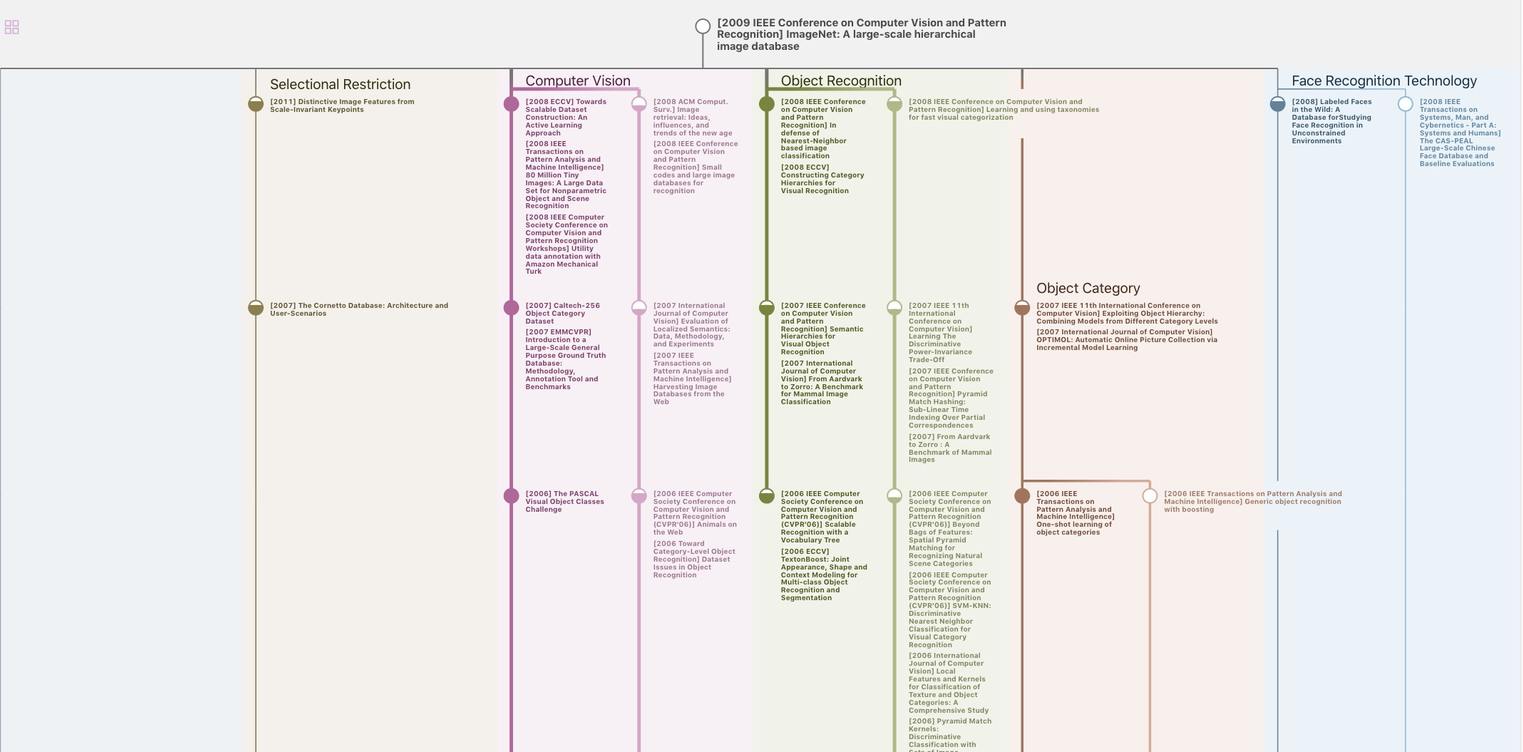
生成溯源树,研究论文发展脉络
Chat Paper
正在生成论文摘要