[Prediction of Spatial Distribution of Heavy Metals in Cultivated Soil Based on Multi-source Auxiliary Variables and Random Forest Model].
Environmental Science(2024)
摘要
Spatial prediction of the concentrations of soil heavy metals (HMs) in cultivated land is critical for monitoring cultivated land contamination and ensuring sustainable eco-agriculture. In this study, 32 environmental variables from terrain, climate, soil attributes, remote-sensing information, vegetation indices, and anthropogenic activities were used as auxiliary variables, and random forest (RF), regression Kriging (RK), ordinary Kriging (OK), and multiple linear regression (MLR) models were proposed to predict the concentrations of As, Cd, Cr, Cu, Hg, Ni, Pb, and Zn in cultivated soils. In comparison to those of RK, OK, and MLR, the RF model had the best prediction performance for As, Cd, Cr, Hg, Pb, and Zn, whereas the OK and RK models had highest prediction performance for Cu and Ni, respectively, showing that R2 was the highest, and mean absolute error (MAE) and root mean square error (RMSE) were the lowest. The prediction performance of the spatial distribution of soil HMs under different prediction methods was basically consistent. The high value areas of eight HMs concentrations were all distributed in the southern plain area. However, the RF model depicted the details of spatial prediction more prominently. Moreover, the importance ranking of influencing factors derived from the RF model indicated that the spatial variation in concentrations of the eight HMs in Lanxi City were mainly affected by the combined effects of Se, TN, pH, elevation, annual average temperature, annual average rainfall, distance from rivers, and distance from factories. Given the above, random forest models could be used as an effective method for the spatial prediction of soil heavy metals, providing scientific reference for regional soil pollution investigation, assessment, and management.
更多查看译文
关键词
soil heavy metals,environmental variables,random forest,spatial prediction,influencing factors
AI 理解论文
溯源树
样例
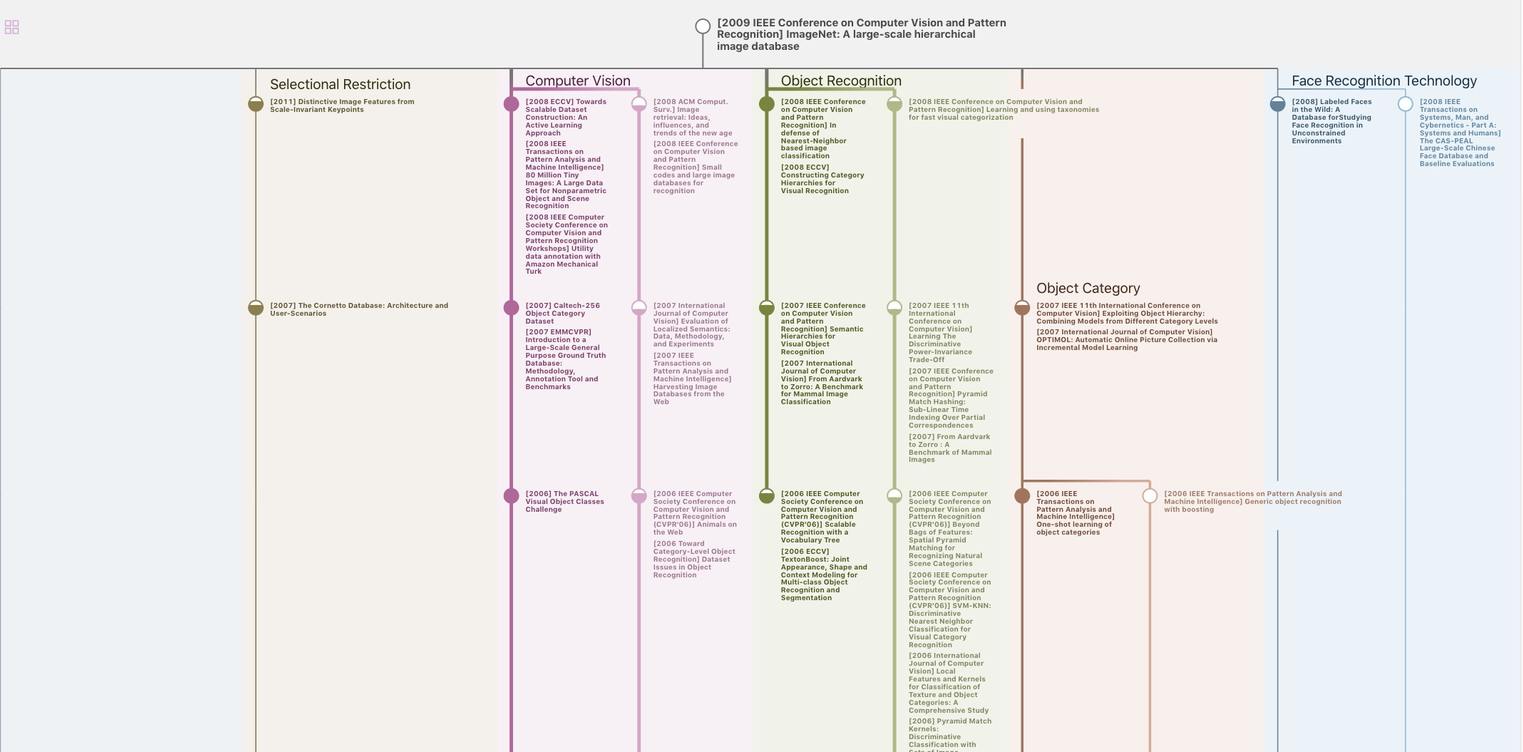
生成溯源树,研究论文发展脉络
Chat Paper
正在生成论文摘要