GRA: Graph Representation Alignment for Semi-Supervised Action Recognition
IEEE TRANSACTIONS ON NEURAL NETWORKS AND LEARNING SYSTEMS(2024)
摘要
Graph convolutional networks (GCNs) have emerged as a powerful tool for action recognition, leveraging skeletal graphs to encapsulate human motion. Despite their efficacy, a significant challenge remains the dependency on huge labeled datasets. Acquiring such datasets is often prohibitive, and the frequent occurrence of incomplete skeleton data, typified by absent joints and frames, complicates the testing phase. To tackle these issues, we present graph representation alignment (GRA), a novel approach with two main contributions: 1) a self-training (ST) paradigm that substantially reduces the need for labeled data by generating high-quality pseudo-labels, ensuring model stability even with minimal labeled inputs and 2) a representation alignment (RA) technique that utilizes consistency regularization to effectively reduce the impact of missing data components. Our extensive evaluations on the NTU RGB+D and Northwestern-UCLA (N-UCLA) benchmarks demonstrate that GRA not only improves GCN performance in data-constrained environments but also retains impressive performance in the face of data incompleteness.
更多查看译文
关键词
Action recognition,consistency regularization,graph convolutional networks (GCNs),graph representation learning,self-training (ST),semi-supervised learning,skeleton action recognition
AI 理解论文
溯源树
样例
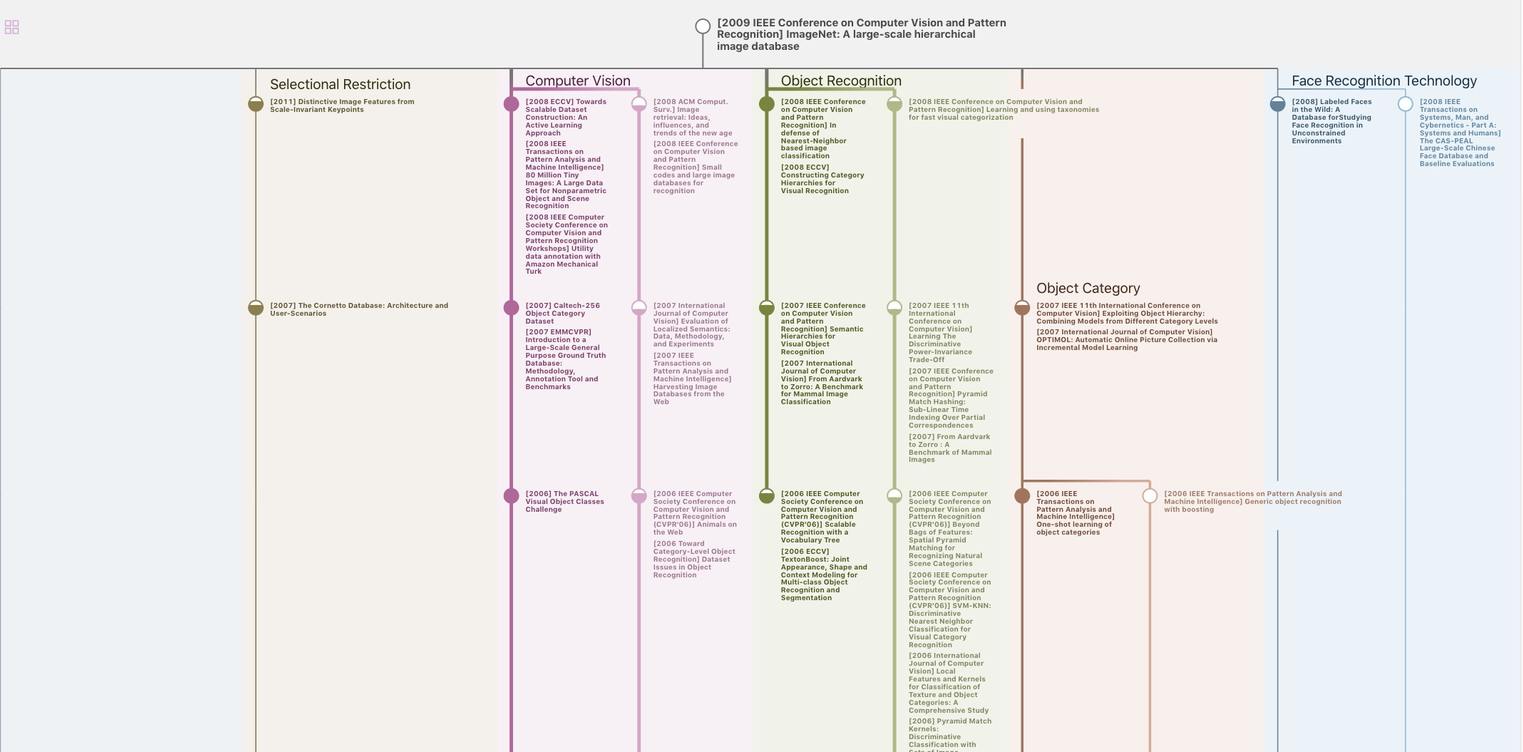
生成溯源树,研究论文发展脉络
Chat Paper
正在生成论文摘要