Toward Balance Deep Semisupervised Clustering
IEEE TRANSACTIONS ON NEURAL NETWORKS AND LEARNING SYSTEMS(2024)
摘要
The goal of balanced clustering is partitioning data into distinct groups of equal size. Previous studies have attempted to address this problem by designing balanced regularizers or utilizing conventional clustering methods. However, these methods often rely solely on classic methods, which limits their performance and primarily focuses on low-dimensional data. Although neural networks exhibit effective performance on high-dimensional datasets, they struggle to effectively leverage prior knowledge for clustering with a balanced tendency. To overcome the above limitations, we propose deep semisupervised balanced clustering, which simultaneously learns clustering and generates balance-favorable representations. Our model is based on the autoencoder paradigm incorporating a semisupervised module. Specifically, we introduce a balance-oriented clustering loss and incorporate pairwise constraints into the penalty term as a pluggable module using the Lagrangian multiplier method. Theoretically, we ensure that the proposed model maintains a balanced orientation and provides a comprehensive optimization process. Empirically, we conducted extensive experiments on four datasets to demonstrate significant improvements in clustering performance and balanced measurements. Our code is available at https://github.com/DuannYu/BalancedSemi-TNNLS.
更多查看译文
关键词
Clustering methods,Feature extraction,Task analysis,Wireless sensor networks,Distributed databases,Data models,Training,Balanced clustering,deep clustering,Lagrangian multipliers,pairwise information
AI 理解论文
溯源树
样例
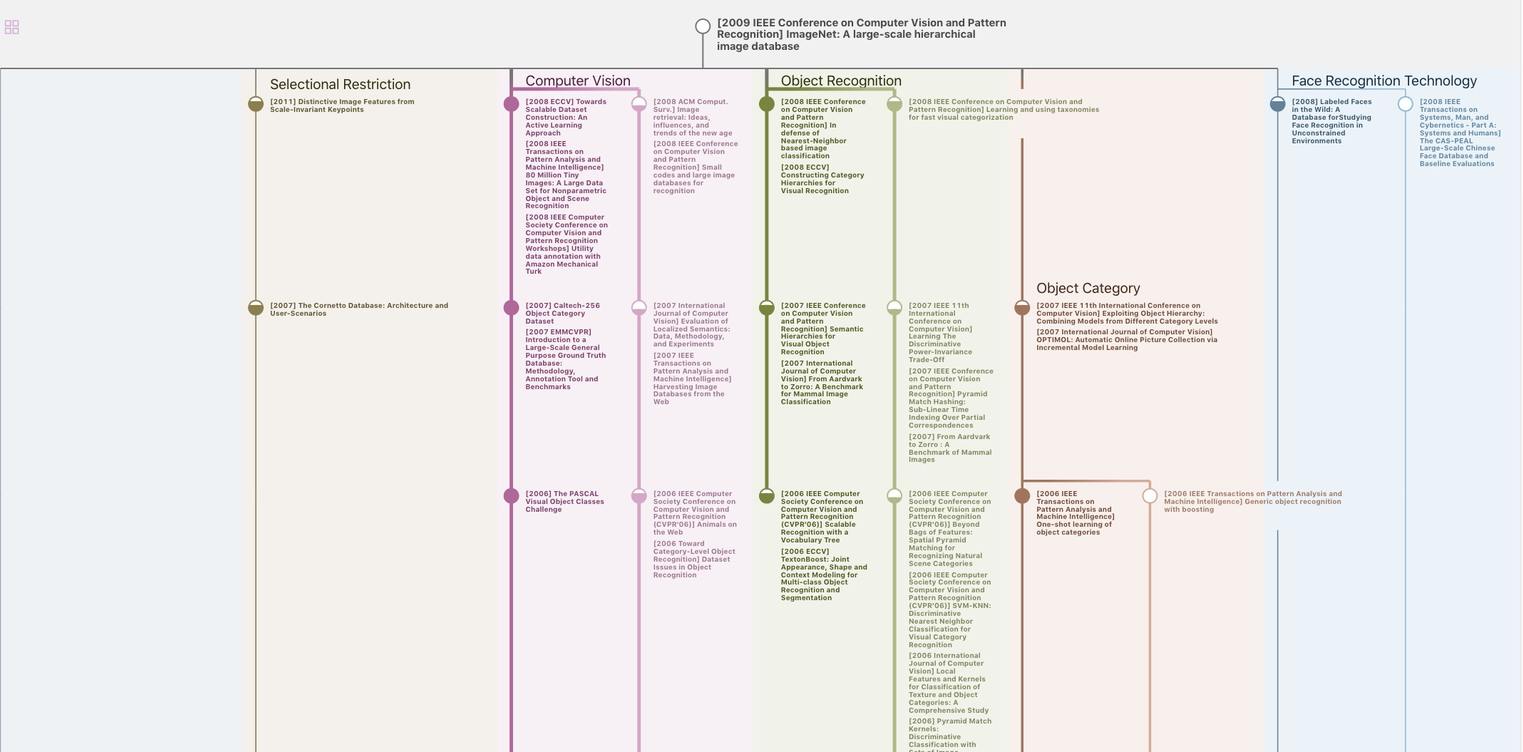
生成溯源树,研究论文发展脉络
Chat Paper
正在生成论文摘要