Development and Validation of a Machine Learning Model to Predict Weekly Risk of Hypoglycemia in Patients with Type 1 Diabetes Based on Continuous Glucose Monitoring.
Diabetes technology & therapeutics(2024)
摘要
AIM:The aim of this study was to develop and validate a prediction model based on CGM data to identify a week-to-week risk profile of excessive hypoglycemia.
METHODS:We analyzed, trained, and internally tested two prediction models using CGM data from 205 type 1 diabetes patients with long-term CGM monitoring. A binary classification approach (XGBoost) combined with feature engineering deployed on the CGM signals was utilized to predict excessive hypoglycemia risk defined by two targets (TBR > 4% and the upper TBR 90th percentile limit) of time below range (TBR) the following week. The models were validated in two independent cohorts with a total of 253 additional patients.
RESULTS:A total of 61,470 weeks of CGM data were included in the analysis. The XGBoost models had a ROC-AUC of 0.83-0.87 (95% confidence interval [CI]; 0.83-0.88) in the test dataset. The external validation showed ROC-AUCs of 0.81-0.90. The most discriminative features included the low blood glucose index (LBGI), the glycemic risk assessment diabetes equation (GRADE), hypoglycemia, the TBR, waveform length, the CV and mean glucose during the previous week. This highlights that the pattern of hypoglycemia combined with glucose variability during the past week contains information on the risk of future hypoglycemia.
CONCLUSION:Prediction models based on real-world CGM data can be used to predict the risk of hypoglycemia in the forthcoming week. The models showed good performance in both the internal and external validation cohorts.
更多查看译文
AI 理解论文
溯源树
样例
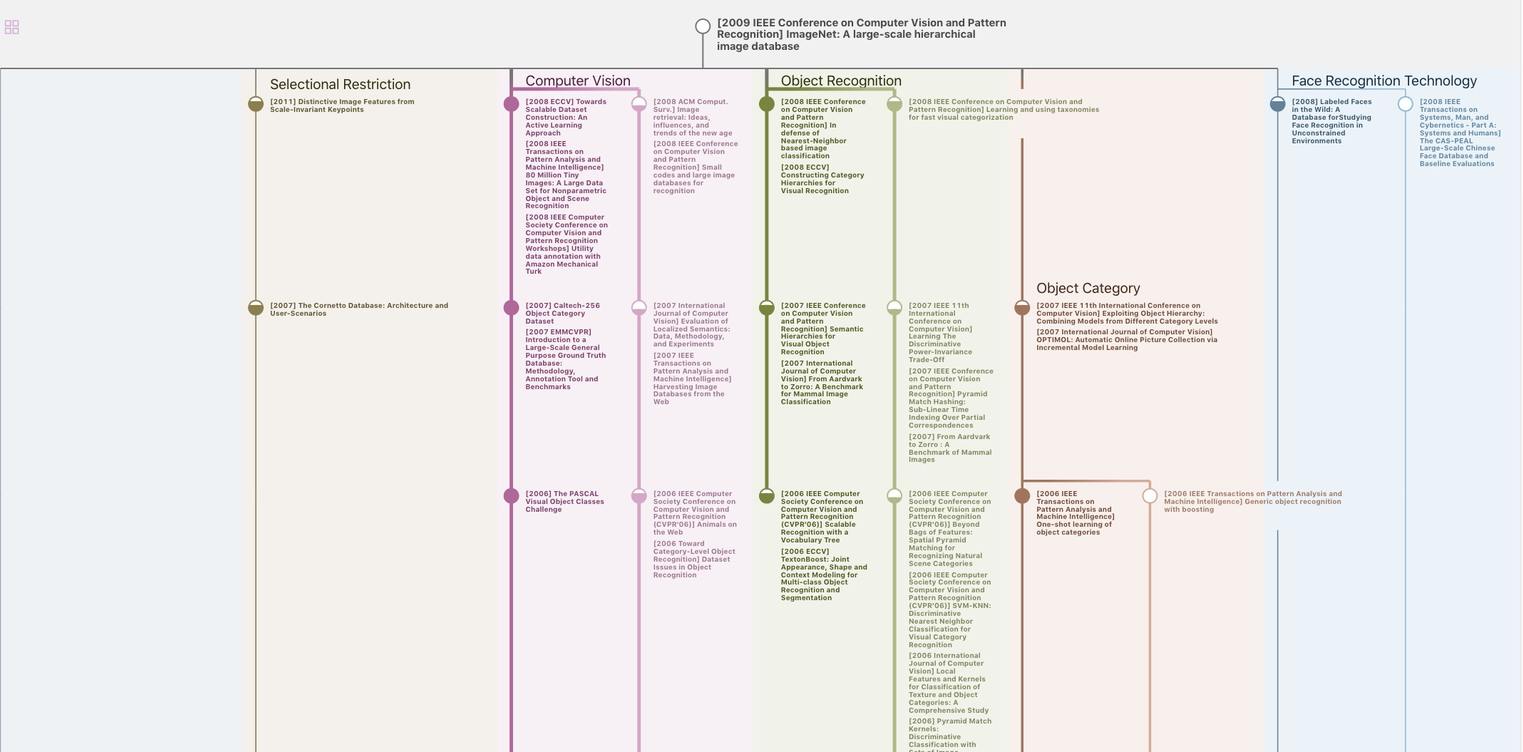
生成溯源树,研究论文发展脉络
Chat Paper
正在生成论文摘要