Neural Network-Assisted Humanization of COVID-19 Hamster scRNAseq Data Reveals Matching Severity States in Human Disease
biorxiv(2024)
摘要
Translating findings from animal models to human disease is essential for dissecting disease mechanisms, developing and testing precise therapeutic strategies. The coronavirus disease 2019 (COVID-19) pandemic has highlighted this need, particularly for models showing disease severity-dependent immune responses. Single-cell transcriptomics (scRNAseq) is well poised to reveal similarities and differences between species at the molecular and cellular level with unprecedented resolution. However, computational methods enabling detailed matching are still scarce. Here, we provide a structured scRNAseq-based approach that we applied to scRNAseq from blood leukocytes originating from humans and hamsters affected with moderate or severe COVID-19. Integration of COVID-19 patient data with two hamster models that develop moderate (Syrian hamster, Mesocricetus auratus) or severe (Roborovski hamster, Phodopus roborovskii) disease revealed that most cellular states are shared across species. A neural network-based analysis using variational autoencoders quantified the overall transcriptomic similarity across species and severity levels, showing highest similarity between neutrophils of Roborovski hamsters and severe COVID-19 patients, while Syrian hamsters better matched patients with moderate disease, particularly in classical monocytes. We further used transcriptome-wide differential expression analysis to identify which disease stages and cell types display strongest transcriptional changes. Consistently, hamster's response to COVID-19 was most similar to humans in monocytes and neutrophils. Disease-linked pathways found in all species specifically related to interferon response or inhibition of viral replication. Analysis of candidate genes and signatures supported the results. Our structured neural network-supported workflow could be applied to other diseases, allowing better identification of suitable animal models with similar pathomechanisms across species.
### Competing Interest Statement
MS received funding from Pfizer Inc. for a project related to pneumococcal vaccination. MS receives funding from Owkin for a project not related to this research. MW reports grants and personal fees from Biotest, grants and personal fees from Pantherna, grants and personal fees from Vaxxilon, personal fees from Aptarion, personal fees from Astra Zeneca, personal fees from Chiesi, personal fees from Insmed, personal fees from Gilead, outside the submitted work. GN reports grants from Biotest AG outside the submitted work. The other authors declare no competing interest.
更多查看译文
AI 理解论文
溯源树
样例
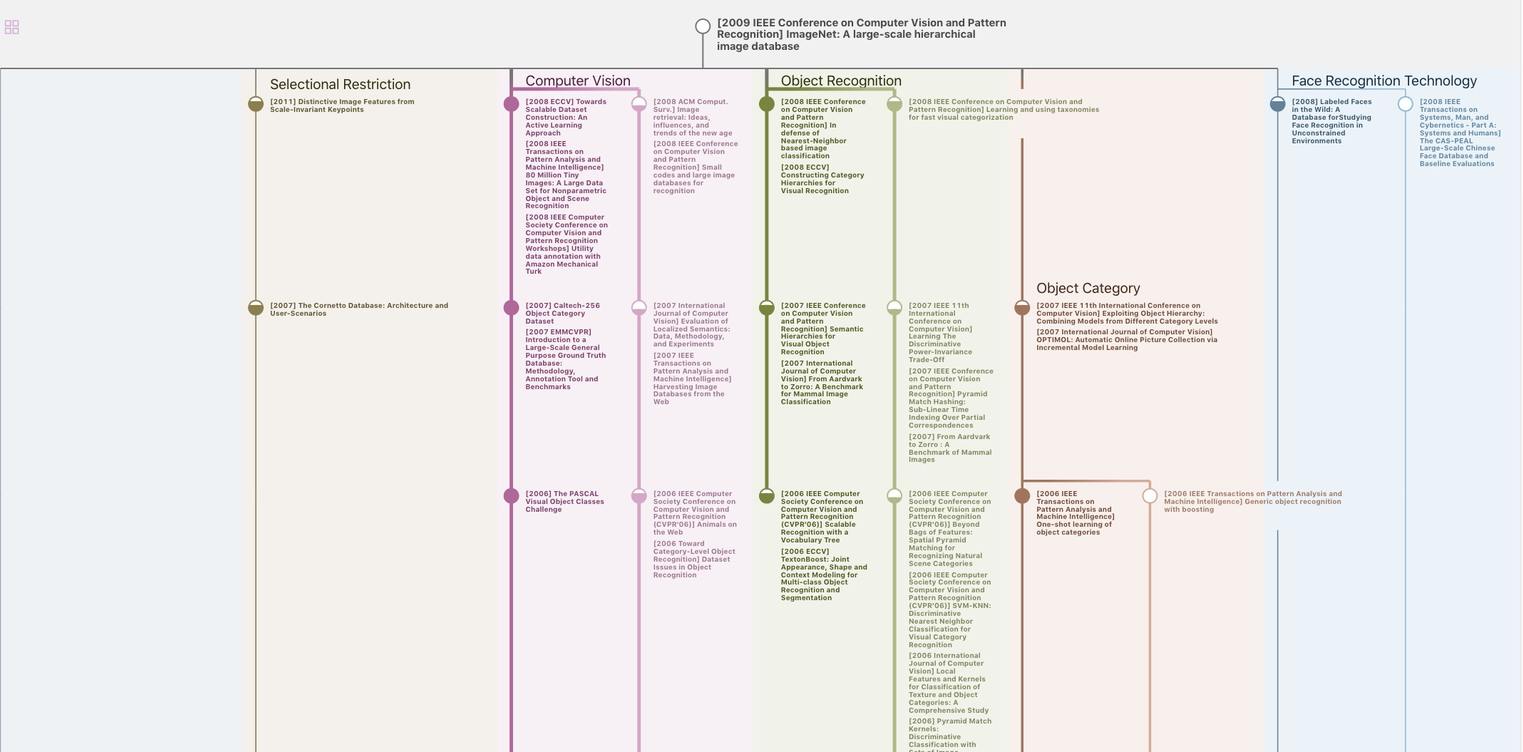
生成溯源树,研究论文发展脉络
Chat Paper
正在生成论文摘要