Improved Early Detection of Drug-Induced Liver Injury by Integrating Predicted in vivo and in vitro Data
biorxiv(2024)
摘要
Drug-induced liver injury (DILI) presents a significant challenge in drug discovery, often leading to clinical trial failures and necessitating drug withdrawals. In this study, we introduce a novel method for DILI prediction that first predicts eleven proxy-DILI labels and then uses them as features in addition to chemical structural features to predict DILI. The features include in vitro (e.g., mitochondrial toxicity, bile salt export pump inhibition) data, in vivo (e.g., preclinical rat hepatotoxicity studies) data, pharmacokinetic parameters of maximum concentration, structural fingerprints, and physicochemical parameters. We trained DILI-prediction models on 1020 compounds from the DILIst dataset and tested on a held-out external test set of 255 compounds from DILIst dataset. The best model, DILIPredictor, attained a balanced accuracy of 70% and an LR+ score of 7.21. This model enabled the early detection of 26 toxic compounds compared to models using only structural features (4.62 LR+ score). Using feature interpretation from DILIPredictor, we were able to identify the chemical substructures causing DILI as well as differentiate cases DILI is caused by compounds in animals but not in humans. For example, DILIPredictor correctly recognised 2-butoxyethanol as non-toxic in humans despite its hepatotoxicity in mice models. Overall, the DILIPredictor model improves the detection of compounds causing DILI with an improved differentiation between animal and human sensitivity as well as potential for mechanism evaluation. DILIPredictor is publicly available at https://broad.io/DILIPredictor for use via web interface and with all code available for download.
### Competing Interest Statement
The authors have declared no competing interest.
更多查看译文
AI 理解论文
溯源树
样例
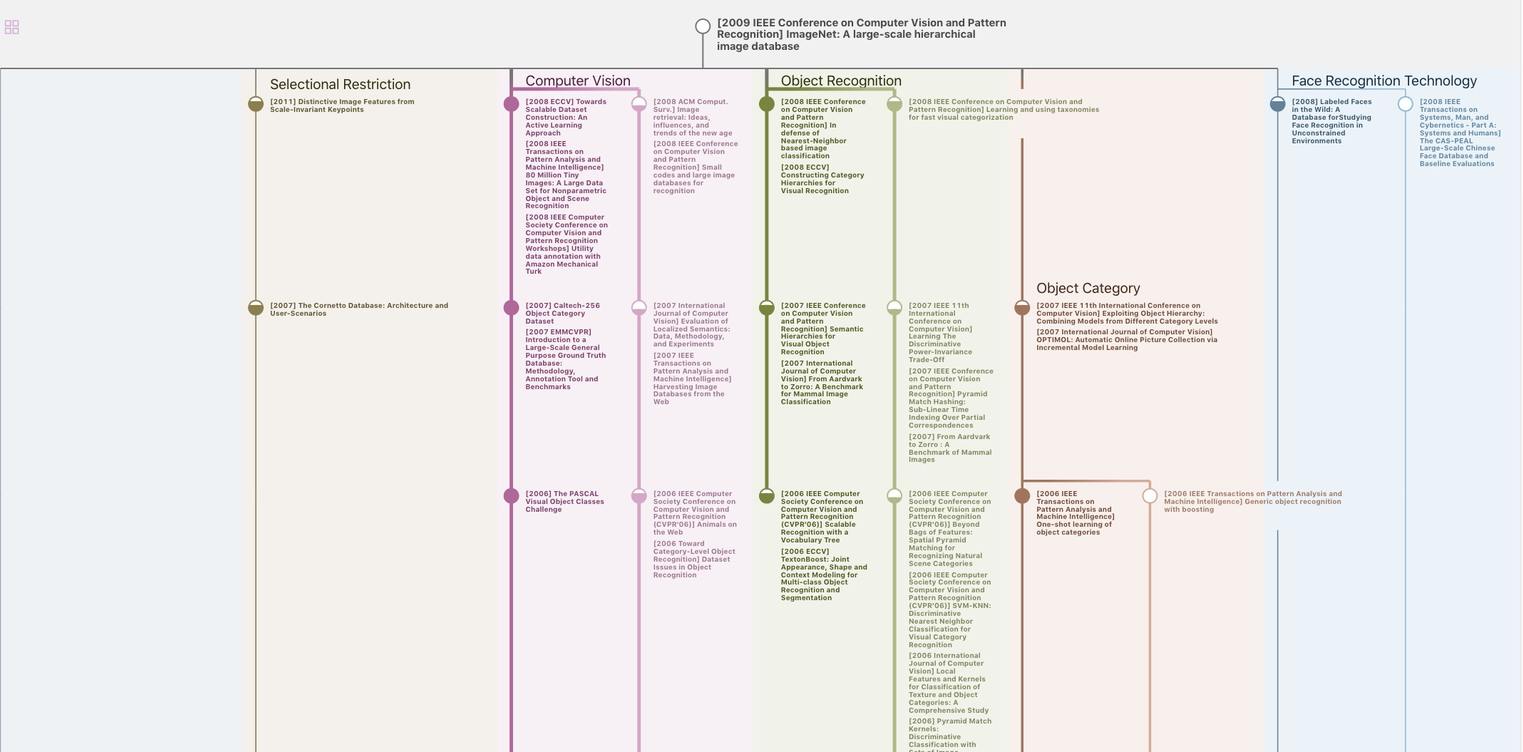
生成溯源树,研究论文发展脉络
Chat Paper
正在生成论文摘要