A hierarchical transformer-based adaptive metric and joint-learning network for few-shot rolling bearing fault diagnosis
MEASUREMENT SCIENCE AND TECHNOLOGY(2024)
摘要
Recently, deep learning techniques have significantly bolstered the advancement of intelligent fault diagnosis. However, in engineering practice, the limited availability of fault samples poses considerable challenges to the existing methods. To address this problem, a hierarchical Transformer-based adaptive metric and joint-learning network (HTAMJN) is suggested in this paper. Firstly, a hierarchical progressive fusion encoder based on orthogonal self-attention is devised, which effectively enhances the model's attention to local features and establishes comprehensive interdependencies. To reduce the demand for labeled data, a metric method with a coupling mechanism is proposed to adaptively enhance the metric benchmark, making the category representation more discriminative. Additionally, a joint optimization scheme based on the episodic paradigm is formulated to improve the recognition capability of the network for different categories under few-shot scenarios. The outcomes demonstrate that the HTAMJN outperforms several comparison models in both few-shot diagnosis and cross-domain recognition tasks in experiments conducted on three bearing datasets, exhibiting superior performance.
更多查看译文
关键词
rolling bearings,intelligent fault diagnosis,few-shot learning,self-attention,metric learning
AI 理解论文
溯源树
样例
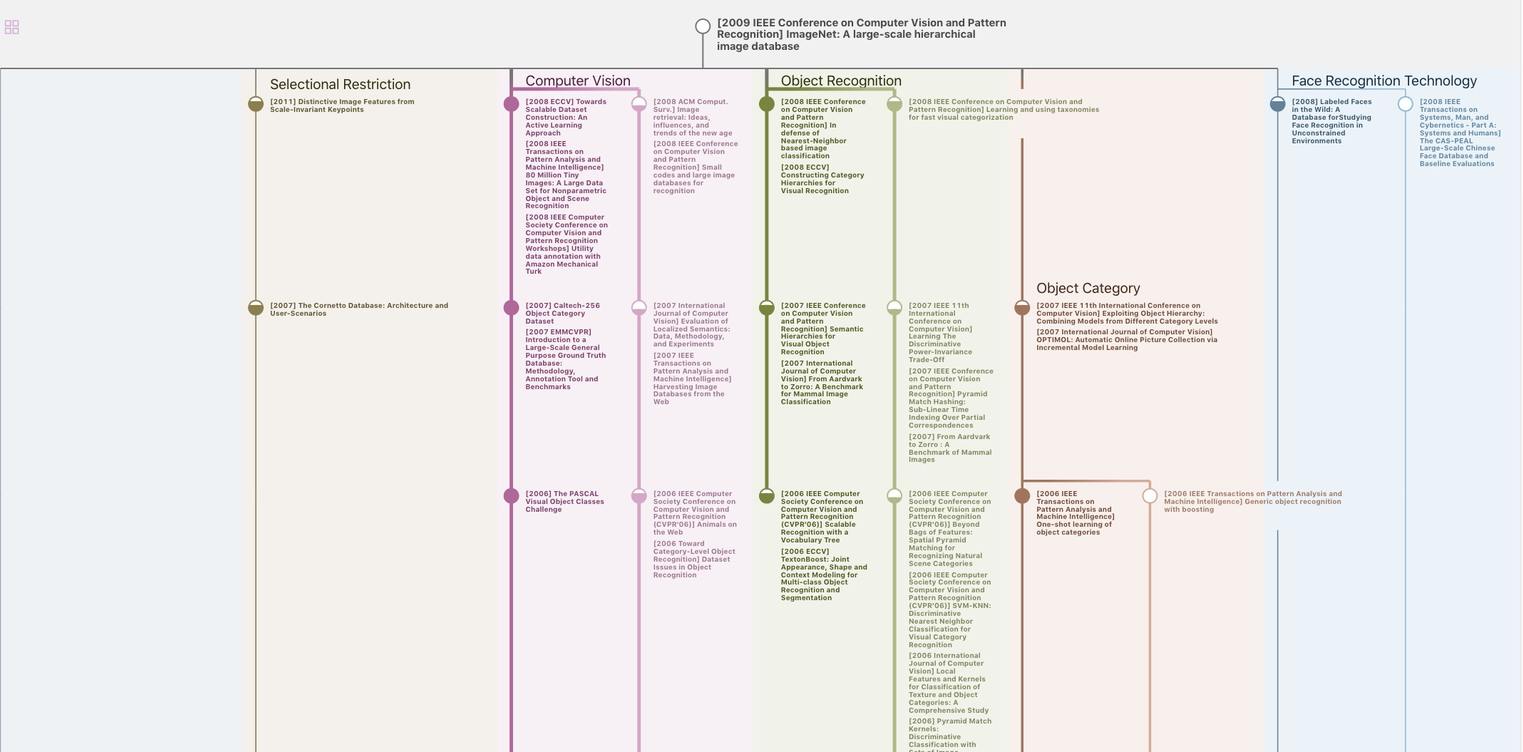
生成溯源树,研究论文发展脉络
Chat Paper
正在生成论文摘要