A reinforcement learning-based routing algorithm for large street networks
INTERNATIONAL JOURNAL OF GEOGRAPHICAL INFORMATION SCIENCE(2024)
摘要
Evacuation planning and emergency routing systems are crucial in saving lives during disasters. Traditional emergency routing systems, despite their best efforts, often struggle to accurately capture the dynamic nature of flood conditions, road closures, and other real-time changes inherent in urban disaster logistics. This paper introduces the ReinforceRouting model, a novel approach to optimizing evacuation routes using reinforcement learning (RL). The model incorporates a unique RL environment that considers multiple criteria, such as traffic conditions, hazardous situations, and the availability of safe routes. The RL agent in this model learns optimal actions through interaction with the environment, receiving feedback in the form of rewards or penalties. The ReinforceRouting model excels in executing prompt and accurate route planning on large road networks, outperforming traditional RL algorithms and shortest-path-based algorithms. A higher safety score and episode reward of the model are demonstrated when compared to these classical methods. This innovative approach to disaster evacuation planning offers a promising avenue for enhancing the efficiency, safety, and reliability of emergency responses in dynamic urban environments.
更多查看译文
关键词
Disaster evacuation,reinforcement learning,Geographic Information Science and Systems (GIS),artificial intelligence,routing algorithm
AI 理解论文
溯源树
样例
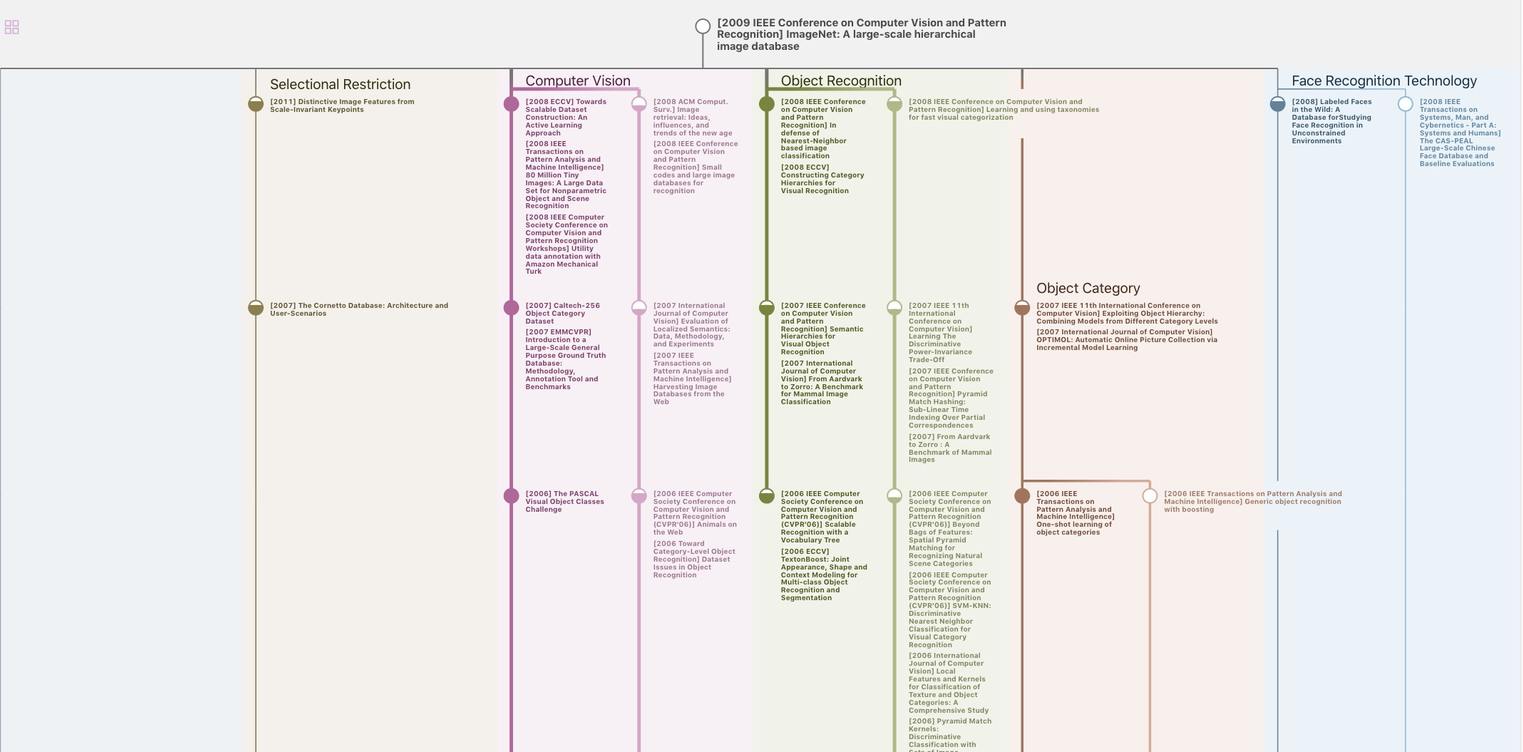
生成溯源树,研究论文发展脉络
Chat Paper
正在生成论文摘要