Integrated clinical and genomic models using machine-learning methods to predict the efficacy of paclitaxel-based chemotherapy in patients with advanced gastric cancer
BMC Cancer(2024)
摘要
Paclitaxel is commonly used as a second-line therapy for advanced gastric cancer (AGC). The decision to proceed with second-line chemotherapy and select an appropriate regimen is critical for vulnerable patients with AGC progressing after first-line chemotherapy. However, no predictive biomarkers exist to identify patients with AGC who would benefit from paclitaxel-based chemotherapy. This study included 288 patients with AGC receiving second-line paclitaxel-based chemotherapy between 2017 and 2022 as part of the K-MASTER project, a nationwide government-funded precision medicine initiative. The data included clinical (age [young-onset vs. others], sex, histology [intestinal vs. diffuse type], prior trastuzumab use, duration of first-line chemotherapy), and genomic factors (pathogenic or likely pathogenic variants). Data were randomly divided into training and validation sets (0.8:0.2). Four machine learning (ML) methods, namely random forest (RF), logistic regression (LR), artificial neural network (ANN), and ANN with genetic embedding (ANN with GE), were used to develop the prediction model and validated in the validation sets. The median patient age was 64 years (range 25–91), and 65.6
更多查看译文
关键词
Advanced gastric cancer,Paclitaxel,Machine learning,Prediction,Artificial neural network
AI 理解论文
溯源树
样例
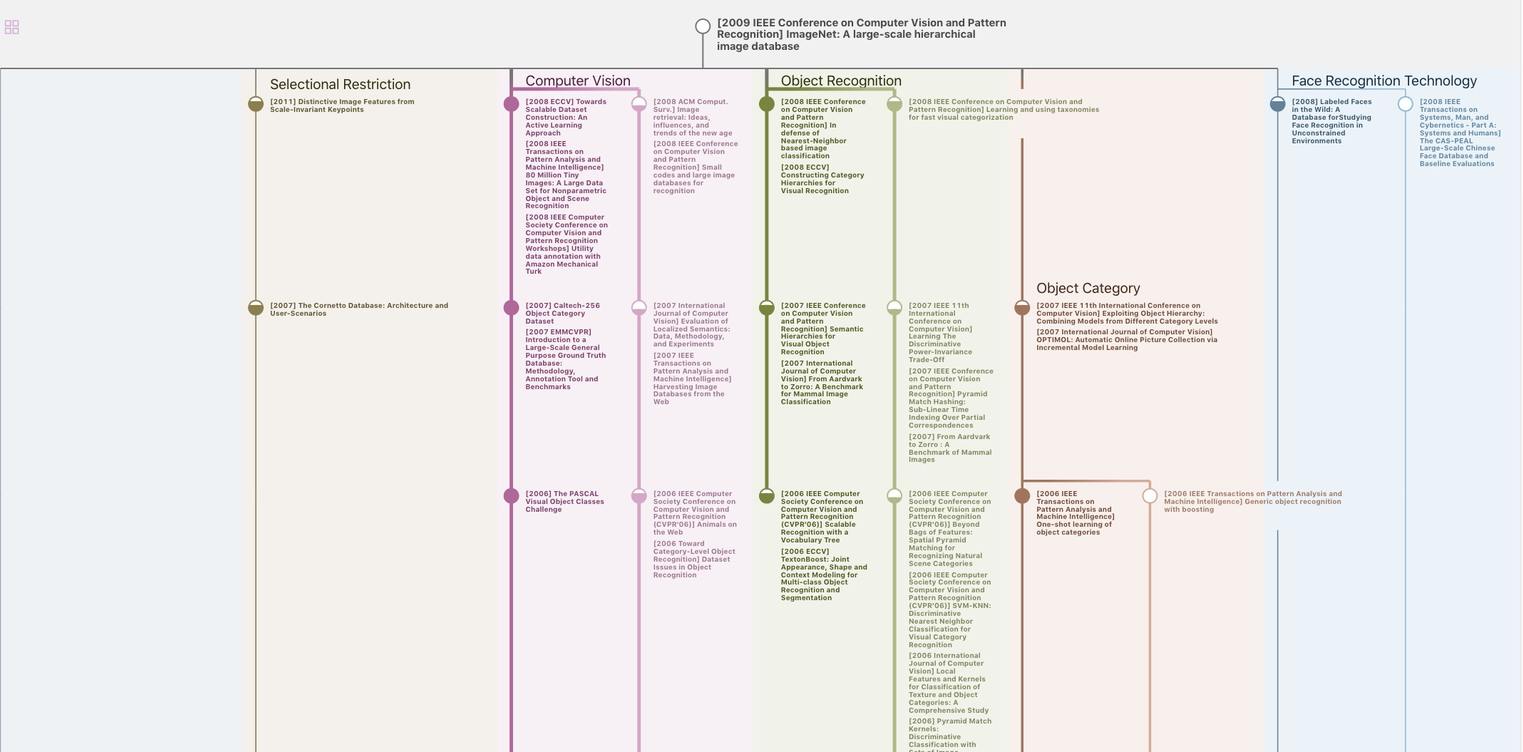
生成溯源树,研究论文发展脉络
Chat Paper
正在生成论文摘要