Online accelerated data-driven learning for optimal feedback control of discrete-time partially uncertain systems
INTERNATIONAL JOURNAL OF ADAPTIVE CONTROL AND SIGNAL PROCESSING(2024)
摘要
In this paper, we develop an online learning algorithm for solving the Bellman equation for affine in the control discrete-time nonlinear uncertain dynamical systems. To ensure accelerated learning of our algorithm in generating optimal control policies, we use an actor-critic structure predicated on higher-order tuner laws. More specifically, we construct a Nesterov-like architecture involving momentum-based learning laws leading to an accelerated convergence of the optimal control policy. The proposed online learning-based optimal control framework guarantees uniform ultimate boundedness of the closed-loop system under the assumption that the system is persistently excited. Finally, two illustrative numerical examples are provided to demonstrate the efficacy of the proposed approach.
更多查看译文
关键词
approximate dynamic programming,discrete-time systems,high-order tuners,momentum-based learning,online learning,optimal control,uniform ultimate boundedness
AI 理解论文
溯源树
样例
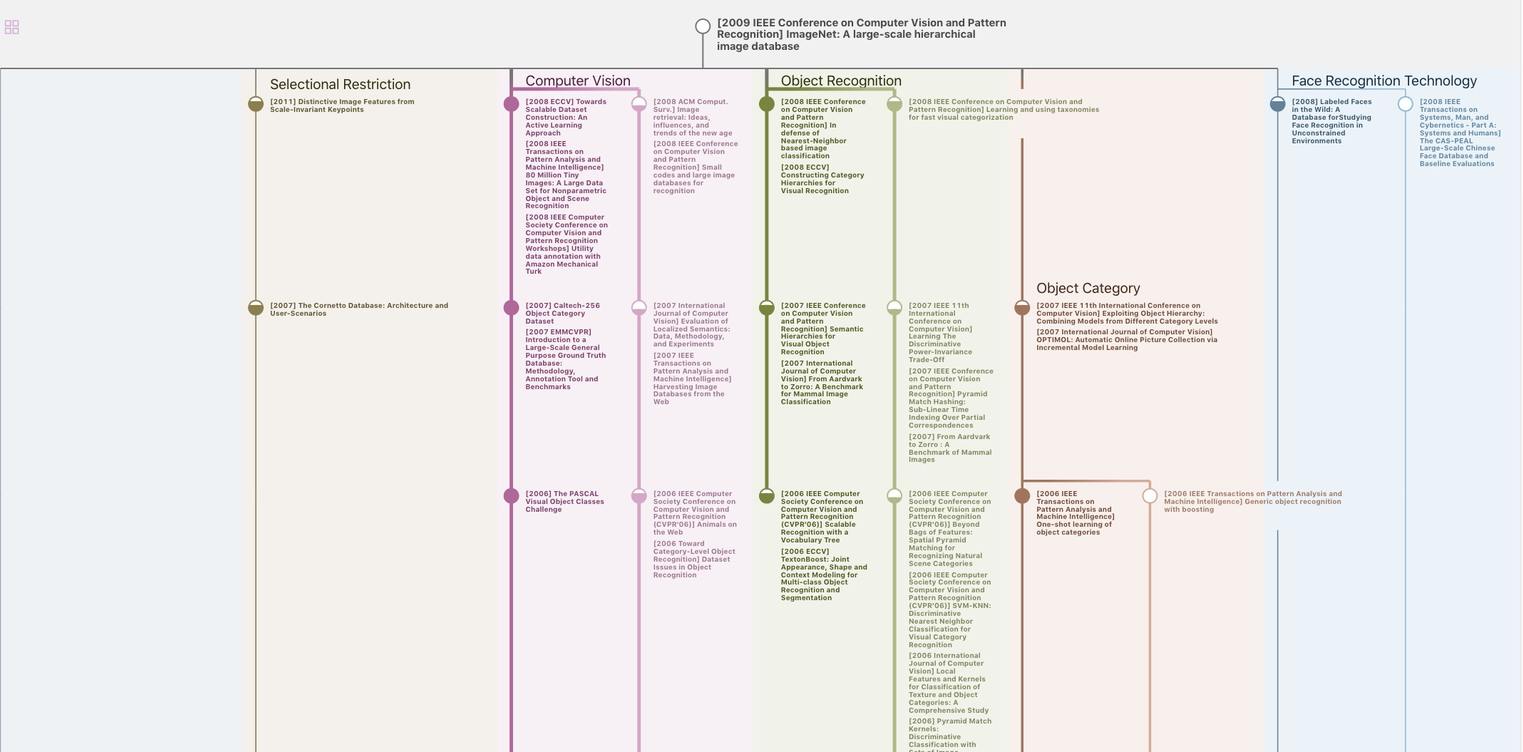
生成溯源树,研究论文发展脉络
Chat Paper
正在生成论文摘要