A Reinforcement Learning Benchmark for Autonomous Driving in General Urban Scenarios
IEEE TRANSACTIONS ON INTELLIGENT TRANSPORTATION SYSTEMS(2023)
摘要
Reinforcement learning (RL) has gained significant interest for its potential to improve decision and control in autonomous driving. However, current approaches have yet to demonstrate sufficient scenario generality and observation generality, hindering their wider utilization. To address these limitations, we propose a unified benchmark simulator for RL algorithms (called IDSim) to facilitate decision and control for high-level autonomous driving, with emphasis on diverse scenarios and a unified observation interface. IDSim is composed of a scenario library and a simulation engine, and is designed with execution efficiency and determinism in mind. The scenario library covers common urban scenarios, with automated random generation of road structure and traffic flow, and the simulation engine operates on the generated scenarios with dynamic interaction support. We conduct four groups of benchmark experiments with five common RL algorithms and focus on challenging signalized intersection scenarios with varying conditions. The results showcase the reliability of the simulator and reveal its potential to improve the generality of RL algorithms. Our analysis suggests that multi-task learning and observation design are potential areas for further algorithm improvement.
更多查看译文
关键词
Roads,Autonomous vehicles,Heuristic algorithms,Benchmark testing,Libraries,Vehicle dynamics,Reinforcement learning,Autonomous driving,benchmark simulator,reinforcement learning
AI 理解论文
溯源树
样例
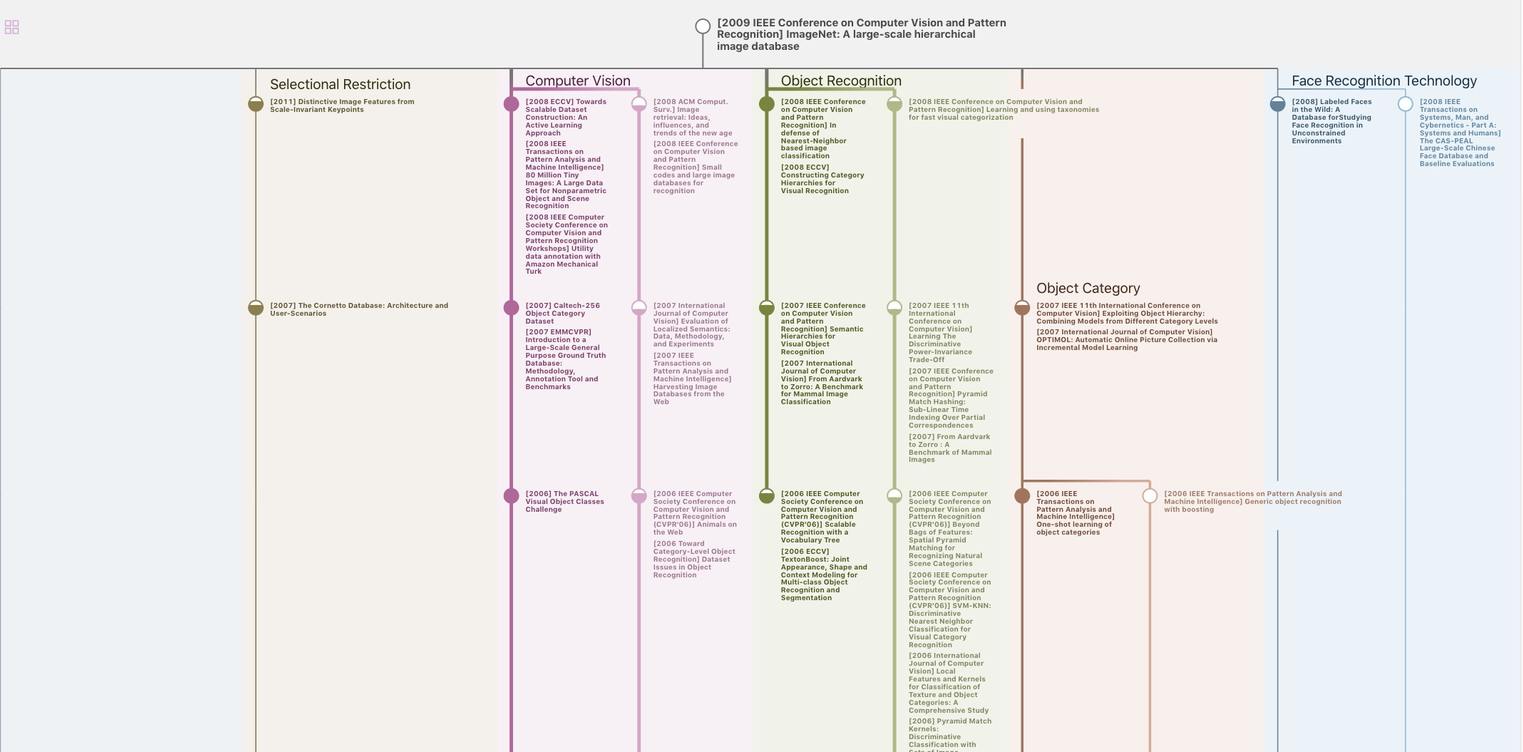
生成溯源树,研究论文发展脉络
Chat Paper
正在生成论文摘要